Receive a weekly summary and discussion of the top papers of the week by leading researchers in the field.
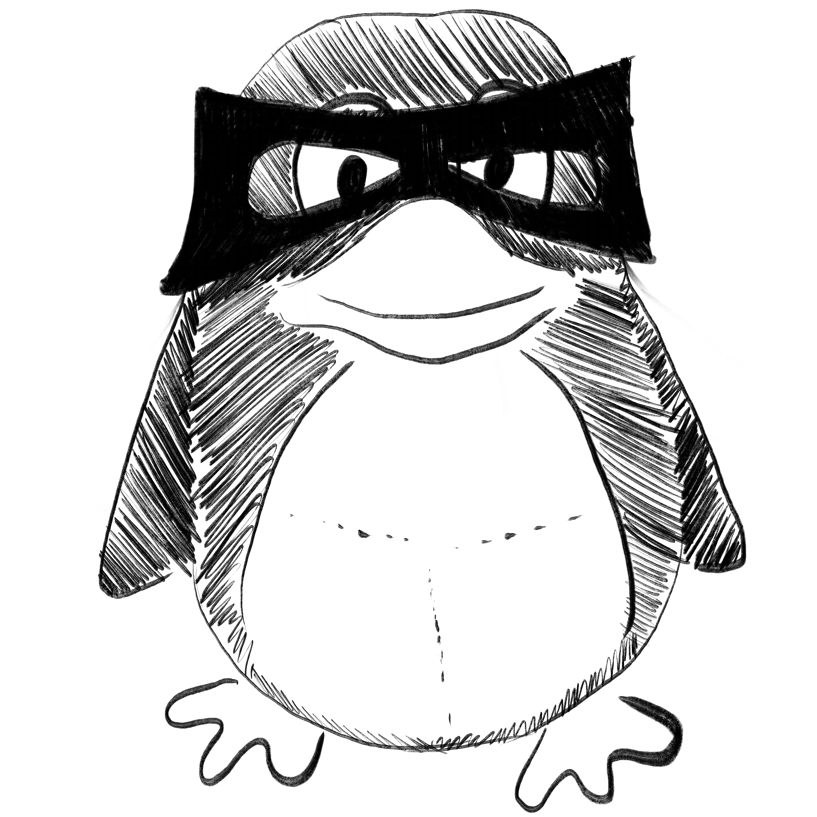
Connectome-based predictive modeling for functional recovery of acute ischemic stroke.
In NeuroImage. Clinical
Peng Syu-Jyun, Chen Yu-Wei, Hung Andrew, Wang Kuo-Wei, Tsai Jang-Zern
2023-Mar-08
BI, Connectome, Functional recovery, Prediction, Resting-state functional MRI, Stroke, mRS
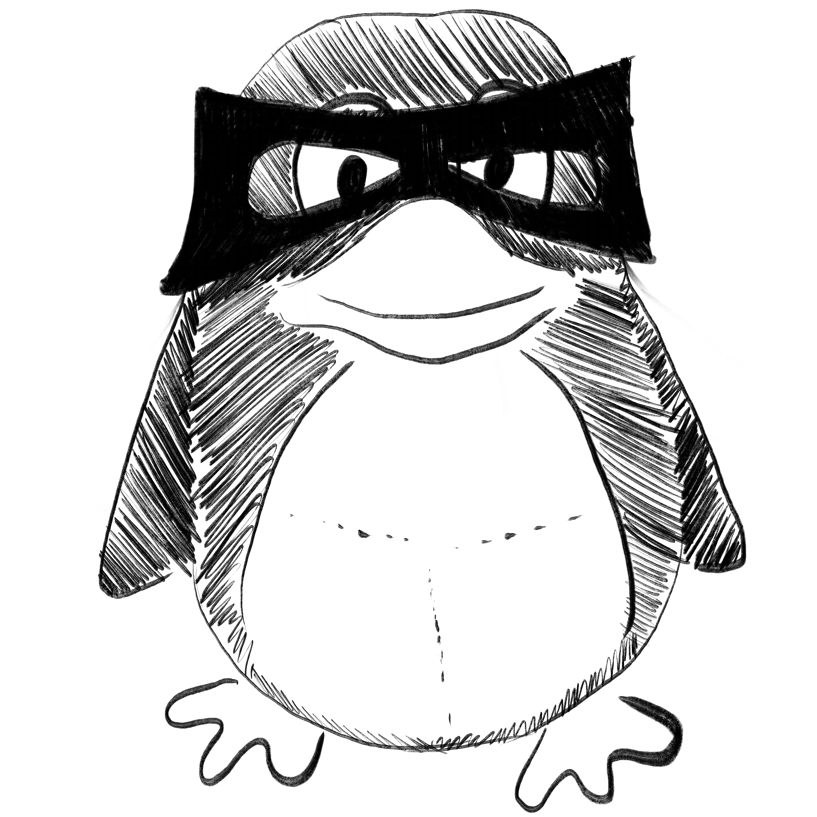
Novel flexible sensing technology for nondestructive detection on live fish health/quality during waterless and low-temperature transportation.
