Receive a weekly summary and discussion of the top papers of the week by leading researchers in the field.
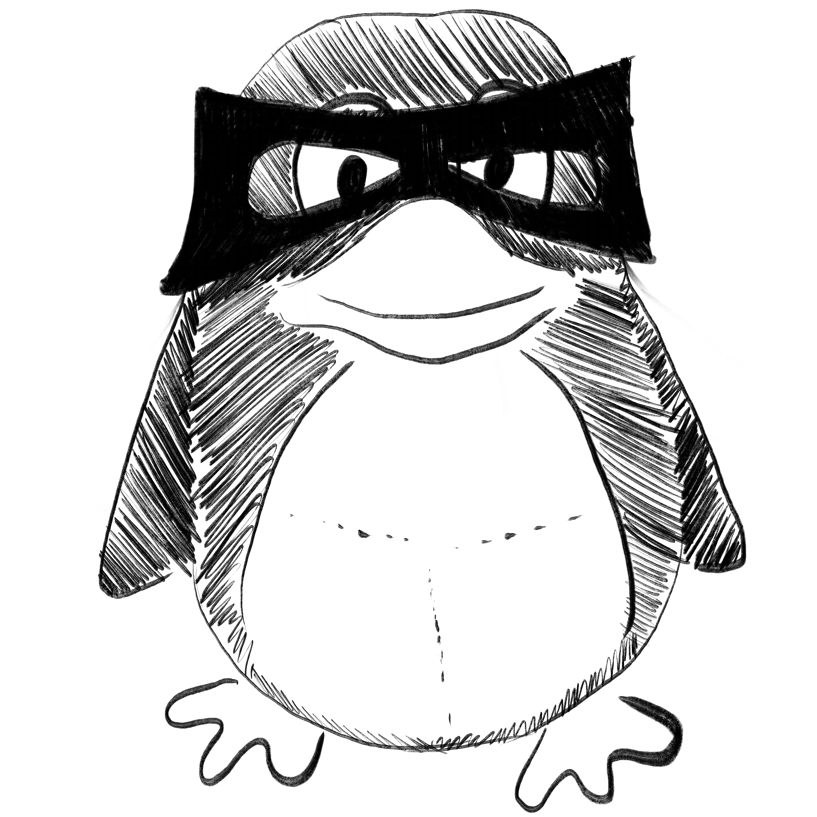
Investigating the neural correlates of affective mentalizing and their association with general intelligence in patients with schizophrenia.
In Schizophrenia research ; h5-index 61.0
BACKGROUND AND HYPOTHESIS :
STUDY DESIGN :
STUDY RESULTS :
CONCLUSIONS :
Tantchik Wladimir, Green Melissa J, Quidé Yann, Erk Susanne, Mohnke Sebastian, Wackerhagen Carolin, Romanczuk-Seiferth Nina, Tost Heike, Schwarz Kristina, Moessnang Carolin, Bzdok Danilo, Meyer-Lindenberg Andreas, Heinz Andreas, Walter Henrik
2023-Mar-13
Mentalizing, Social cognition, fMRI, mPFC
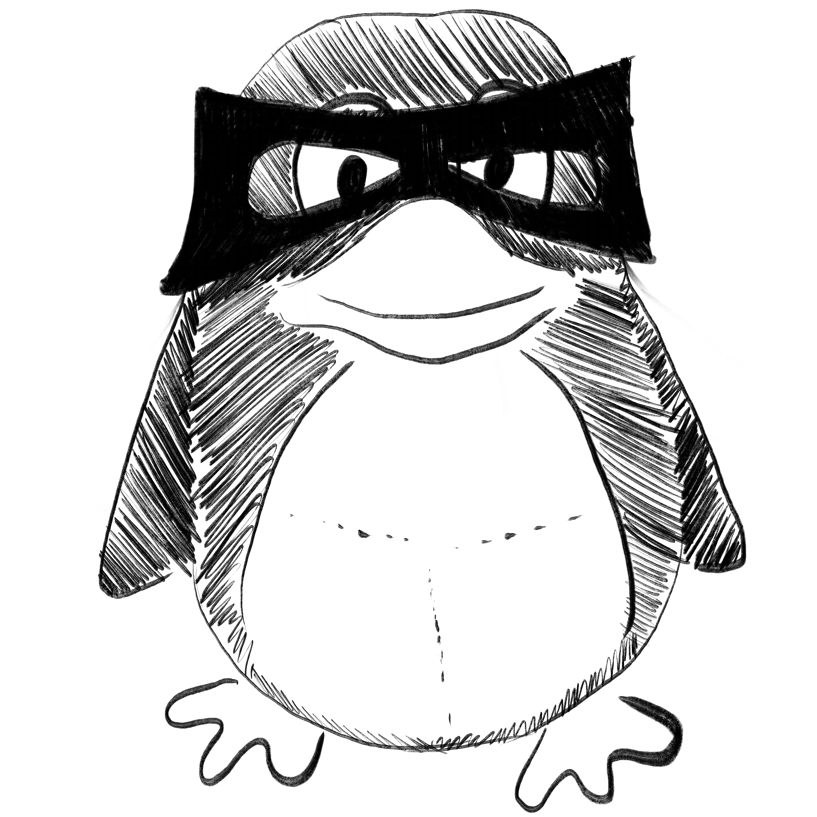
Review: Recent advancements and moving trends in chemical analysis of fire debris.
