Receive a weekly summary and discussion of the top papers of the week by leading researchers in the field.
Demographic characteristics, clinical symptoms, biochemical markers and probability of occurrence of severe dengue: A multicenter hospital-based study in Bangladesh.
In PLoS neglected tropical diseases ; h5-index 79.0
Yang Jingli, Mosabbir Abdullah Al, Raheem Enayetur, Hu Wenbiao, Hossain Mohammad Sorowar
2023-Mar-15
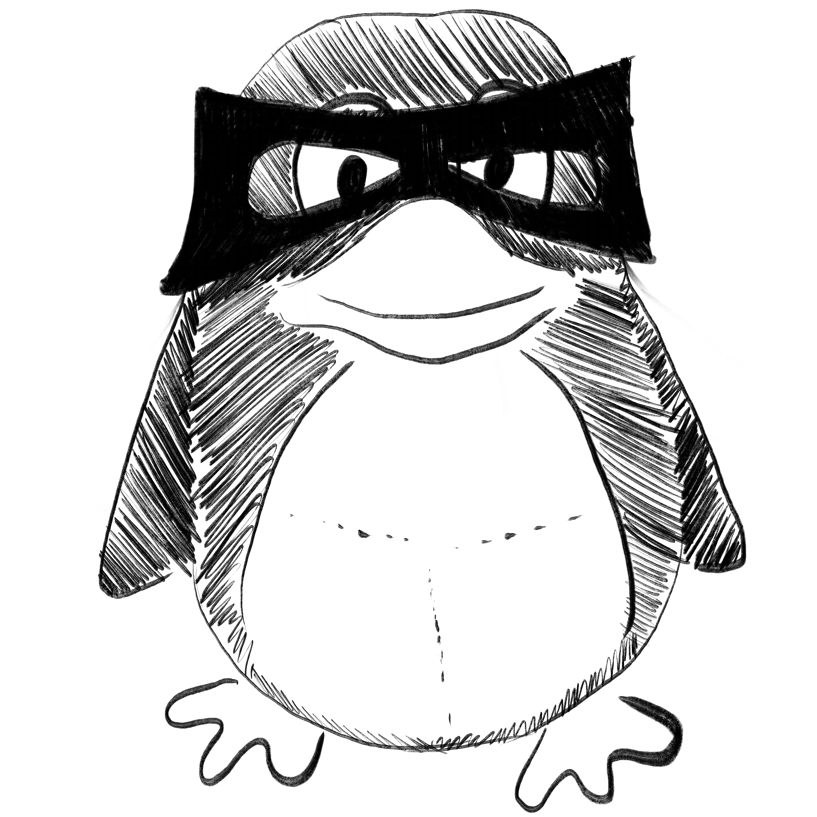
A combinatorial approach to uncover an additional Integrator subunit.
In Cell reports ; h5-index 119.0
Offley Sarah R, Pfleiderer Moritz M, Zucco Avery, Fraudeau Angelique, Welsh Sarah A, Razew Michal, Galej Wojciech P, Gardini Alessandro
2023-Mar-13
AlphaFold2, CP: Neuroscience, Integrator complex, RNA polymerase II, RNA polymerase II pausing, large protein complexes, molecular modeling, omics, pause-release, transcription, transcription factors, transcriptional regulation
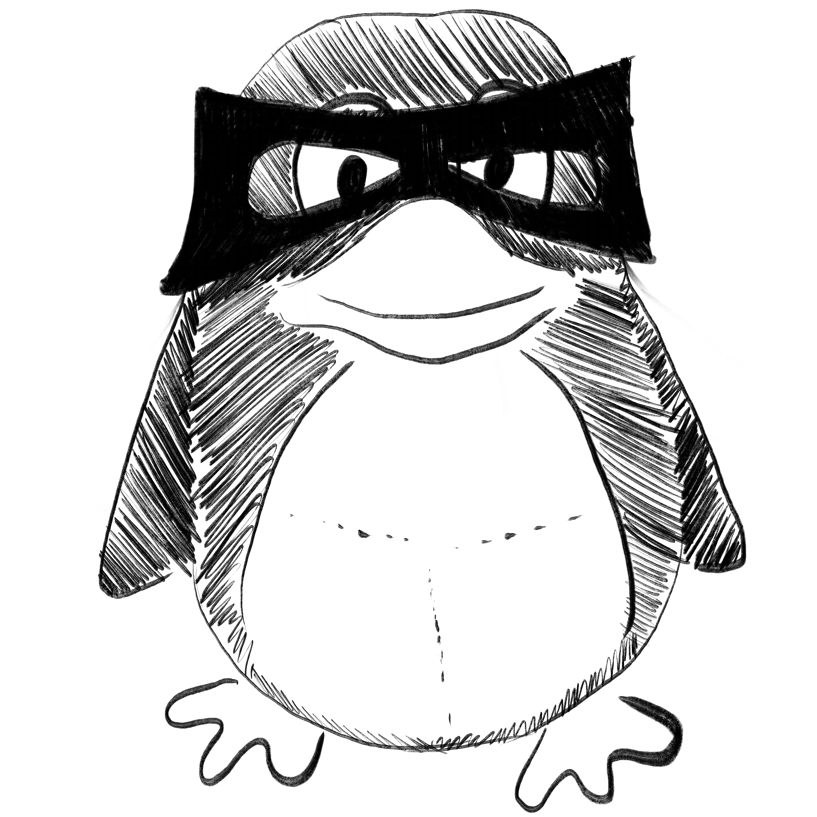
Development and validation of deep learning based embryo selection across multiple days of transfer.