In Biosensors & bioelectronics
Feng Huanhuan, Fu Yifan, Huang Shihao, Glamuzina Branko, Zhang Xiaoshuan
2023-Mar-08
Biosensors, Flexible sensing, Internet of things, Machine learning (ML) fusion, Nondestructive detection
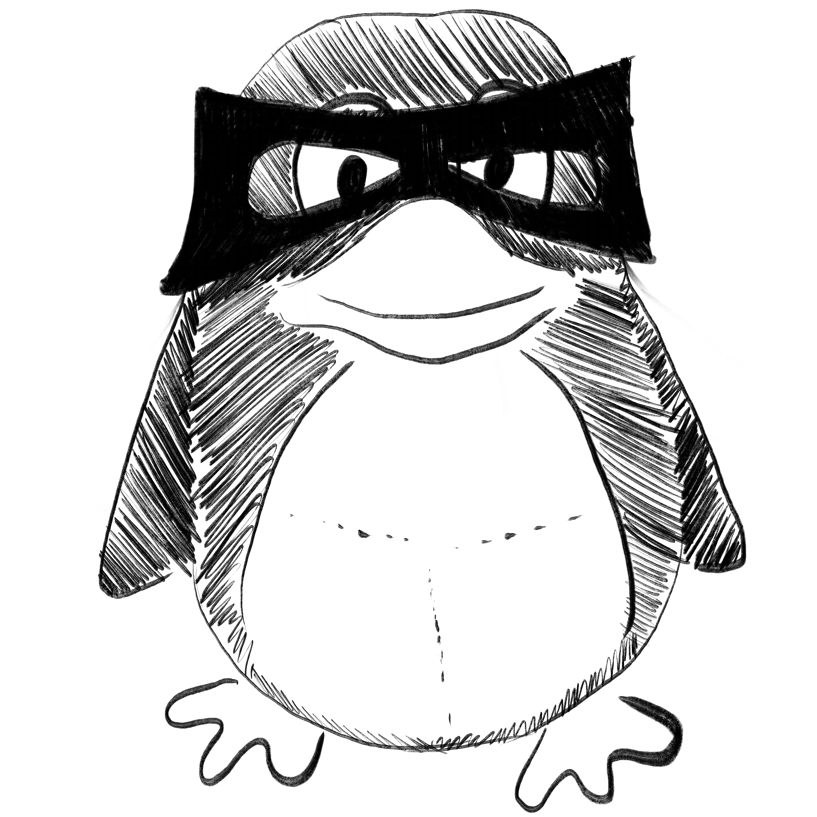
The HARM models: Predicting longitudinal physical aggression in patients with schizophrenia at an individual level.
In Journal of psychiatric research ; h5-index 59.0
Watts Devon, Mamak Mini, Moulden Heather, Upfold Casey, de Azevedo Cardoso Taiane, Kapczinski Flavio, Chaimowitz Gary
2023-Mar-01
Artificial intelligence, Computational neuroscience, Criminality, Machine learning, Precision psychiatry, Psychotic disorders, Schizophrenia
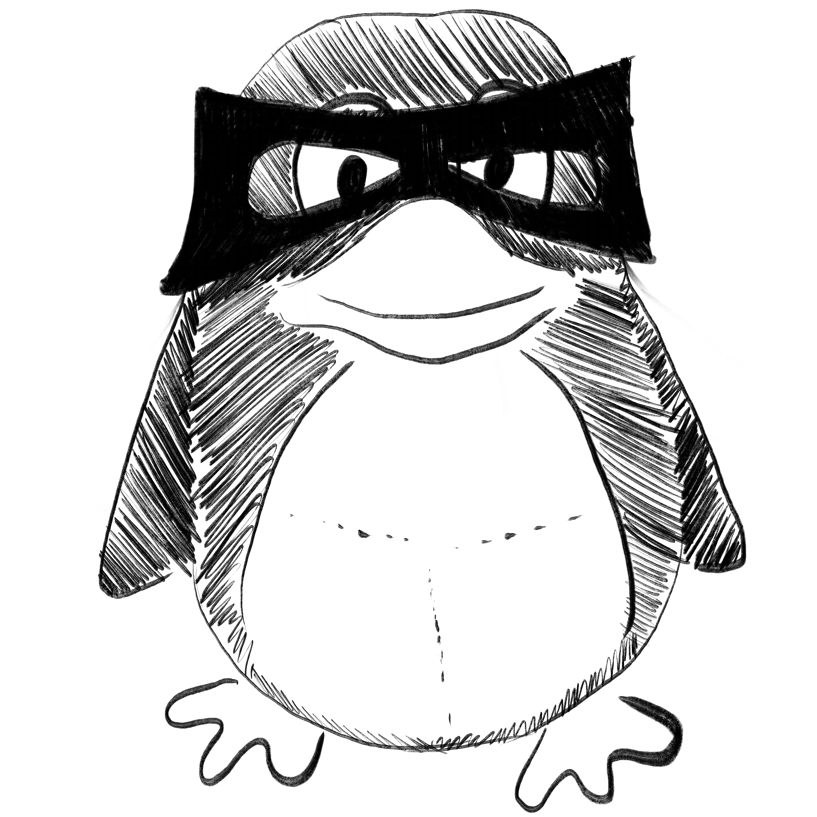
Exploring the Knowledge Attained by Machine Learning on Ion Transport across Polyamide Membranes Using Explainable Artificial Intelligence.
