Receive a weekly summary and discussion of the top papers of the week by leading researchers in the field.
Visual-Linguistic Causal Intervention for Radiology Report Generation
ArXiv Preprint
Weixing Chen, Yang Liu, Ce Wang, Guanbin Li, Jiarui Zhu, Liang Lin
2023-03-16
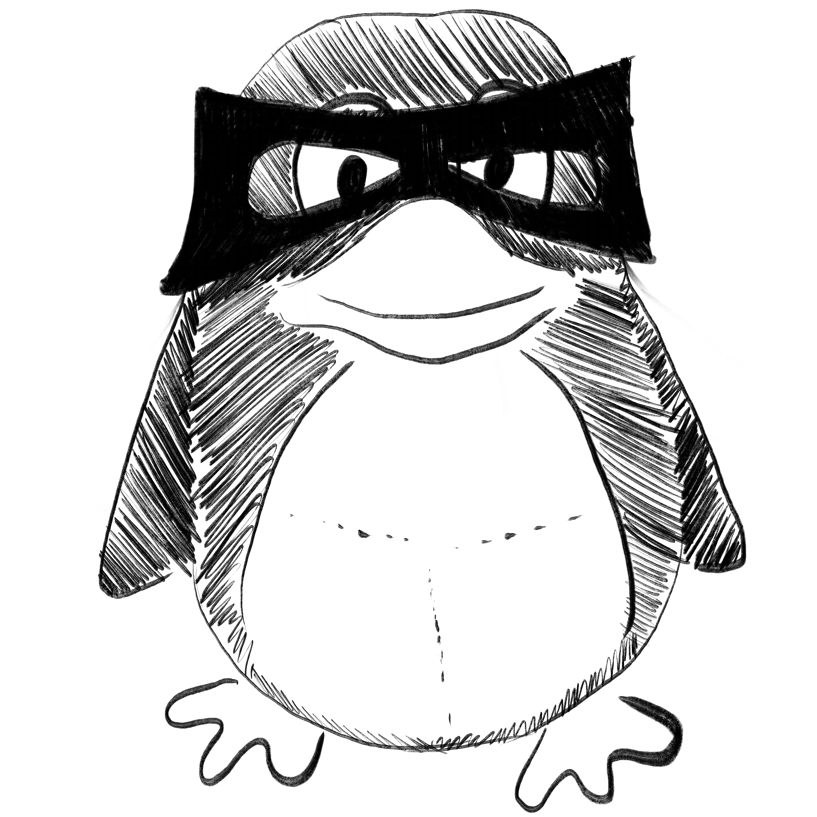
[Artificial intelligence and psychiatry: questions from psychiatrists to ChatGPT].
In Revue medicale suisse
Prada Paco, Perroud Nader, Thorens Gabriel
2023-Mar-15
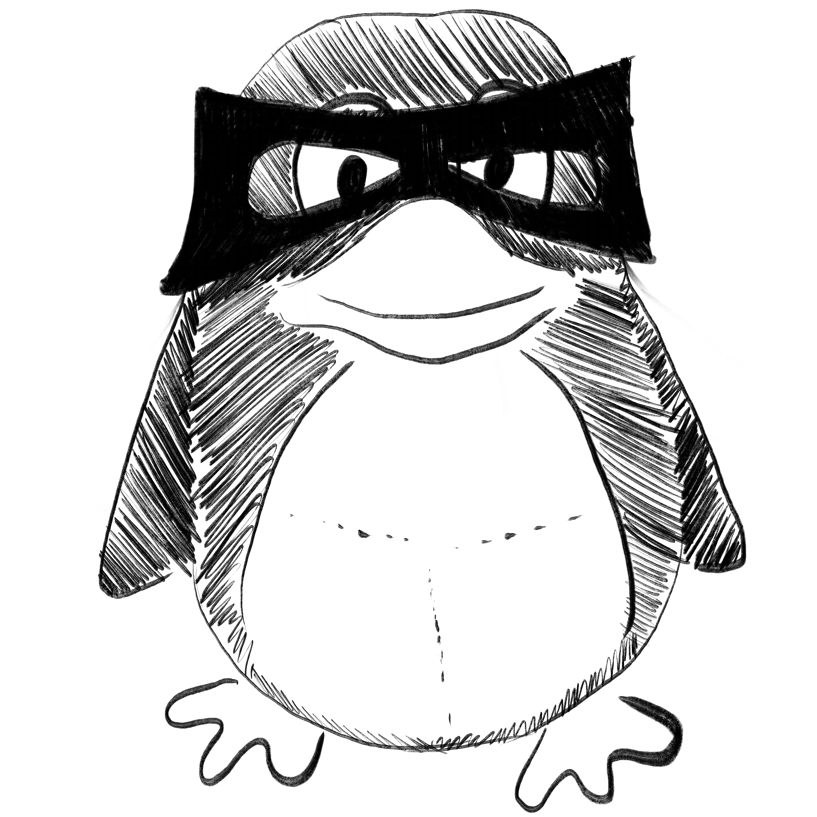