In Forensic science international
Low YuanTing, Tyrrell Eadaoin, Gillespie Eoin, Quigley Cormac
2023-Feb-28
Accelerants, Arson investigation, Chemometrics, Destructive techniques, Fire debris analysis, Ignitable liquids, Non-destructive techniques
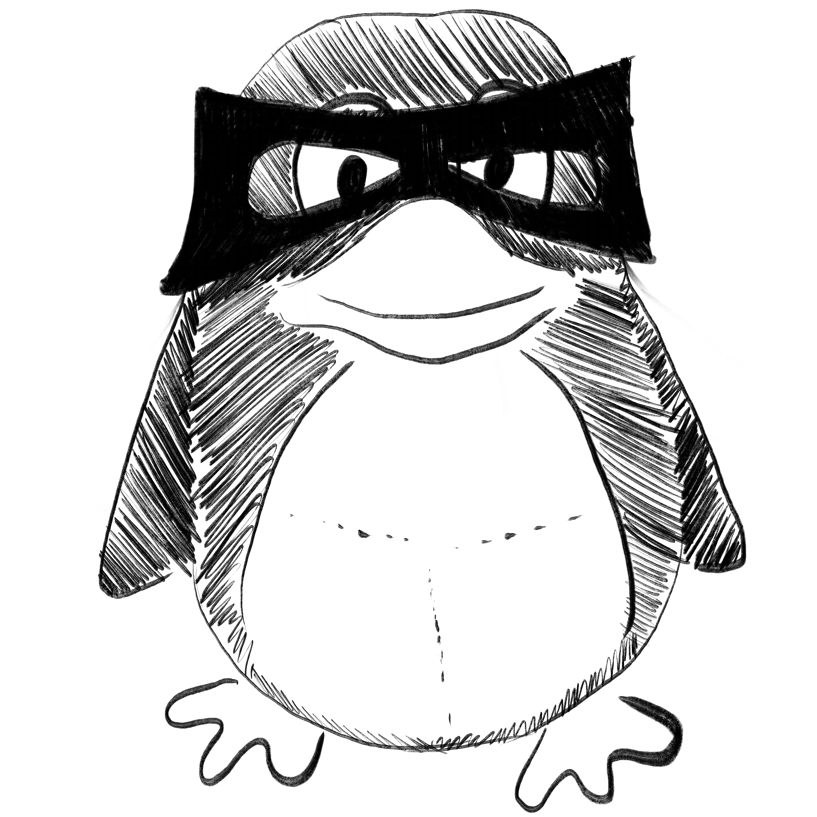
High-efficient Bloch simulation of magnetic resonance imaging sequences based on deep learning.
