Receive a weekly summary and discussion of the top papers of the week by leading researchers in the field.
Artificial intelligence CAD tools in trauma imaging: a scoping review from the American Society of Emergency Radiology (ASER) AI/ML Expert Panel.
In Emergency radiology
BACKGROUND :
PURPOSE :
METHODS :
RESULTS :
CONCLUSIONS :
Dreizin David, Staziaki Pedro V, Khatri Garvit D, Beckmann Nicholas M, Feng Zhaoyong, Liang Yuanyuan, Delproposto Zachary S, Klug Maximiliano, Spann J Stephen, Sarkar Nathan, Fu Yunting
2023-Mar-14
Artificial intelligence, Computer-aided detection, Emergency, Emergency radiology, Imaging, Machine learning, Radiology, Scoping review, Trauma
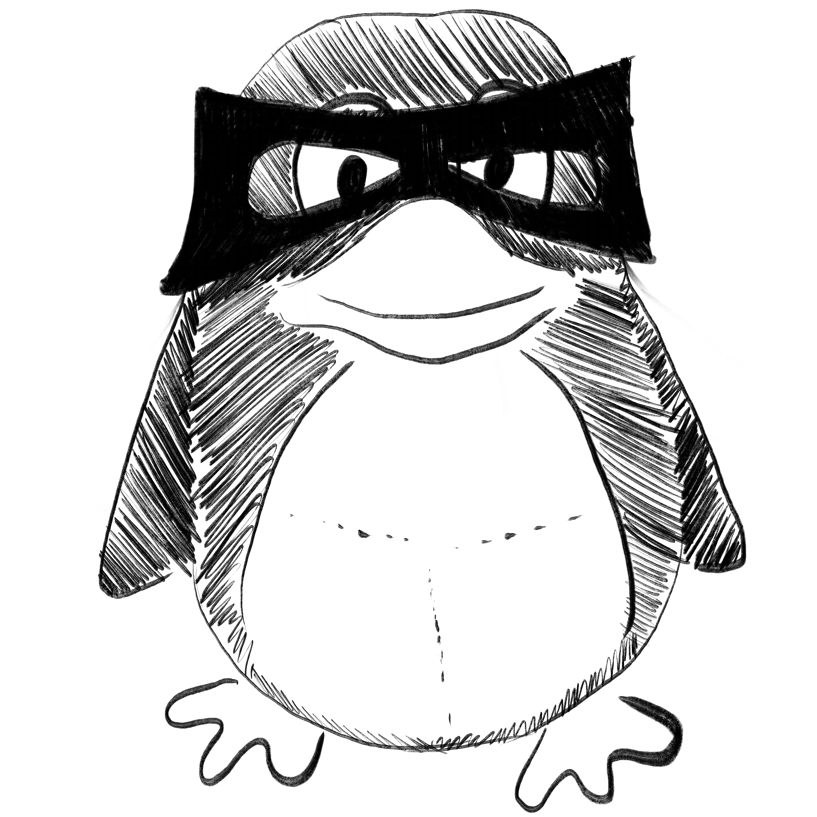
Ensemble learning-based gene signature and risk model for predicting prognosis of triple-negative breast cancer.
In Functional & integrative genomics
Li Tiancheng, Chen Siqi, Zhang Yuqi, Zhao Qianqian, Ma Kai, Jiang Xiwei, Xiang Rongwu, Zhai Fei, Ling Guixia
2023-Mar-14
Genomics, Immune, Machine learning, Myeloid cell, Triple-negative breast cancer
Artificial intelligence in BreastScreen Norway: a retrospective analysis of a cancer-enriched sample including 1254 breast cancer cases.
