Receive a weekly summary and discussion of the top papers of the week by leading researchers in the field.
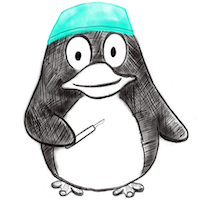
Using Machine Learning and Deep Learning Algorithms to Predict Postoperative Outcomes Following Anterior Cervical Discectomy and Fusion.
In Clinical spine surgery
STUDY DESIGN :
OBJECTIVE :
SUMMARY OF BACKGROUND DATA :
METHODS :
RESULTS :
CONCLUSIONS :
LEVEL OF EVIDENCE :
Khazanchi Rushmin, Bajaj Anitesh, Shah Rohan M, Chen Austin R, Reyes Samuel G, Kurapaty Steven S, Hsu Wellington K, Patel Alpesh A, Divi Srikanth N
2023-Mar-13
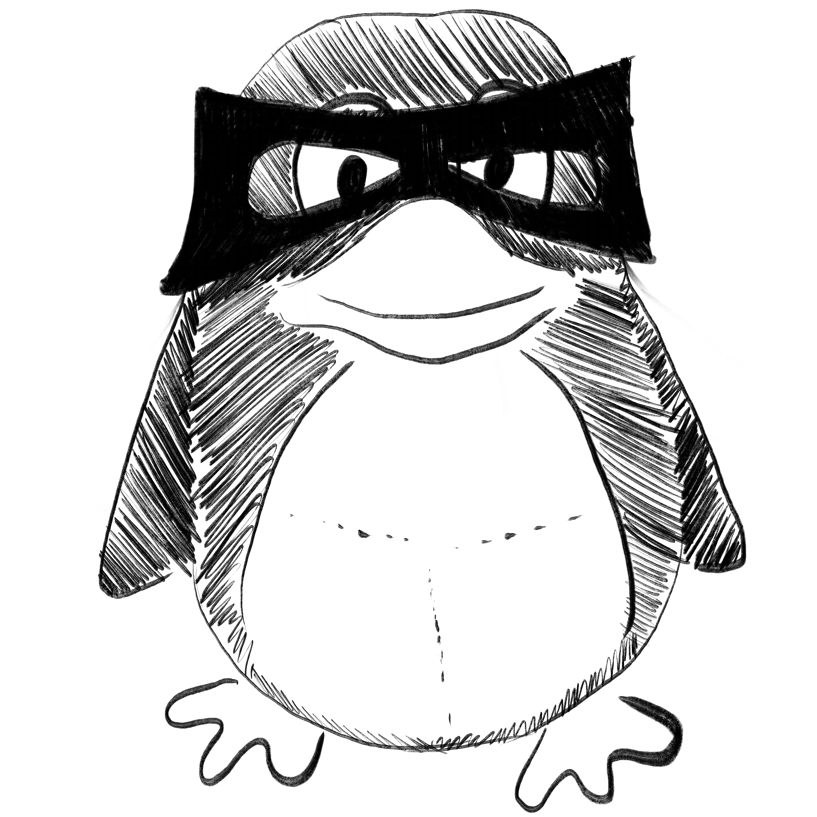
Prediction of wear of dental composite materials using machine learning algorithms.