In Physics in medicine and biology
OBJECTIVE :
APPROACH :
MAIN RESULTS :
SIGNIFICANCE :
Huang Haitao, Yang Qinqin, Wang Jiechao, Zhang Pujie, Cai Shuhui, Cai Congbo
2023-Mar-15
Bloch simulation, deep learning, dynamic convolution, magnetic resonance imaging, synthetic data generation
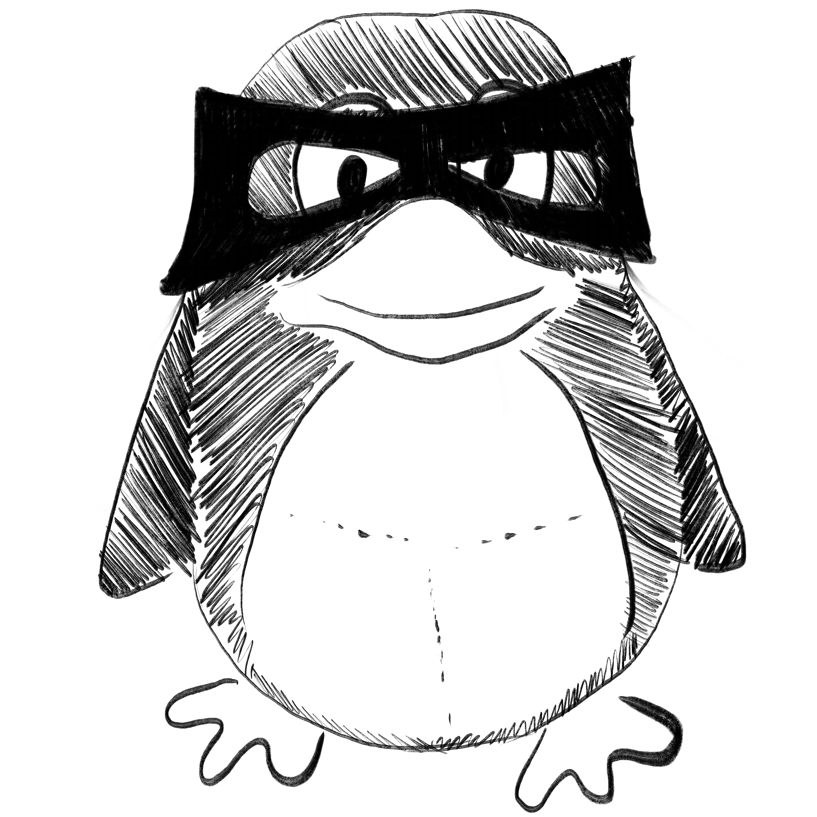
[Artificial intelligence in medicine: present and future].
In Gaceta medica de Mexico
Lanzagorta-Ortega Dioselina, Carrillo-Pérez Diego L, Carrillo-Esper Raúl
2022-Dec-15
Artificial intelligence, Medical education, Medical errors, Patient care, Precision medicine
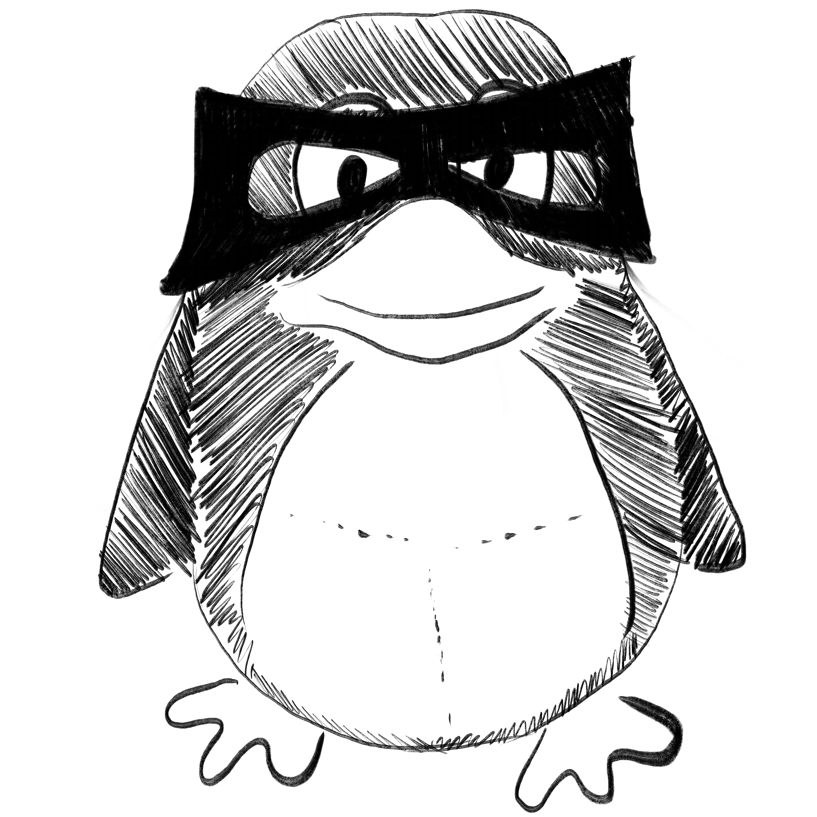
Emergency department use and Artificial Intelligence in Pelotas: design and baseline results.