In Scientific reports ; h5-index 158.0
Theilgaard Lassen Jacob, Fly Kragh Mikkel, Rimestad Jens, Nygård Johansen Martin, Berntsen Jørgen
2023-Mar-14
Investigation of liquid biopsy analytes in peripheral blood of individuals after SARS-CoV-2 infection.
In EBioMedicine
BACKGROUND :
METHODS :
FINDINGS :
INTERPRETATION :
FUNDING :
Qi Elizabeth, Courcoubetis George, Liljegren Emmett, Herrera Ergueen, Nguyen Nathalie, Nadri Maimoona, Ghandehari Sara, Kazemian Elham, Reckamp Karen L, Merin Noah M, Merchant Akil, Mason Jeremy, Figueiredo Jane C, Shishido Stephanie N, Kuhn Peter
2023-Mar-13
COVID-19, Liquid biopsy, Long COVID, Post-COVID sequelae, Post-acute COVID-19 syndrome (PACS), SARS-CoV-2
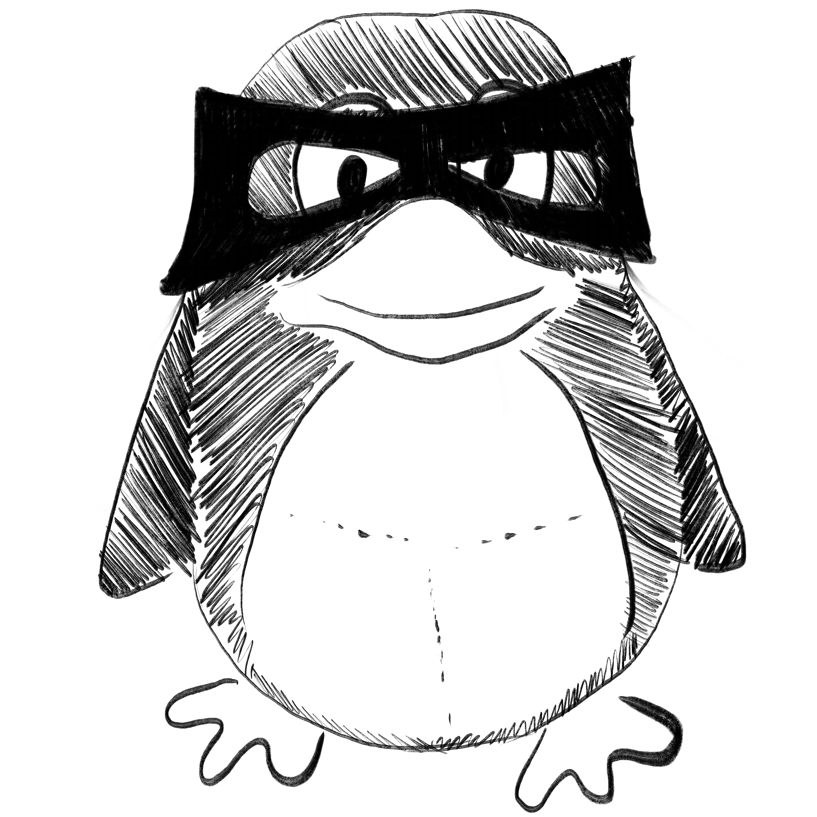
Plant Disease Detection using Region-Based Convolutional Neural Network
ArXiv Preprint
Hasin Rehana, Muhammad Ibrahim, Md. Haider Ali
2023-03-16
Geometric and dosimetric evaluation of deep learning based auto-segmentation for clinical target volume on breast cancer.
In Journal of applied clinical medical physics ; h5-index 28.0
BACKGROUND :
MATERIALS AND METHODS :
RESULTS :
CONCLUSIONS :
Zhong Yang, Guo Ying, Fang Yingtao, Wu Zhiqiang, Wang Jiazhou, Hu Weigang
2023-Mar-15
auto-segmentation, dosimetric impact, geometric metrics, radiotherapy target
Weekly Summary
Receive a weekly summary and discussion of the top papers of the week by leading researchers in the field.