In European radiology ; h5-index 62.0
OBJECTIVES :
METHODS :
RESULTS :
CONCLUSIONS :
KEY POINTS :
Koch Henrik Wethe, Larsen Marthe, Bartsch Hauke, Kurz Kathinka Dæhli, Hofvind Solveig
2023-Mar-14
Artificial intelligence, Breast neoplasm, Mammographic density, Mammography, Mass screening
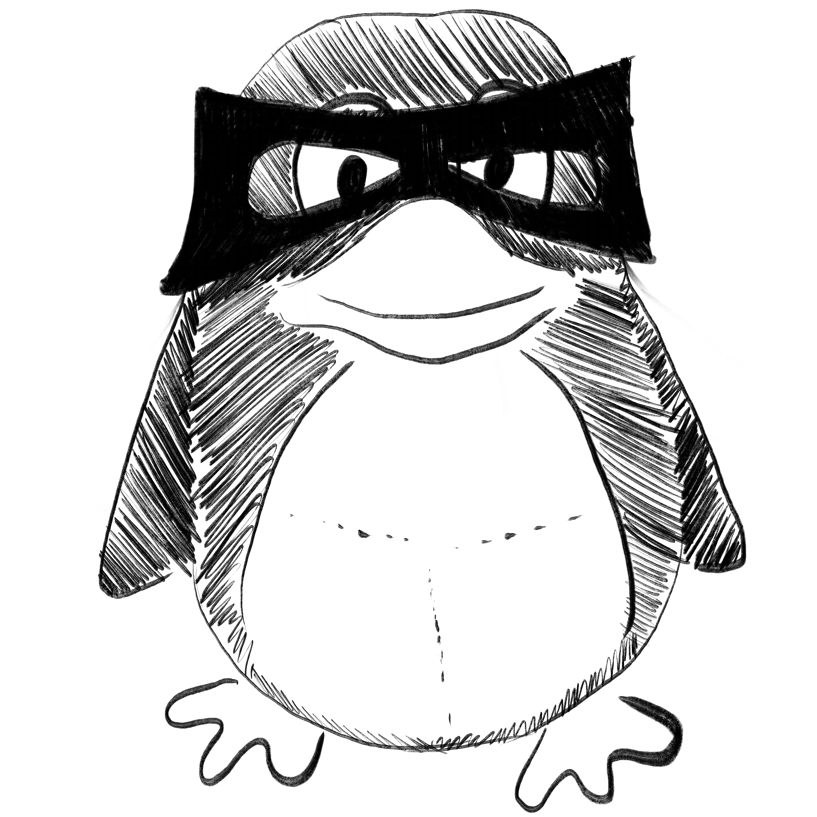
Predicting Pain in People With Sickle Cell Disease in the Day Hospital Using the Commercial Wearable Apple Watch: Feasibility Study.
In JMIR formative research
BACKGROUND :
OBJECTIVE :
METHODS :
RESULTS :
CONCLUSIONS :
Stojancic Rebecca Sofia, Subramaniam Arvind, Vuong Caroline, Utkarsh Kumar, Golbasi Nuran, Fernandez Olivia, Shah Nirmish
2023-Mar-14
Apple Watch, consumer wearable, machine learning, mobile health, pain, predict, prediction, sickle cell disease, smartwatch, vaso-occlusive crises, wearable
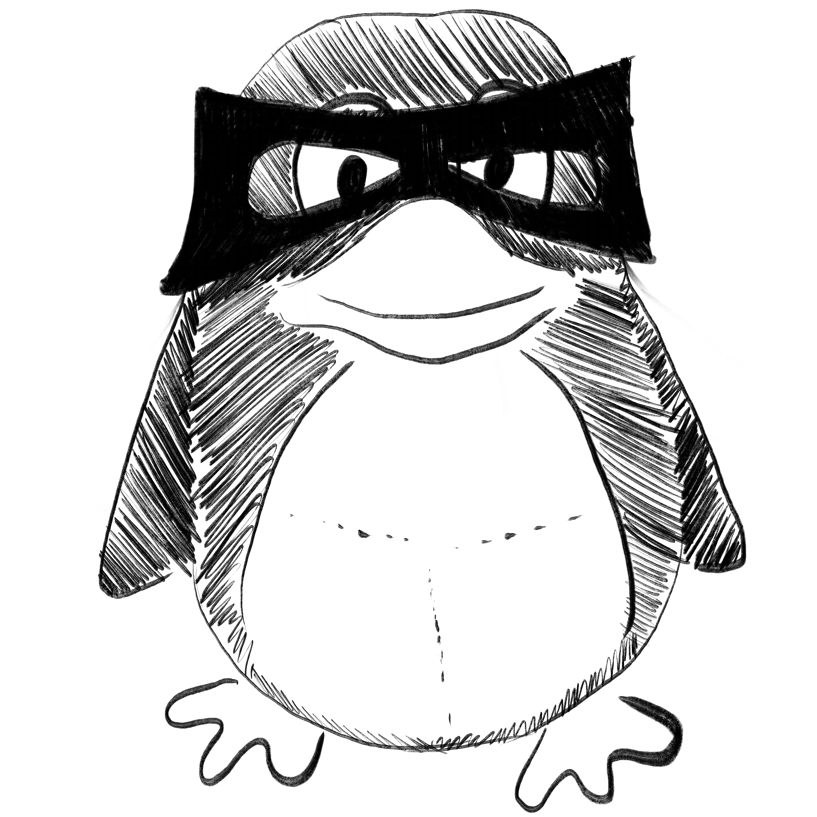
Enabling fully automated insulin delivery through meal detection and size estimation using Artificial Intelligence.