In Revista brasileira de epidemiologia = Brazilian journal of epidemiology
OBJETIVO :
METHODS :
RESULTS :
CONCLUSION :
Delpino Felipe Mendes, Figueiredo Lílian Munhoz, Costa Ândria Krolow, Carreno Ioná, Silva Luan Nascimento da, Flores Alana Duarte, Pinheiro Milena Afonso, Silva Eloisa Porciúncula da, Marques Gabriela Ávila, Saes Mirelle de Oliveira, Duro Suele Manjourany Silva, Facchini Luiz Augusto, Vissoci João Ricardo Nickenig, Flores Thaynã Ramos, Demarco Flávio Fernando, Blumenberg Cauane, Chiavegatto Filho Alexandre Dias Porto, Silva Inácio Crochemore da, Batista Sandro Rodrigues, Arcêncio Ricardo Alexandre, Nunes Bruno Pereira
2023
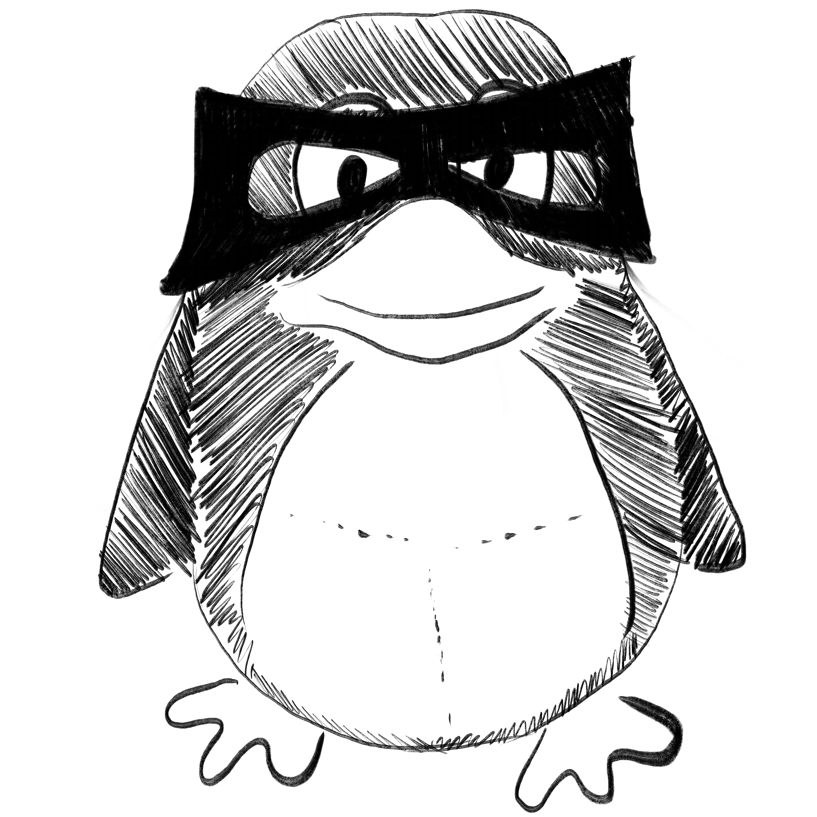
Exploring the intersectionality of characteristics among those who experienced opioid overdoses: A cluster analysis.
In Health reports
BACKGROUND :
DATA AND METHODS :
RESULTS :
INTERPRETATION :
Chu Kenneth, Carrière Gisèle, Garner Rochelle, Bosa Kevin, Hennessy Deirdre, Sanmartin Claudia
2023-Mar-15
cluster analysis, intersectionality, linked data, opioid overdose
Weekly Summary
Receive a weekly summary and discussion of the top papers of the week by leading researchers in the field.