Receive a weekly summary and discussion of the top papers of the week by leading researchers in the field.
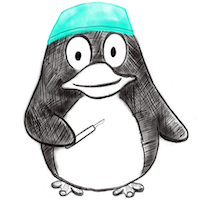
Preoperative Prognosis Assessment of Lumbar Spinal Surgery for Low Back Pain and Sciatica Patients based on Multimodalities and Multimodal Learning
ArXiv Preprint
Li-Chin Chen, Jung-Nien Lai, Hung-En Lin, Hsien-Te Chen, Kuo-Hsuan Hung, Yu Tsao
2023-03-16
Clinicomics-guided distant metastasis prediction in breast cancer via artificial intelligence.
In BMC cancer
BACKGROUND :
METHODS :
RESULTS :
CONCLUSION :
Zhang Chao, Qi Lisha, Cai Jun, Wu Haixiao, Xu Yao, Lin Yile, Li Zhijun, Chekhonin Vladimir P, Peltzer Karl, Cao Manqing, Yin Zhuming, Wang Xin, Ma Wenjuan
2023-Mar-14
Artificial Intelligence, Breast Cancer, Image, Metastasis, Prediction
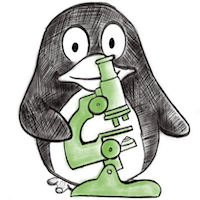
The NCI Imaging Data Commons as a platform for reproducible research in computational pathology
ArXiv Preprint
Daniela P. Schacherer, Markus D. Herrmann, David A. Clunie, Henning Höfener, William Clifford, William J. R. Longabaugh, Steve Pieper, Ron Kikinis, Andrey Fedorov, André Homeyer
2023-03-16
Transcranial focused ultrasound-mediated unbinding of phenytoin from plasma proteins for suppression of chronic temporal lobe epilepsy in a rodent model.
In Scientific reports ; h5-index 158.0
Kim Evgenii, Kim Hyun-Chul, Van Reet Jared, Böhlke Mark, Yoo Seung-Schik, Lee Wonhye
2023-Mar-13
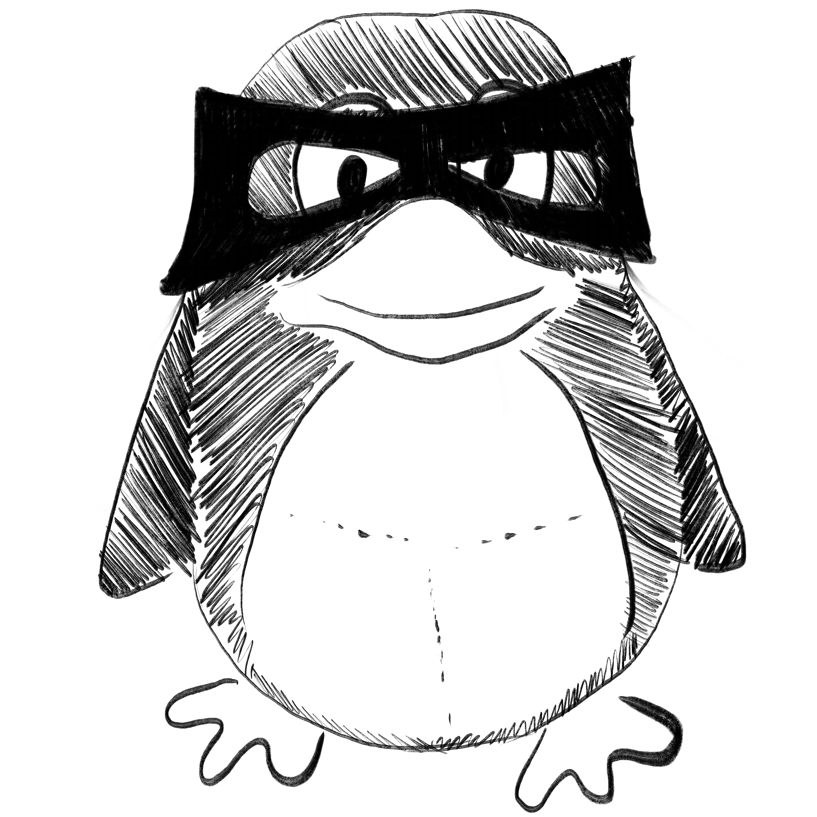
The predictive model for COVID-19 pandemic plastic pollution by using deep learning method.
In Scientific reports ; h5-index 158.0
Nanehkaran Yaser A, Licai Zhu, Azarafza Mohammad, Talaei Sona, Jinxia Xu, Chen Junde, Derakhshani Reza
2023-Mar-13
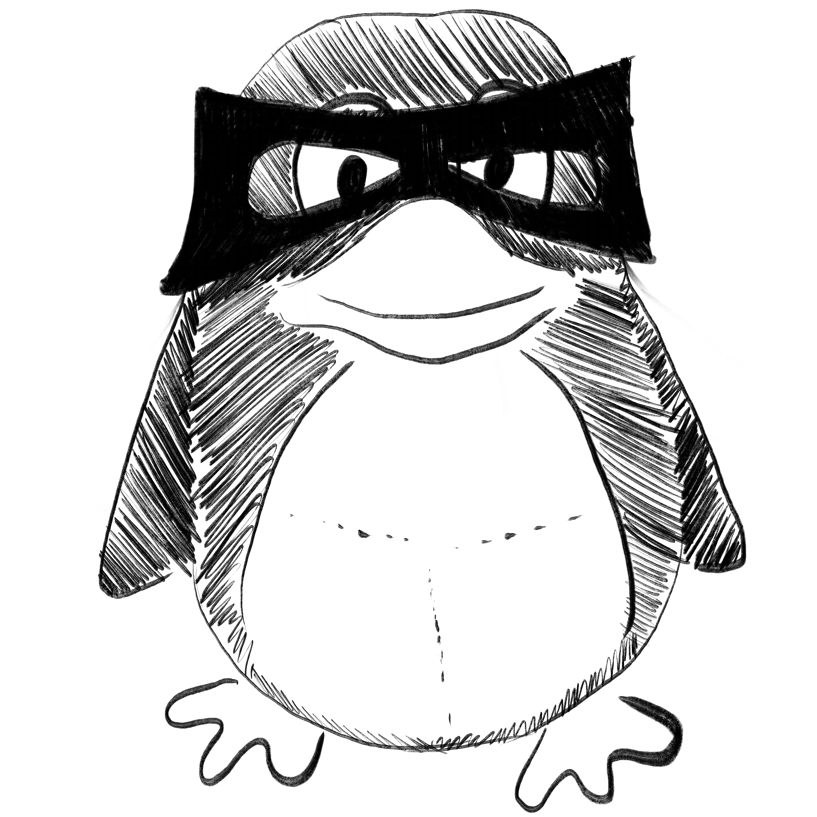
Differentially expressed genes in systemic sclerosis: Towards predictive medicine with new molecular tools for clinicians.
In Autoimmunity reviews ; h5-index 77.0
Keret Shiri, Rimar Doron, Lansiaux Pauline, Feldman Erik, Lescoat Alain, Milman Neta, Farge Dominique
2023-Mar-12
Autologous hematopoietic stem cell transplantation, Intrinsic subsets, Machine learning, RNA gene expression, Systemic sclerosis
Weekly Summary
Receive a weekly summary and discussion of the top papers of the week by leading researchers in the field.