In Environmental science & technology ; h5-index 132.0
Jeong Nohyeong, Epsztein Razi, Wang Ruoyu, Park Shinyun, Lin Shihong, Tong Tiezheng
2023-Mar-14
explainable artificial intelligence, ion transport, machine learning, membrane separation mechanisms, nanofiltration, reverse osmosis
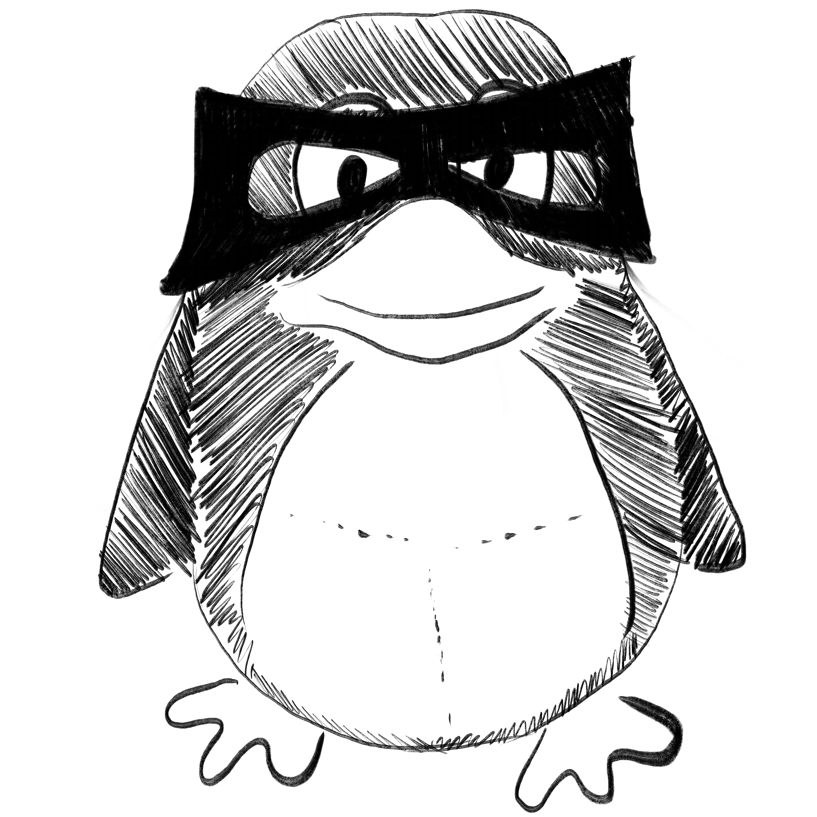
Taking advantage of quasi-periodic signals for S2S operational forecast from a perspective of deep learning.
In Scientific reports ; h5-index 158.0
Zhou Yang, Zhao Qifan
2023-Mar-13
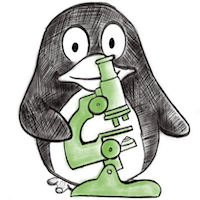
Task-specific Fine-tuning via Variational Information Bottleneck for Weakly-supervised Pathology Whole Slide Image Classification
ArXiv Preprint
Honglin Li, Chenglu Zhu, Yunlong Zhang, Yuxuan Sun, Zhongyi Shui, Wenwei Kuang, Sunyi Zheng, Lin Yang
2023-03-15
Weekly Summary
Receive a weekly summary and discussion of the top papers of the week by leading researchers in the field.