Receive a weekly summary and discussion of the top papers of the week by leading researchers in the field.
Deep learning for automated, interpretable classification of lumbar spinal stenosis and facet arthropathy from axial MRI.
In European radiology ; h5-index 62.0
OBJECTIVES :
METHODS :
RESULTS :
CONCLUSION :
KEY POINTS :
Bharadwaj Upasana Upadhyay, Christine Miranda, Li Steven, Chou Dean, Pedoia Valentina, Link Thomas M, Chin Cynthia T, Majumdar Sharmila
2023-Mar-15
Arthropathy, Deep learning, MRI, Stenosis
Contrast-enhanced CT radiomics improves the prediction of abdominal aortic aneurysm progression.
In European radiology ; h5-index 62.0
OBJECTIVES :
METHODS :
RESULTS :
CONCLUSION :
KEY POINTS :
Wang Yan, Xiong Fei, Leach Joseph, Kao Evan, Tian Bing, Zhu Chengcheng, Zhang Yue, Hope Michael, Saloner David, Mitsouras Dimitrios
2023-Mar-15
Abdominal aortic aneurysm, Computed tomography, Machine learning, Thrombus
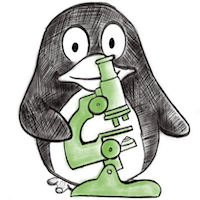
Expanding inclusion criteria for active surveillance in intermediate-risk prostate cancer: a machine learning approach.
In World journal of urology ; h5-index 40.0
PURPOSE :
METHODS :
RESULTS :
CONCLUSIONS :
Baboudjian Michael, Breda Alberto, Roumeguère Thierry, Uleri Alessandro, Roche Jean-Baptiste, Touzani Alae, Lacetera Vito, Beauval Jean-Baptiste, Diamand Romain, Simone Guiseppe, Windisch Olivier, Benamran Daniel, Fourcade Alexandre, Fiard Gaelle, Durand-Labrunie Camille, Roumiguié Mathieu, Sanguedolce Francesco, Oderda Marco, Barret Eric, Fromont Gaëlle, Dariane Charles, Charvet Anne-Laure, Gondran-Tellier Bastien, Bastide Cyrille, Lechevallier Eric, Palou Joan, Ruffion Alain, Van Der Bergh Roderick C N, Peltier Alexandre, Ploussard Guillaume
2023-Mar-15
Active surveillance, Intermediate risk, Machine learning, Oncological outcomes, Prostate cancer
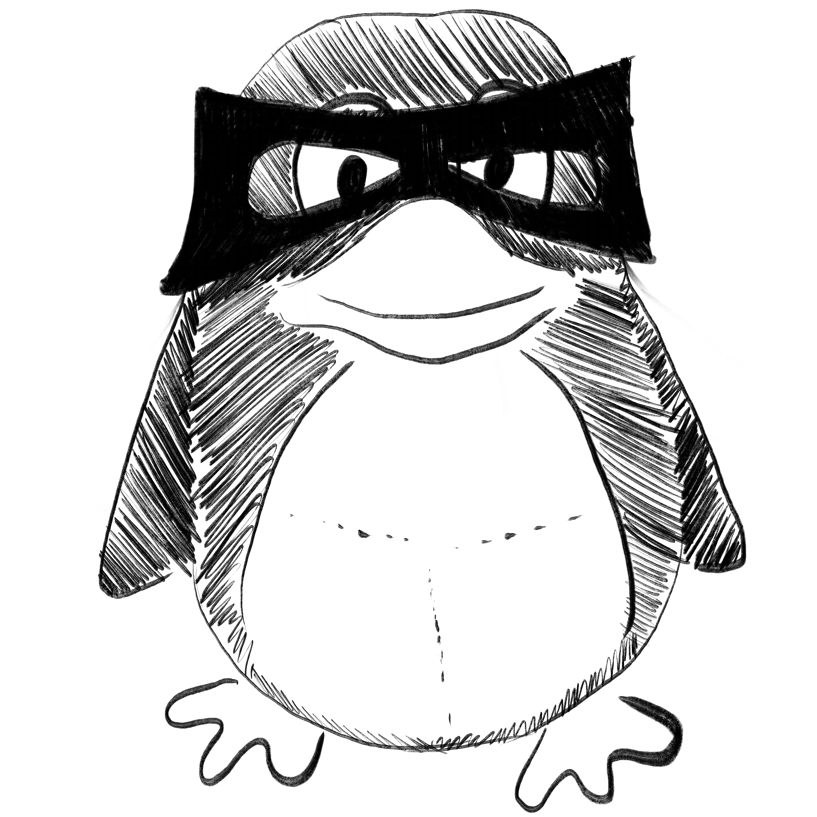
Smartphone and Wearable Sensors for the Estimation of Facioscapulohumeral Muscular Dystrophy Disease Severity: Cross-sectional Study.
