Receive a weekly summary and discussion of the top papers of the week by leading researchers in the field.
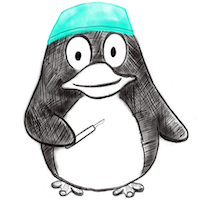
Pathologist Validation of a Machine Learning-Derived Feature for Colon Cancer Risk Stratification.
In JAMA network open
IMPORTANCE :
OBJECTIVE :
DESIGN, SETTING, AND PARTICIPANTS :
MAIN OUTCOMES AND MEASURES :
RESULTS :
CONCLUSIONS AND RELEVANCE :
L’Imperio Vincenzo, Wulczyn Ellery, Plass Markus, Müller Heimo, Tamini Nicolò, Gianotti Luca, Zucchini Nicola, Reihs Robert, Corrado Greg S, Webster Dale R, Peng Lily H, Chen Po-Hsuan Cameron, Lavitrano Marialuisa, Liu Yun, Steiner David F, Zatloukal Kurt, Pagni Fabio
2023-Mar-01
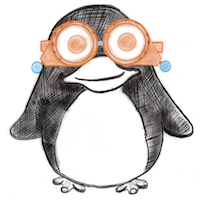
Electrical Stimulation Induced Current Distribution in Peripheral Nerves Varies Significantly with the Extent of Nerve Damage: A Computational Study Utilizing Convolutional Neural Network and Realistic Nerve Models.
In International journal of neural systems
Du Jinze, Morales Andres, Kosta Pragya, Bouteiller Jean-Marie C, Martinez-Navarrete Gema, Warren David J, Fernandez Eduardo, Lazzi Gianluca
2023-Mar-15
Computational model, electrical stimulation, peripheral nerve, tissue safety
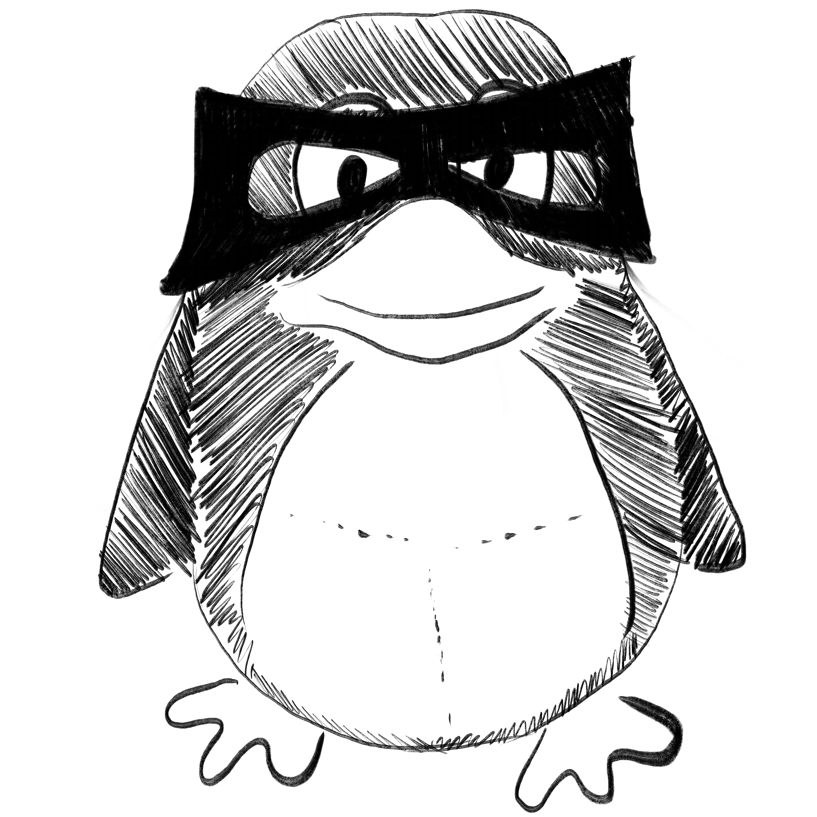
Blind Users Accessing Their Training Images in Teachable Object Recognizers.