In Computer methods in biomechanics and biomedical engineering
Suryawanshi Abhijeet, Behera Niranjana
2023-Mar-15
K-nearest neighbors wear, Machine learning, dental composite, extreme gradient boosting, multi-layer perception
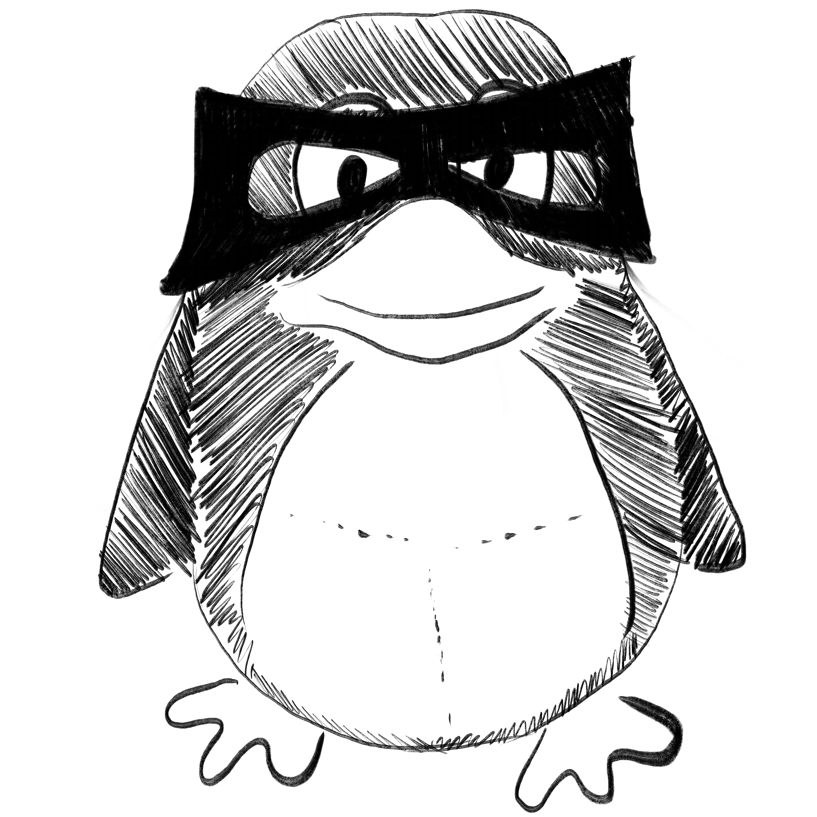
Active fault tolerant deep brain stimulator for epilepsy using deep neural network.
In Biomedizinische Technik. Biomedical engineering
Senthilvelmurugan Nambi Narayanan, Subbian Sutha
2023-Mar-16
AFTDBS, DNN, HH model, MPC, PI, RDBS, epileptic seizure, machine learning algorithm
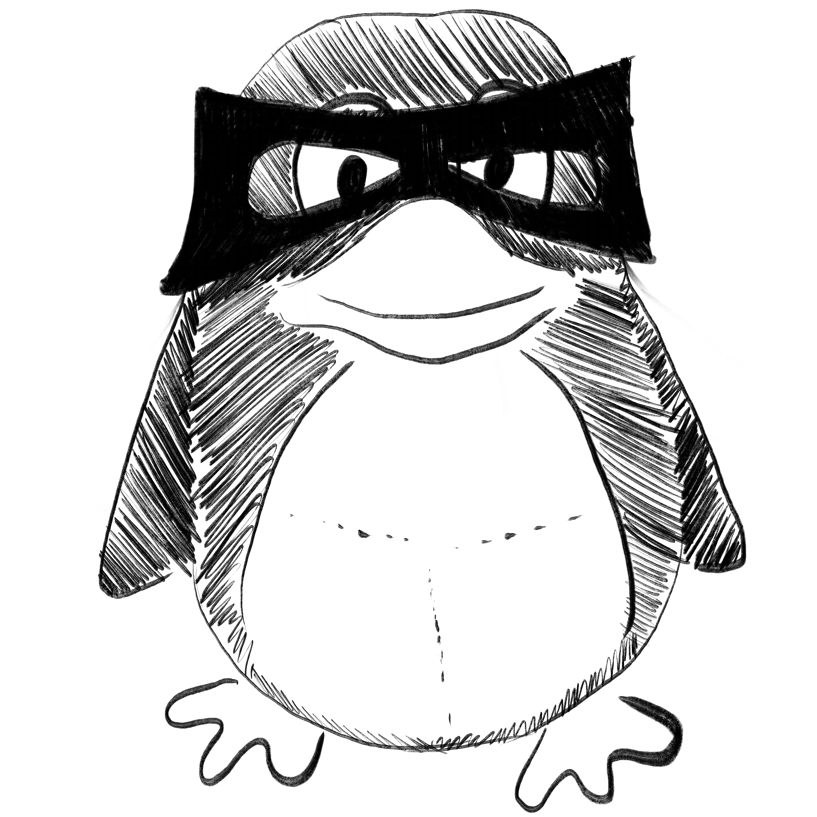
Inter-domain distance prediction based on deep learning for domain assembly.