In JMIR formative research
BACKGROUND :
OBJECTIVE :
METHODS :
RESULTS :
CONCLUSIONS :
TRIAL REGISTRATION :
Zhuparris Ahnjili, Maleki Ghobad, Koopmans Ingrid, Doll Robert J, Voet Nicoline, Kraaij Wessel, Cohen Adam, van Brummelen Emilie, De Maeyer Joris H, Groeneveld Geert Jan
2023-Mar-15
FSHD, Time Up and Go, facioscapulohumeral muscular dystrophy, mHealth, machine learning, mobile health, mobile phone, neuromuscular disease, regression, smartphone, wearables
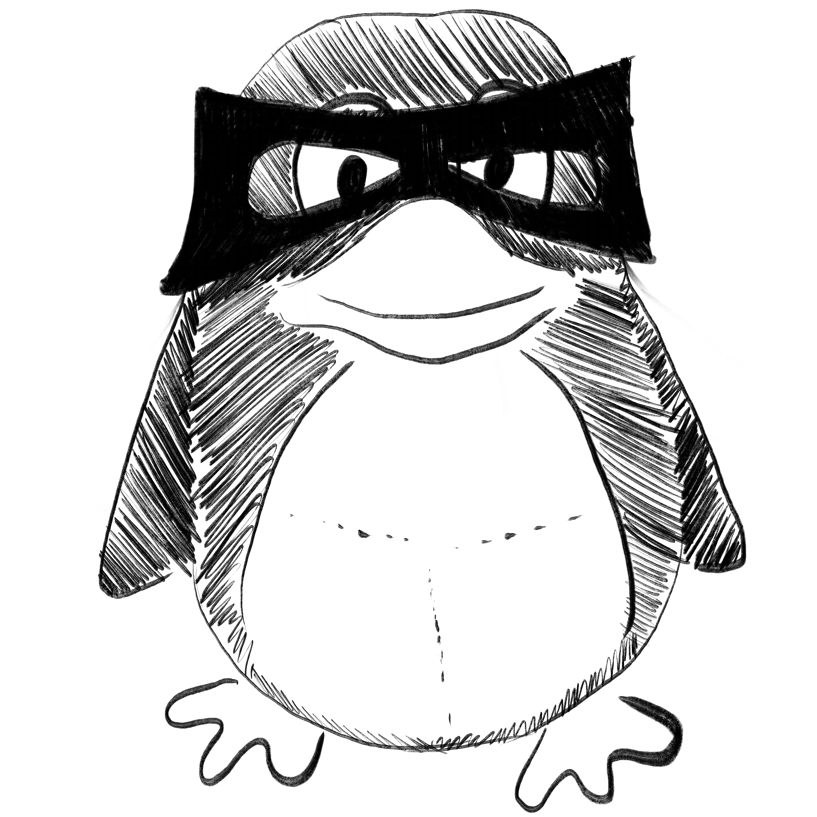
Objective Prediction of Next-Day's Affect Using Multimodal Physiological and Behavioral Data: Algorithm Development and Validation Study.
In JMIR formative research
BACKGROUND :
OBJECTIVE :
METHODS :
RESULTS :
CONCLUSIONS :
Jafarlou Salar, Lai Jocelyn, Azimi Iman, Mousavi Zahra, Labbaf Sina, Jain Ramesh C, Dutt Nikil, Borelli Jessica L, Rahmani Amir
2023-Mar-15
affective computing, mental health, wearable devices
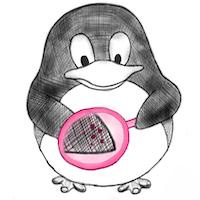
Development and Clinical Evaluation of an Artificial Intelligence Support Tool for Improving Telemedicine Photo Quality.
In JAMA dermatology ; h5-index 54.0
IMPORTANCE :
OBJECTIVE :
DESIGN, SETTING, AND PARTICIPANTS :
INTERVENTIONS :
MAIN OUTCOMES AND MEASURES :
RESULTS :
CONCLUSIONS AND RELEVANCE :
Vodrahalli Kailas, Ko Justin, Chiou Albert S, Novoa Roberto, Abid Abubakar, Phung Michelle, Yekrang Kiana, Petrone Paige, Zou James, Daneshjou Roxana
2023-Mar-15
Weekly Summary
Receive a weekly summary and discussion of the top papers of the week by leading researchers in the field.