Receive a weekly summary and discussion of the top papers of the week by leading researchers in the field.
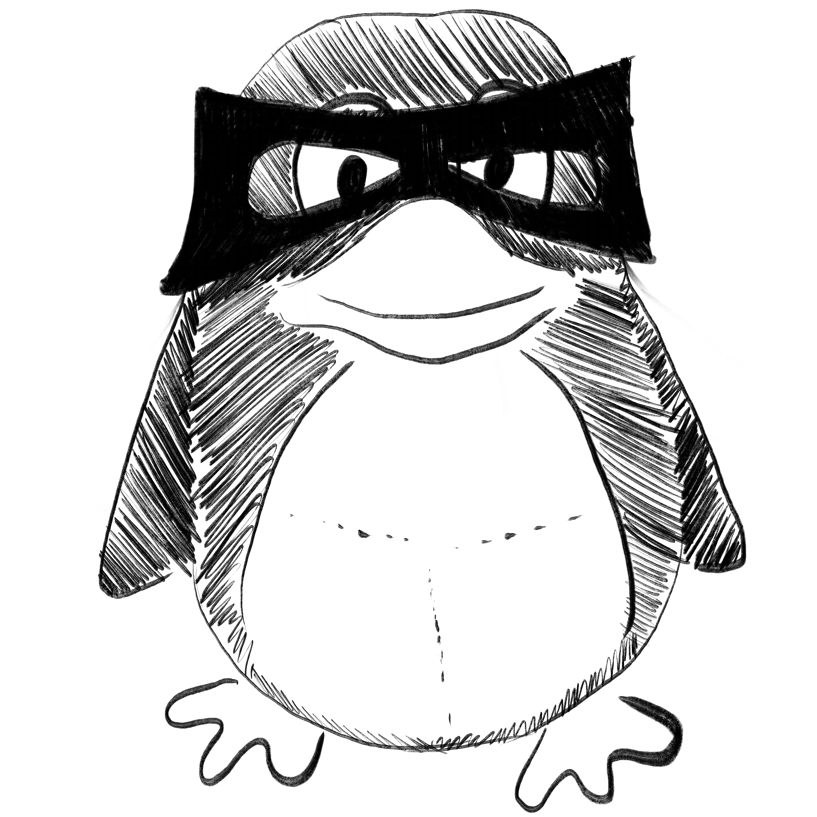
Tile-based microscopic image processing for malaria screening using a deep learning approach.
In BMC medical imaging
BACKGROUND :
METHODS :
RESULTS :
CONCLUSIONS :
Shewajo Fetulhak Abdurahman, Fante Kinde Anlay
2023-Mar-22
Deep learning, Malaria, Object detection, Plasmodium falciparum, Thick smear microscopic image, Tile-based image processing, YOLOV4
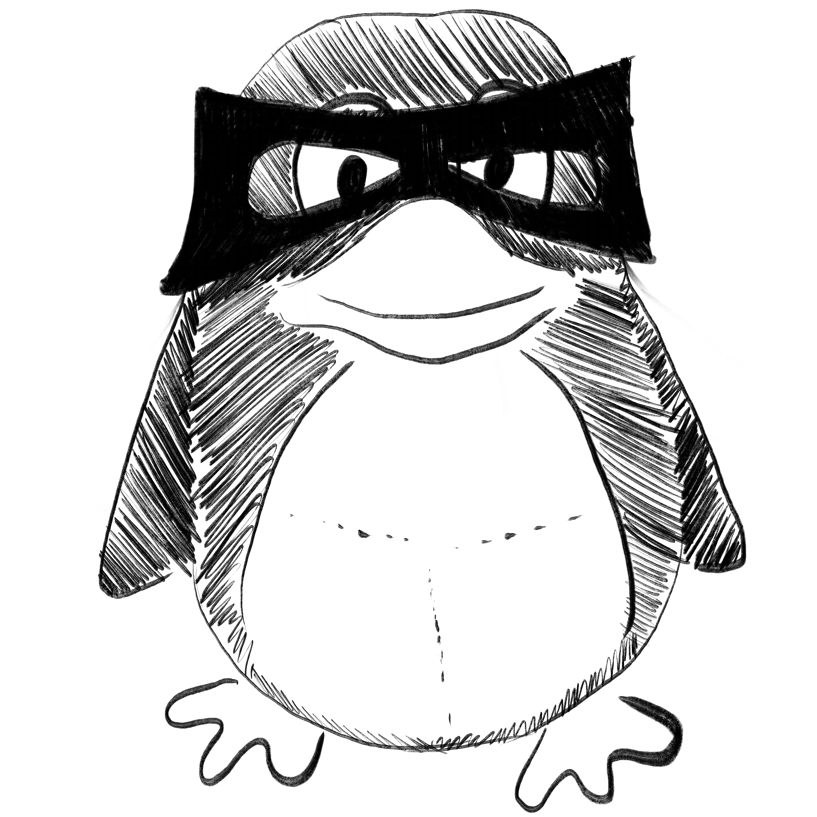
Prediction of vancomycin initial dosage using artificial intelligence models applying ensemble strategy.
