Receive a weekly summary and discussion of the top papers of the week by leading researchers in the field.
Predicting Hypoperfusion Lesion and Target Mismatch in Stroke from Diffusion-weighted MRI Using Deep Learning.
In Radiology ; h5-index 91.0
Yu Yannan, Christensen Soren, Ouyang Jiahong, Scalzo Fabien, Liebeskind David S, Lansberg Maarten G, Albers Gregory W, Zaharchuk Greg
2022-Dec-06
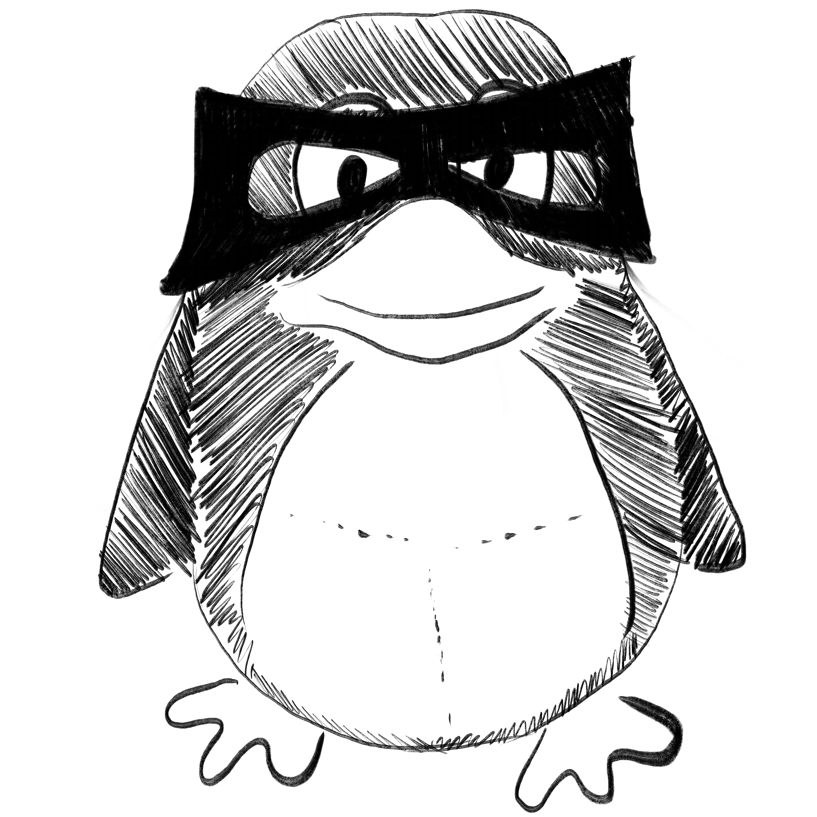
Know Before You Go: Data-Driven Beach Water Quality Forecasting.
In Environmental science & technology ; h5-index 132.0
Searcy Ryan T, Boehm Alexandria B