In Briefings in bioinformatics
Ge Fengqi, Peng Chunxiang, Cui Xinyue, Xia Yuhao, Zhang Guijun
2023-Mar-15
deep learning, domain assembly, inter-domain distance, multi-domain protein
Are deep models in radiomics performing better than generic models? A systematic review.
In European radiology experimental
BACKGROUND :
METHODS :
RESULTS :
CONCLUSIONS :
Demircioğlu Aydin
2023-Mar-15
Artificial intelligence, Deep learning, Machine learning, Radiology, Radiomics
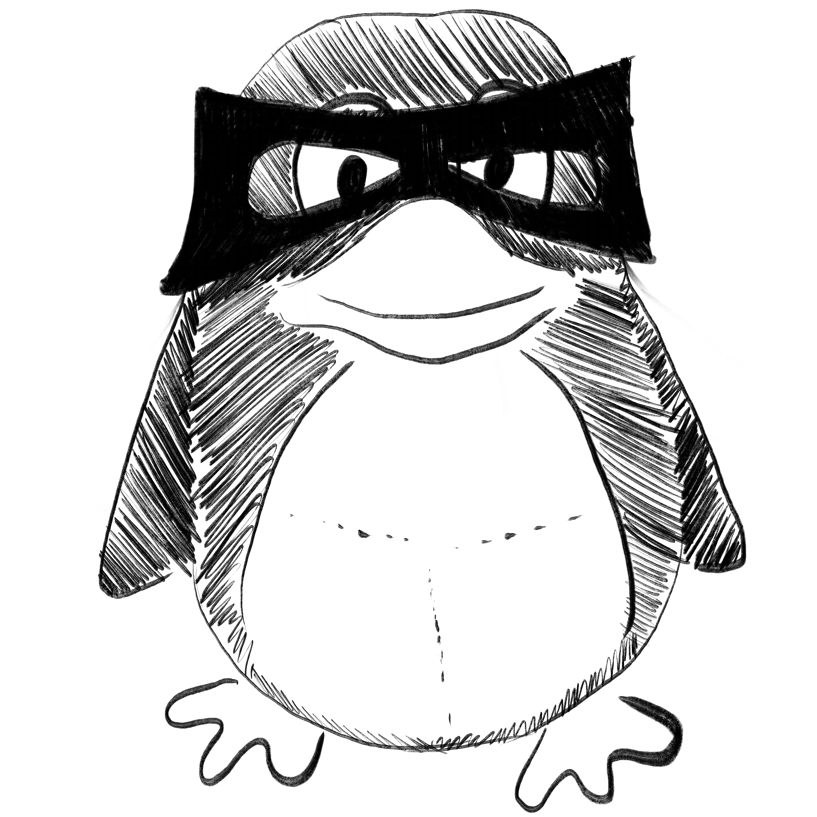
A methylation clock model of mild SARS-CoV-2 infection provides insight into immune dysregulation.
In Molecular systems biology
Mao Weiguang, Miller Clare M, Nair Venugopalan D, Ge Yongchao, Amper Mary Anne S, Cappuccio Antonio, George Mary-Catherine, Goforth Carl W, Guevara Kristy, Marjanovic Nada, Nudelman German, Pincas Hanna, Ramos Irene, Sealfon Rachel S G, Soares-Schanoski Alessandra, Vangeti Sindhu, Vasoya Mital, Weir Dawn L, Zaslavsky Elena, Kim-Schulze Seunghee, Gnjatic Sacha, Merad Miriam, Letizia Andrew G, Troyanskaya Olga G, Sealfon Stuart C, Chikina Maria
2023-Mar-15
DNA methylation, SARS-CoV-2, machine learning model, temporal dynamics, trained immunity
Weekly Summary
Receive a weekly summary and discussion of the top papers of the week by leading researchers in the field.