Receive a weekly summary and discussion of the top papers of the week by leading researchers in the field.
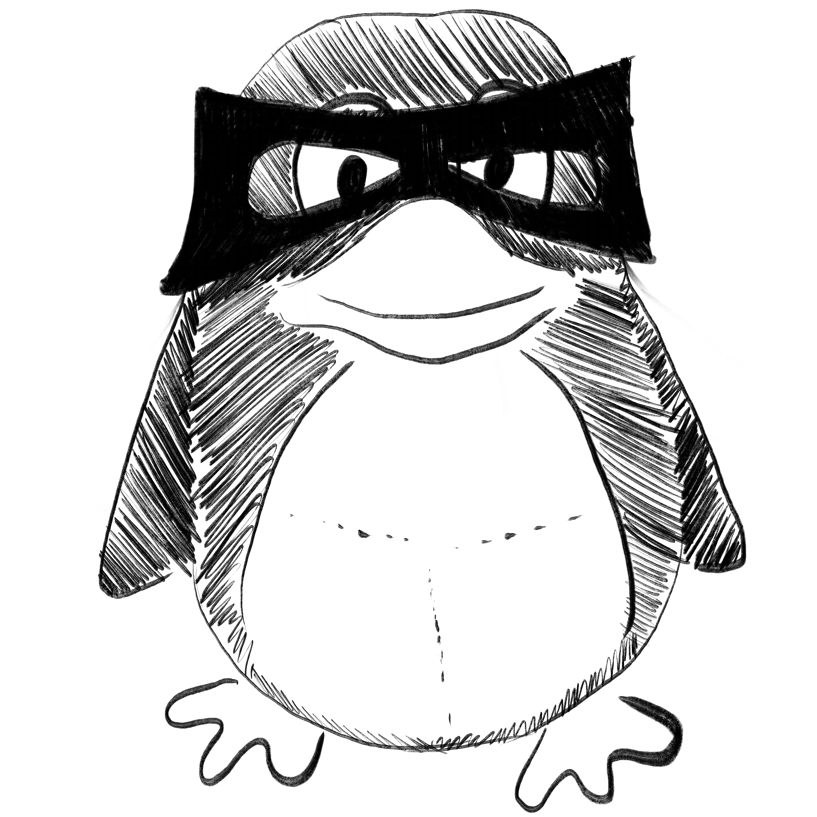
A bibliometric review of global visibility, impact and adoption of electronic invoicing: The past and the future.
In Heliyon
Olaleye Sunday Adewale, Sanusi Ismaila Temitayo, Dada Oluwaseun Alexander, Agbo Friday Joseph
2023-Mar
Automation, Bibliometric, Carbon footprint, Electronic invoicing, Electronic signature, Taxation
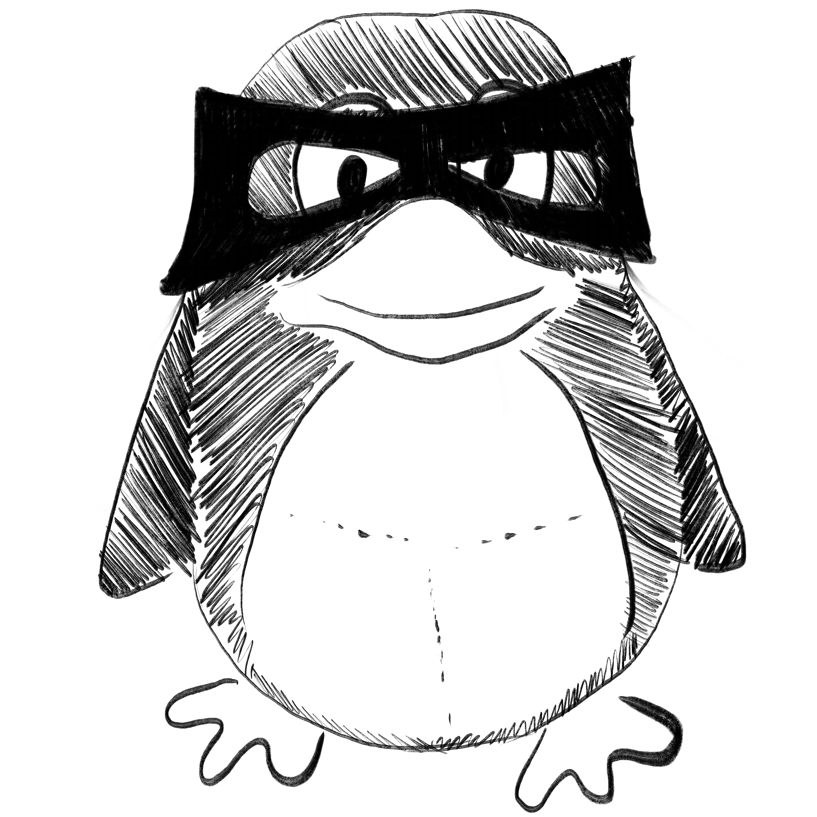
Smaller is better? Unduly nice accuracy assessments in roof detection using remote sensing data with machine learning and k-fold cross-validation.