2022-Dec-06
data-driven models, machine learning, water quality forecasting
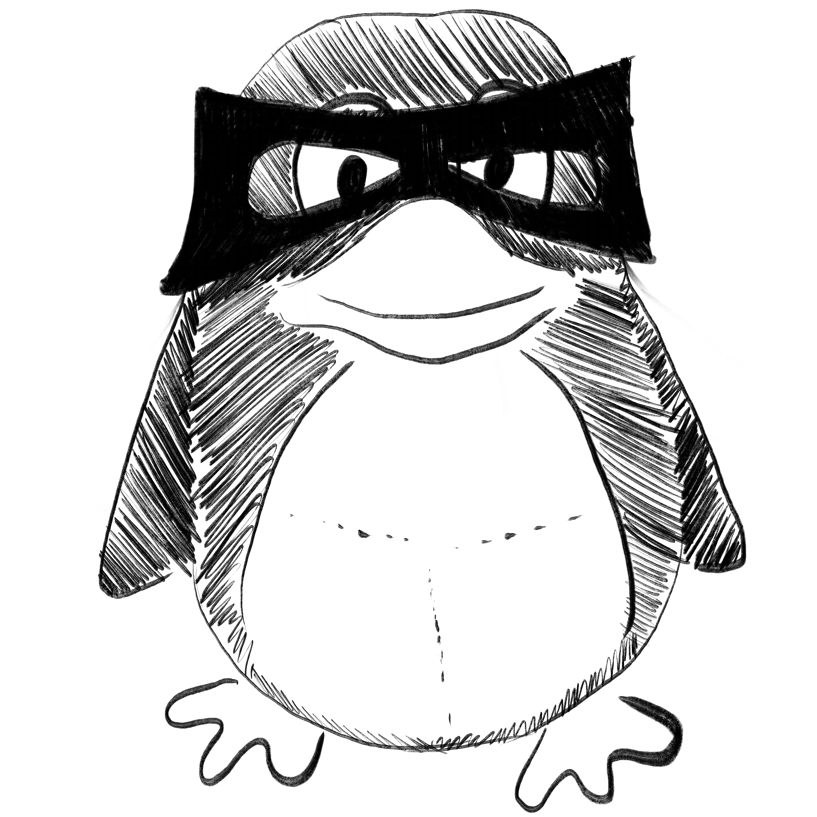
Large Data Set-Driven Machine Learning Models for Accurate Prediction of the Thermoelectric Figure of Merit.
In ACS applied materials & interfaces ; h5-index 147.0
Li Yi, Zhang Jingzi, Zhang Ke, Zhao Mengkun, Hu Kailong, Lin Xi
2022-Dec-06
data-driven, machine learning, the figure of merit, thermoelectric materials, zT prediction
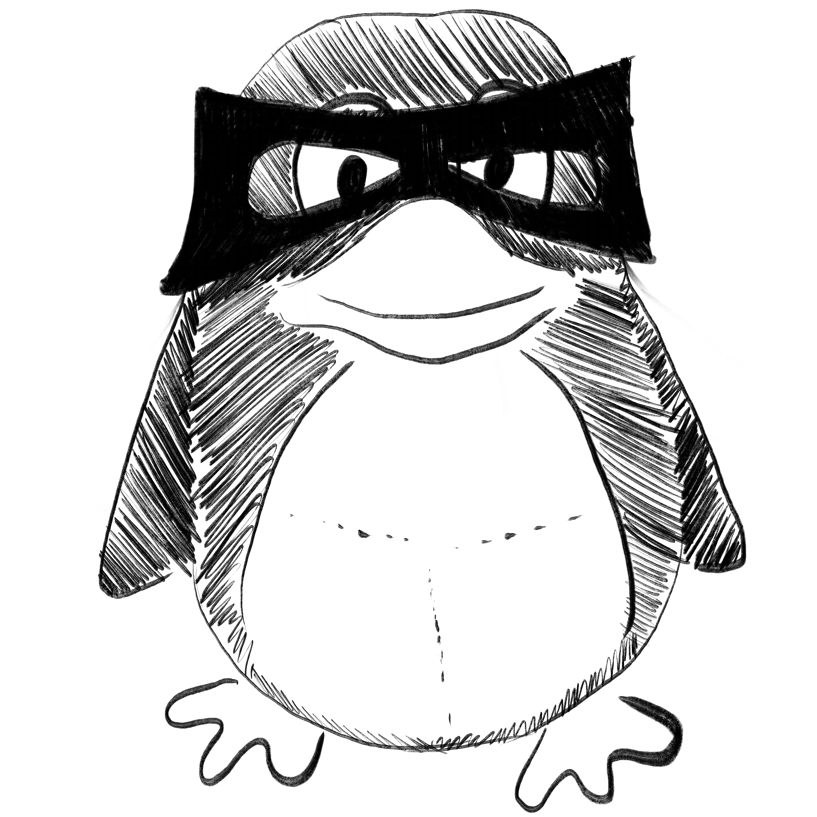
Structural Analysis and Prediction of Hematotoxicity Using Deep Learning Approaches.