In BMC bioinformatics
BACKGROUND :
RESULTS :
CONCLUSIONS :
Ho Wen-Hsien, Huang Tian-Hsiang, Chen Yenming J, Zeng Lang-Yin, Liao Fen-Fen, Liou Yeong-Cheng
2023-Mar-22
Ensemble strategy, Monitoring of blood concentration of drugs, Therapeutic drug monitoring (TDM), Vancomycin
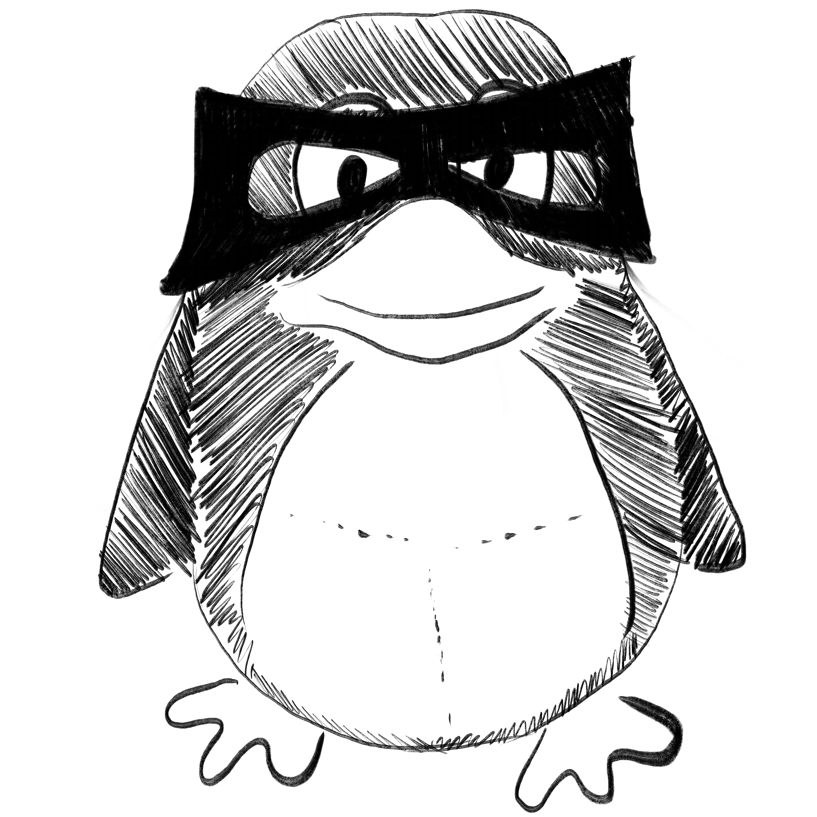
Dense reinforcement learning for safety validation of autonomous vehicles.