In Heliyon
Abriha Dávid, Srivastava Prashant K, Szabó Szilárd
2023-Mar
Accuracy assessment, Object-based pixel purity, Post-classification, Roof classification, Salt-and-pepper effect, Urban environment
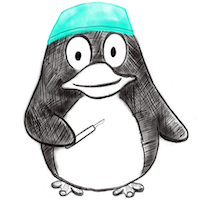
Development and validation of machine learning models for postoperative venous thromboembolism prediction in colorectal cancer inpatients: a retrospective study.
In Journal of gastrointestinal oncology
BACKGROUND :
METHODS :
RESULTS :
CONCLUSIONS :
Qin Li, Liang Zhikun, Xie Jingwen, Ye Guozeng, Guan Pengcheng, Huang Yaoyao, Li Xiaoyan
2023-Feb-28
Surgical colorectal cancer patient, machine learning model, venous thromboembolism (VTE)
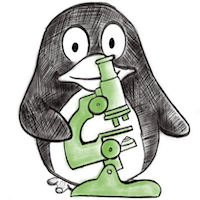
Paving New Roads Using Allium sativum as a Repurposed Drug and Analyzing its Antiviral Action Using Artificial Intelligence Technology.
In Iranian journal of pharmaceutical research : IJPR
CONTEXT :
OBJECTIVES :
METHOD :
RESULTS :
CONCLUSIONS :
Atoum Manar Fayiz, Padma Kanchi Ravi, Don Kanchi Ravi
2022-Dec
Allium sativum, Flavonoid, Immunomodulatory, SARS-CoV-2
Investigating nurses' acceptance of patients' bring your own device implementation in a clinical setting: A pilot study.
In Asia-Pacific journal of oncology nursing
OBJECTIVE :
METHODS :
RESULTS :
CONCLUSIONS :
Chien Shuo-Chen, Chen Chun-You, Chien Chia-Hui, Iqbal Usman, Yang Hsuan-Chia, Hsueh Huei-Chia, Weng Shuen-Fu, Jian Wen-Shan
2023-Mar
Bring your own device, Internet of things, Nurse acceptance, Smart hospital, Technology acceptance model
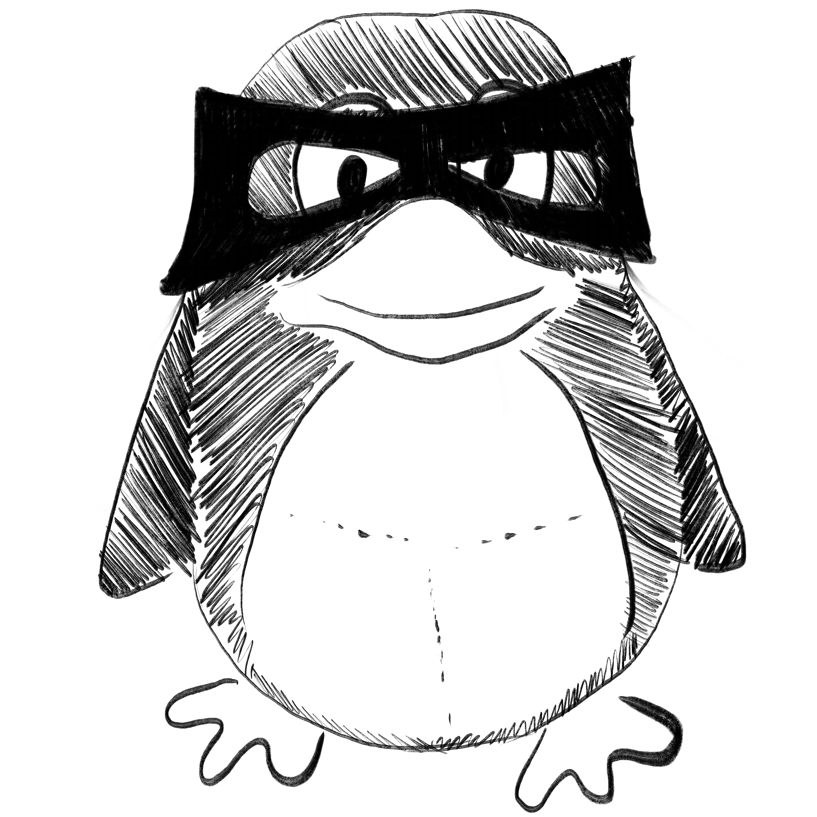
Automated pancreatic islet viability assessment for transplantation using bright-field deep morphological signature.
In Computational and structural biotechnology journal
Habibalahi Abbas, Campbell Jared M, Walters Stacey N, Mahbub Saabah B, Anwer Ayad G, Grey Shane T, Goldys Ewa M
2023
AI, artificial intelligence, DMOG, dimethyloxalylglycine, DMS, deep morphological signatures, Deep morphological signature, ECG, electrocardiogram, EEG, electroencephalogram, EMCCD, electron multiplying charge coupling device, FD, Fisher Distance, GSIS, glucose stimulated insulin secretion, IoU, intersection over union, MEG, magnetoencephalography, MRI, magnetic resonance imaging, PCA, principal component analysis, Pancreatic islet, ROS, reactive oxygen species, SI, swarm intelligence, SVM, support vector machine, Transplantation
Weekly Summary
Receive a weekly summary and discussion of the top papers of the week by leading researchers in the field.