Receive a weekly summary and discussion of the top papers of the week by leading researchers in the field.
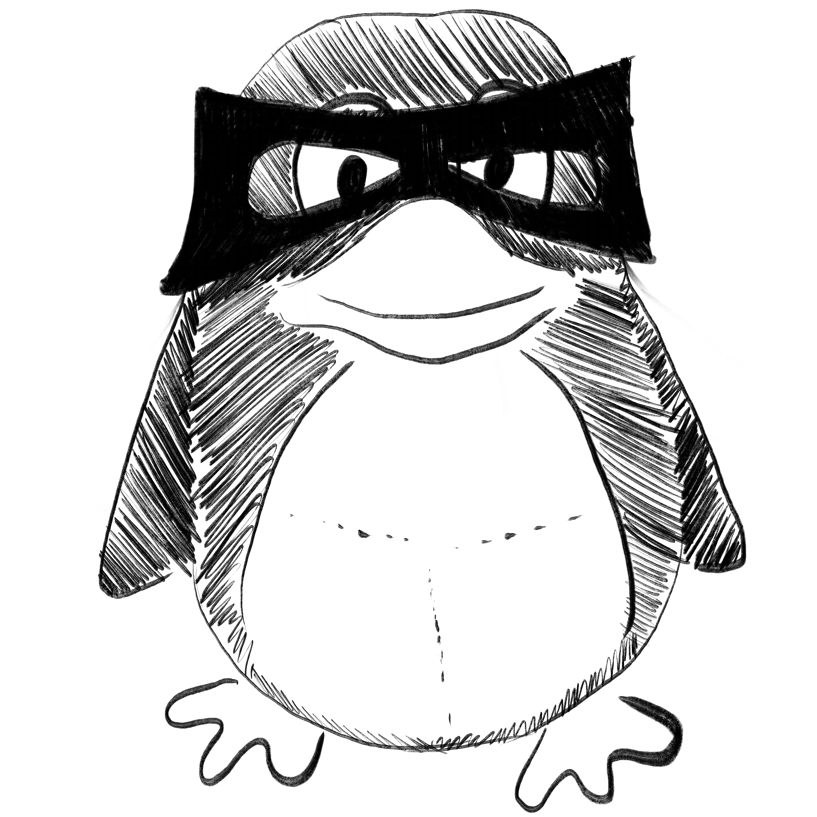
Connecting higher education to workplace activities and earnings.
In PloS one ; h5-index 176.0
Chau Hung, Bana Sarah H, Bouvier Baptiste, Frank Morgan R
2023
Deep Learning for Synthetic CT from Bone MRI in the Head and Neck.
In AJNR. American journal of neuroradiology
BACKGROUND AND PURPOSE :
MATERIALS AND METHODS :
RESULTS :
CONCLUSIONS :
Bambach S, Ho M-L
2022-Aug
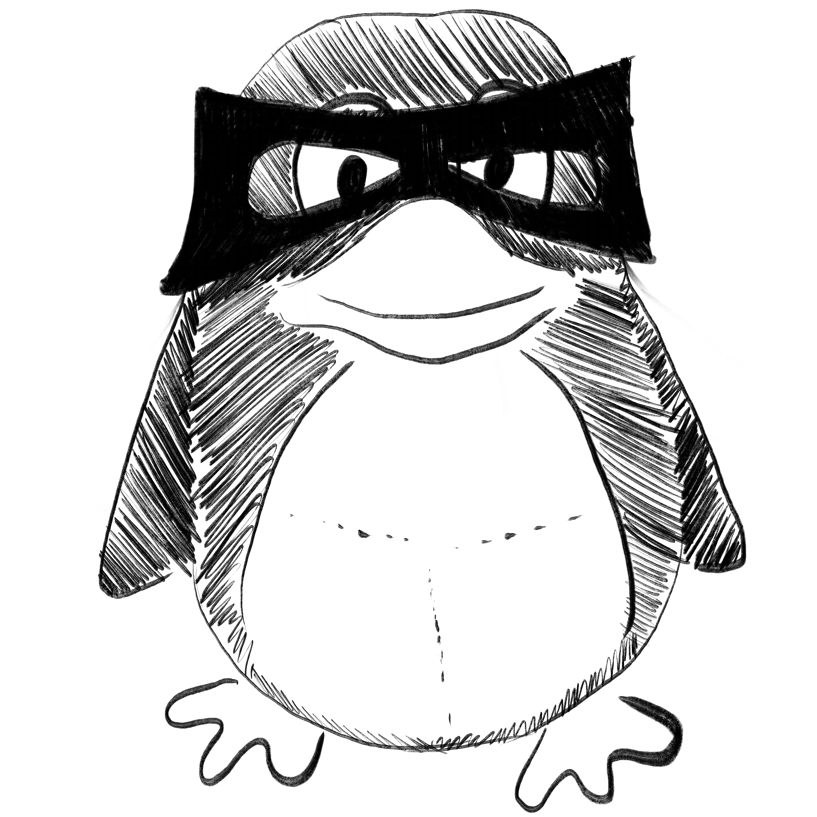
Correlation analysis of deep learning methods in S-ICD screening.
In Annals of noninvasive electrocardiology : the official journal of the International Society for Holter and Noninvasive Electrocardiology, Inc
BACKGROUND :
METHODS :
RESULTS :
CONCLUSION :
ElRefai Mohamed, Abouelasaad Mohamed, Wiles Benedict M, Dunn Anthony J, Coniglio Stefano, Zemkoho Alain B, Morgan John, Roberts Paul R
2023-Mar-15
deep learning tools, screening, subcutaneous implantable cardiac defibrillators
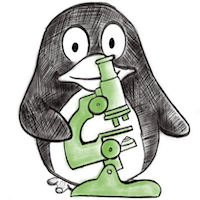
Single-cell Sequence Analysis Combined with Multiple Machine Learning to Identify Markers in Sepsis Patients: LILRA5.
In Inflammation
Ning Jingyuan, Fan Xiaoqing, Sun Keran, Wang Xuan, Li Hongru, Jia Keqi, Ma Cuiqing
2023-Mar-15
LILRA5, machine learning, molecular docking, sepsis, single cell
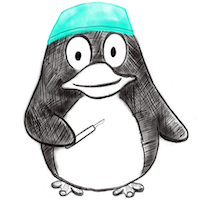
Unlocking the microbial studies through computational approaches: how far have we reached?
In Environmental science and pollution research international
Kumar Rajnish, Yadav Garima, Kuddus Mohammed, Ashraf Ghulam Md, Singh Rachana
2023-Mar-15
Artificial intelligence, Deep learning, Machine learning, Metagenomics, Microbiology
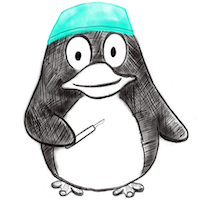
ResMIBCU-Net: an encoder-decoder network with residual blocks, modified inverted residual block, and bi-directional ConvLSTM for impacted tooth segmentation in panoramic X-ray images.
In Oral radiology
OBJECTIVE :
METHODS :
RESULTS :
CONCLUSION :
Imak Andaç, Çelebi Adalet, Polat Onur, Türkoğlu Muammer, Şengür Abdulkadir
2023-Mar-15
Deep learning, Encoder–decoder network, Impacted tooth detection, Oral health, Panoramic radiography
Weekly Summary
Receive a weekly summary and discussion of the top papers of the week by leading researchers in the field.