In Nature ; h5-index 368.0
Feng Shuo, Sun Haowei, Yan Xintao, Zhu Haojie, Zou Zhengxia, Shen Shengyin, Liu Henry X
2023-Mar
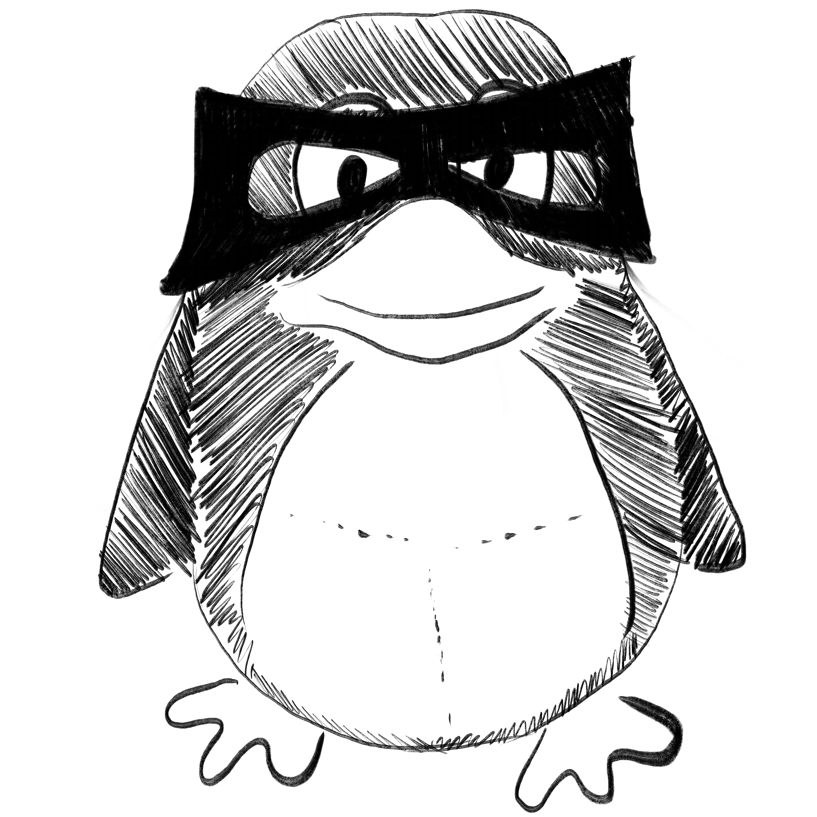
Forecasting PM2.5 concentrations using statistical modeling for Bengaluru and Delhi regions.
In Environmental monitoring and assessment
Agarwal Akash, Sahu Manoranjan
2023-Mar-23
ARIMA, Air quality forecasting, Data analytics, Machine learning
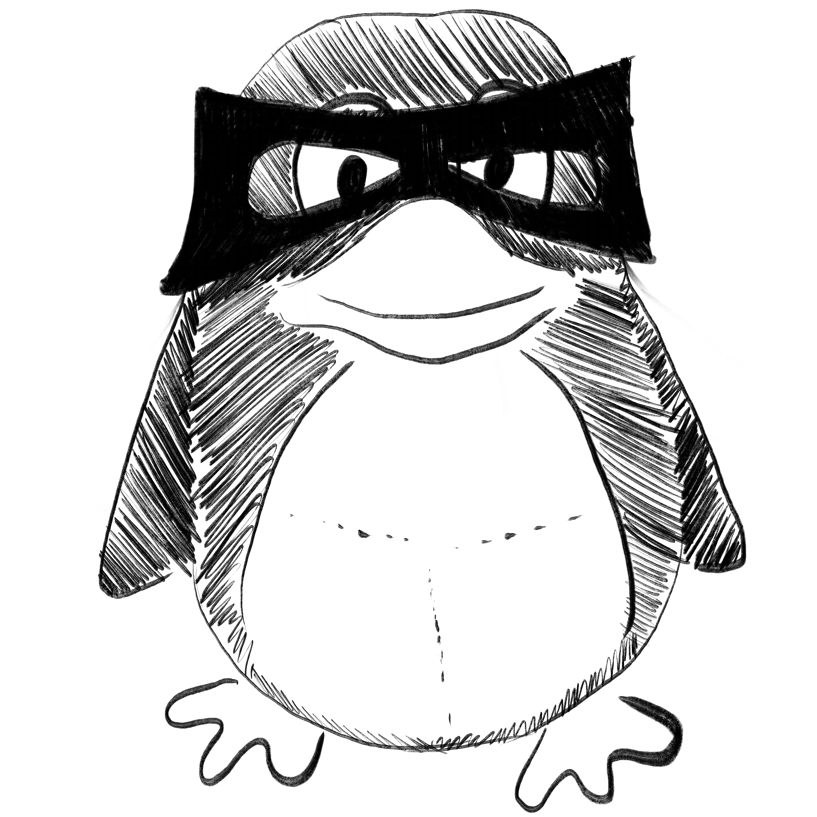
Ranking parameters driving siring success during sperm competition in the North African houbara bustard.