In ASSETS. Annual ACM Conference on Assistive Technologies
Hong Jonggi, Gandhi Jaina, Mensah Ernest Essuah, Zeraati Farnaz Zamiri, Jarjue Ebrima Haddy, Lee Kyungjun, Kacorri Hernisa
2022-Oct
blind, machine teaching, object recognition, participatory machine learning, visual impairment
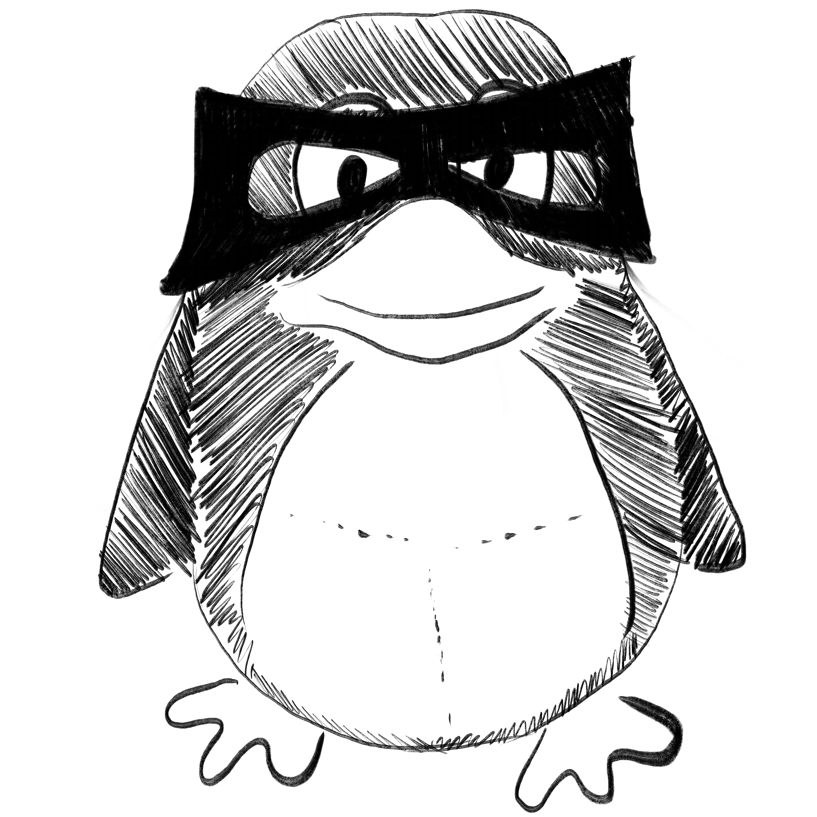
Niche-Based Microbial Community Assemblage in Urban Transit Systems and the Influence of City Characteristics.
In Microbiology spectrum
Xiong Guangzhou, Ji Lei, Cheng Mingyue, Ning Kang
2023-Mar-14
artificial intelligence, city characteristics, microbiota assemblage, urban transit system
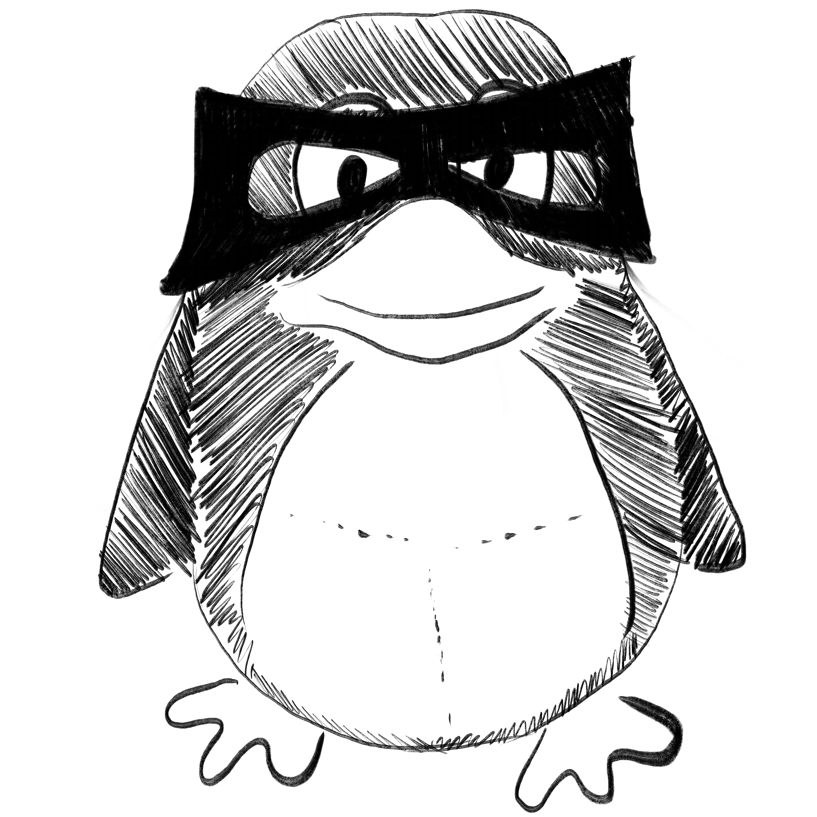
The Rise of ChatGPT: Exploring its Potential in Medical Education.
In Anatomical sciences education
Lee Hyunsu
2023-Mar-14
Artificial Intelligence, ChatGPT, Medical education, Natural language processing, Virtual teaching assistant
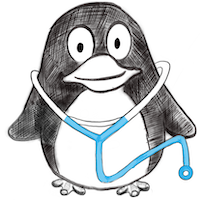
Predicting metastasis in gastric cancer patients: machine learning-based approaches.
In Scientific reports ; h5-index 158.0
Talebi Atefeh, Celis-Morales Carlos A, Borumandnia Nasrin, Abbasi Somayeh, Pourhoseingholi Mohamad Amin, Akbari Abolfazl, Yousefi Javad
2023-Mar-13
Weekly Summary
Receive a weekly summary and discussion of the top papers of the week by leading researchers in the field.