Diode Characteristics in Magnetic Domain Wall Devices via Geometrical Pinning for Neuromorphic Computing.
In ACS applied materials & interfaces ; h5-index 147.0
Rahaman Hasibur, Kumar Durgesh, Chung Hong Jing, Maddu Ramu, Lim Sze Ter, Jin Tianli, Piramanayagam S N
2023-Mar-15
asymmetric pinning potential, domain wall devices, domain wall diodes, neuromorphic computing, pine-tree devices, spināorbit torque, synapses
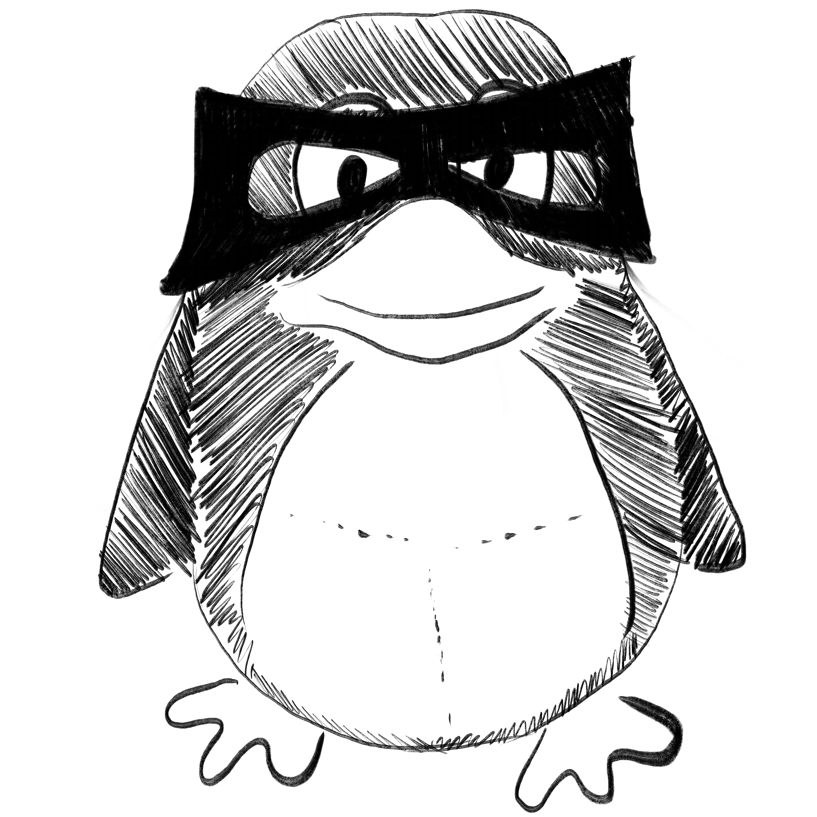
A methylation clock model of mild SARS-CoV-2 infection provides insight into immune dysregulation.