In Communications biology
Sorci Gabriele, Hussein Hiba Abi, Levêque Gwènaëlle, Saint Jalme Michel, Lacroix Frédéric, Hingrat Yves, Lesobre Loïc
2023-Mar-22
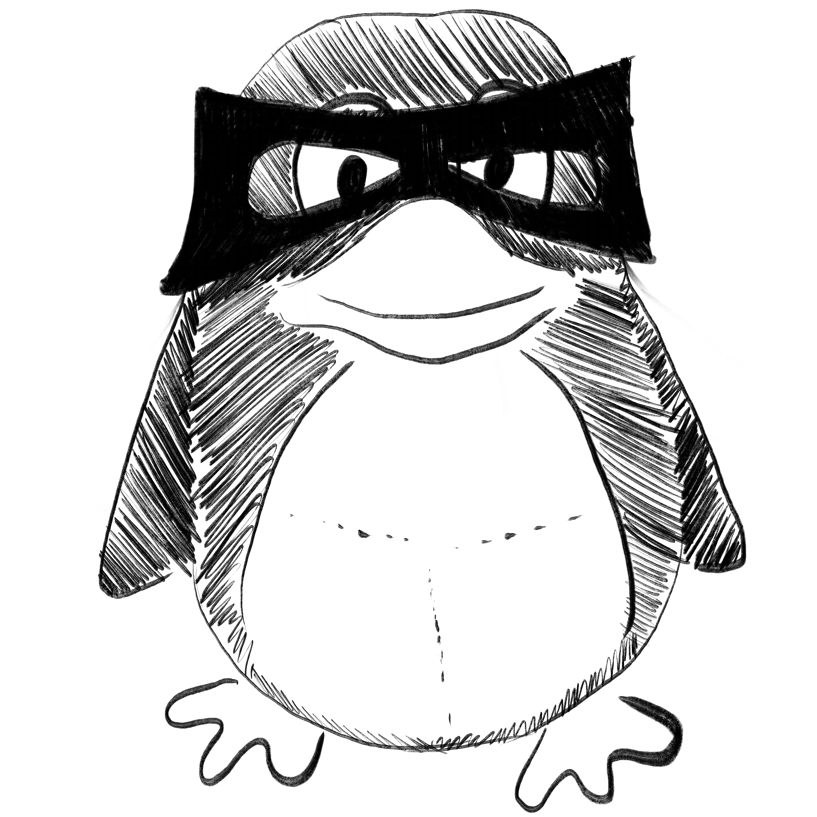
Establishing a prediction model of severe acute mountain sickness using machine learning of support vector machine recursive feature elimination.
In Scientific reports ; h5-index 158.0
Yang Min, Wu Yang, Yang Xing-Biao, Liu Tao, Zhang Ya, Zhuo Yue, Luo Yong, Zhang Nan
2023-Mar-21
Weekly Summary
Receive a weekly summary and discussion of the top papers of the week by leading researchers in the field.