In Journal of chemical information and modeling
Long Teng-Zhi, Shi Shao-Hua, Liu Shao, Lu Ai-Ping, Liu Zhao-Qian, Li Min, Hou Ting-Jun, Cao Dong-Sheng
2022-Dec-06
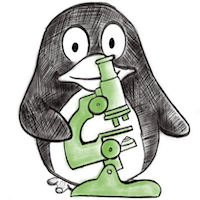
Machine Learning To Stratify Methicillin-Resistant Staphylococcus aureus Risk among Hospitalized Patients with Community-Acquired Pneumonia.
In Antimicrobial agents and chemotherapy ; h5-index 79.0
Rhodes Nathaniel J, Rohani Roxane, Yarnold Paul R, Pawlowski Anna E, Malczynski Michael, Qi Chao, Sutton Sarah H, Zembower Teresa R, Wunderink Richard G
2022-Dec-06
MRSA infection, antibiotic stewardship, community-acquired pneumonia, machine learning, predictive model
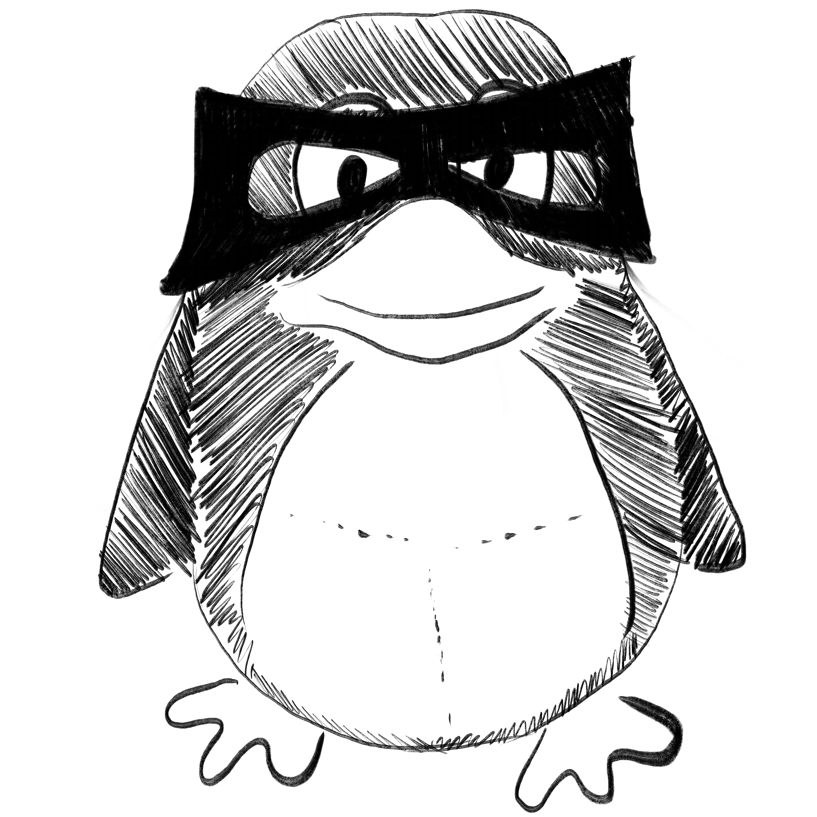
Development of a Peripheral Blood Transcriptomic Gene Signature to Predict Bronchopulmonary Dysplasia.
In American journal of physiology. Lung cellular and molecular physiology
BACKGROUND :
OBJECTIVE :
METHODS :
RESULTS :
CONCLUSIONS :
Moreira Alvaro, Tovar Miriam, Smith Alisha M, Lee Grace C, Meunier Justin A, Cheema Zoya, Moreira Axel, Winter Caitlyn, Mustafa Shamimunisa B, Seidner Steven R, Findley Tina Oak, Garcia Joe G N, Thébaud Bernard, Kwinta Przemko, Ahuja Sunil K
2022-Dec-06
artificial intelligence, bronchopulmonary dysplasia, prediction, preterm neonate, transcriptome
Weekly Summary
Receive a weekly summary and discussion of the top papers of the week by leading researchers in the field.