In Molecular systems biology
Mao Weiguang, Miller Clare M, Nair Venugopalan D, Ge Yongchao, Amper Mary Anne S, Cappuccio Antonio, George Mary-Catherine, Goforth Carl W, Guevara Kristy, Marjanovic Nada, Nudelman German, Pincas Hanna, Ramos Irene, Sealfon Rachel S G, Soares-Schanoski Alessandra, Vangeti Sindhu, Vasoya Mital, Weir Dawn L, Zaslavsky Elena, Kim-Schulze Seunghee, Gnjatic Sacha, Merad Miriam, Letizia Andrew G, Troyanskaya Olga G, Sealfon Stuart C, Chikina Maria
2023-Mar-15
DNA methylation, SARS-CoV-2, machine learning model, temporal dynamics, trained immunity
Prediction of Future Caries in 1-Year-Old Children via the Salivary Microbiome.
In Journal of dental research ; h5-index 65.0
Raksakmanut R, Thanyasrisung P, Sritangsirikul S, Kitsahawong K, Seminario A L, Pitiphat W, Matangkasombut O
2023-Mar-15
biomarkers, dental caries, infant, machine learning, microbiota, saliva
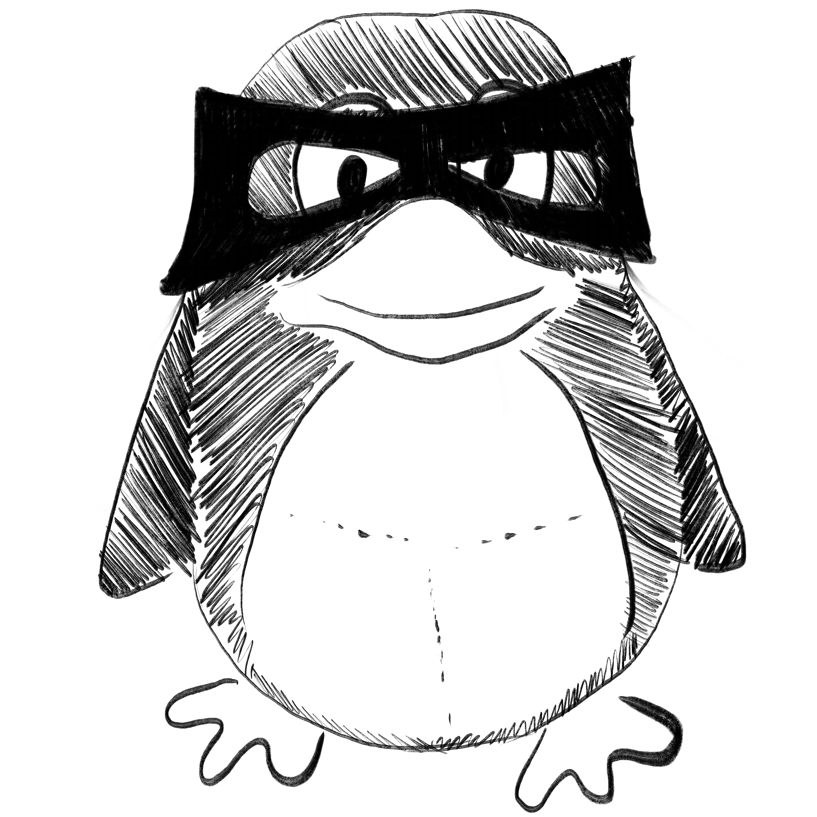
Developing a machine learning model to detect diagnostic uncertainty in clinical documentation.
In Journal of hospital medicine
BACKGROUND AND OBJECTIVE :
DESIGN, SETTING AND PARTICIPANTS :
RESULTS :
CONCLUSION :
Marshall Trisha L, Nickels Lindsay C, Brady Patrick W, Edgerton Ezra J, Lee James J, Hagedorn Philip A
2023-Mar-15
Weekly Summary
Receive a weekly summary and discussion of the top papers of the week by leading researchers in the field.