In NPJ digital medicine
Mosquera-Lopez Clara, Wilson Leah M, El Youssef Joseph, Hilts Wade, Leitschuh Joseph, Branigan Deborah, Gabo Virginia, Eom Jae H, Castle Jessica R, Jacobs Peter G
2023-Mar-13
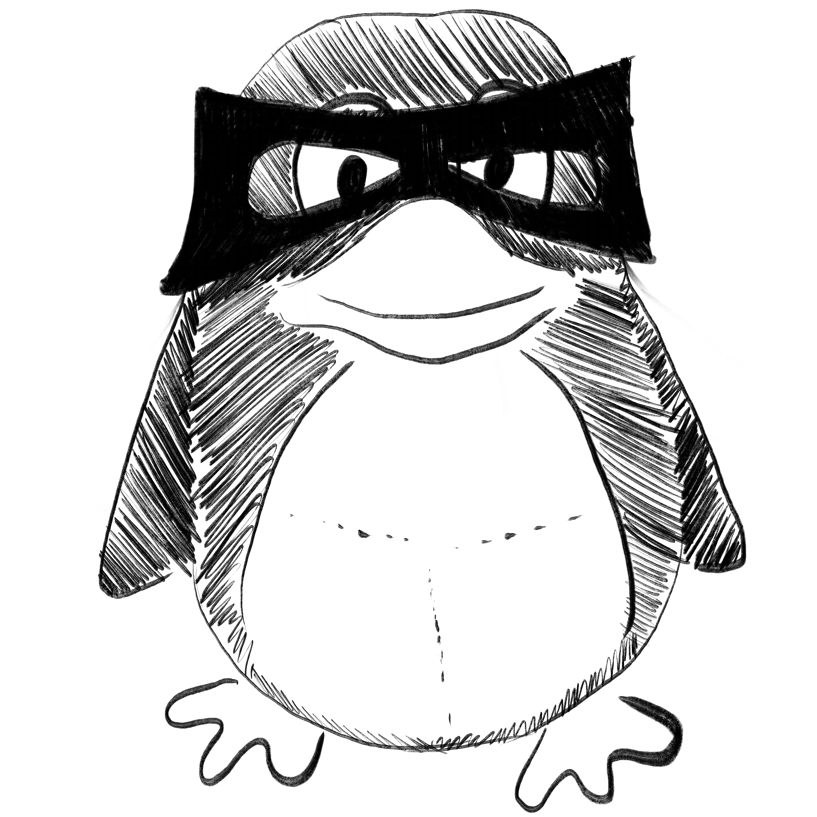
Comprehensive analysis of clinical data for COVID-19 outcome estimation with machine learning models.
In Biomedical signal processing and control
Morís Daniel I, de Moura Joaquim, Marcos Pedro J, Rey Enrique Míguez, Novo Jorge, Ortega Marcos
2023-Jul
COVID-19, Classification, Clinical data, Feature selection, Machine learning
Weekly Summary
Receive a weekly summary and discussion of the top papers of the week by leading researchers in the field.