Receive a weekly summary and discussion of the top papers of the week by leading researchers in the field.
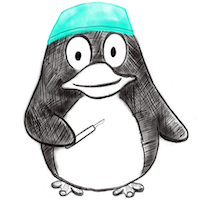
Automatic registration of dental CT and 3D scanned model using deep split jaw and surface curvature.
In Computer methods and programs in biomedicine
BACKGROUND AND OBJECTIVES :
METHODS :
RESULTS :
CONCLUSIONS :
Kim Minchang, Chung Minyoung, Shin Yeong-Gil, Kim Bohyoung
2023-Mar-07
Deep learning, Dental computed tomography (CT), Metal artifacts reduction, Registration, Scanned surface model
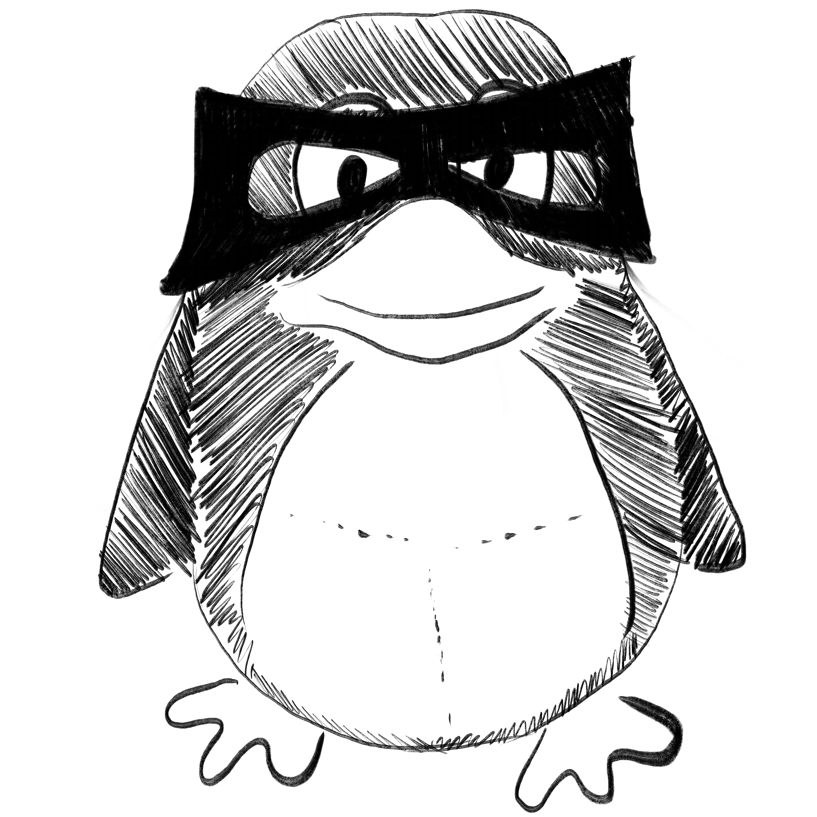
HARDC : A novel ECG-based heartbeat classification method to detect arrhythmia using hierarchical attention based dual structured RNN with dilated CNN.
In Neural networks : the official journal of the International Neural Network Society
Islam Md Shofiqul, Hasan Khondokar Fida, Sultana Sunjida, Uddin Shahadat, Lio’ Pietro, Quinn Julian M W, Moni Mohammad Ali
2023-Mar-05
Arrhythmia, BiGRU–BiLSTM, Dilated CNN, ECG, Hierarchical attention, Preprocessing
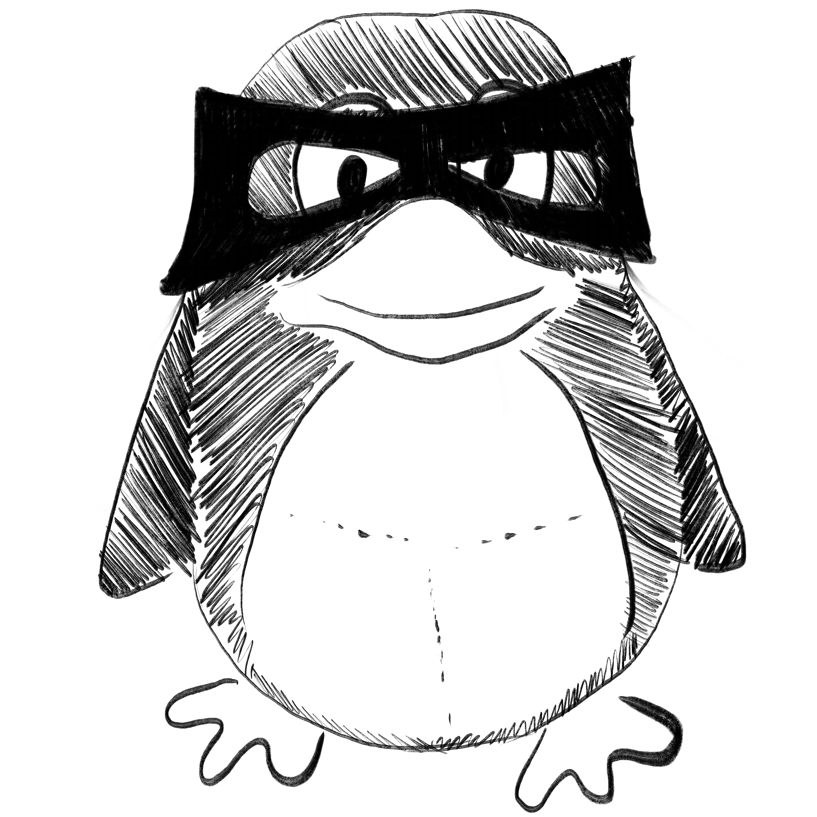
An integrated evaluation approach of wearable lower limb exoskeletons for human performance augmentation.
In Scientific reports ; h5-index 158.0
Zhang Xiao, Chen Xue, Huo Bo, Liu Chenglin, Zhu Xiaorong, Zu Yuanyuan, Wang Xiliang, Chen Xiao, Sun Qing
2023-Mar-14
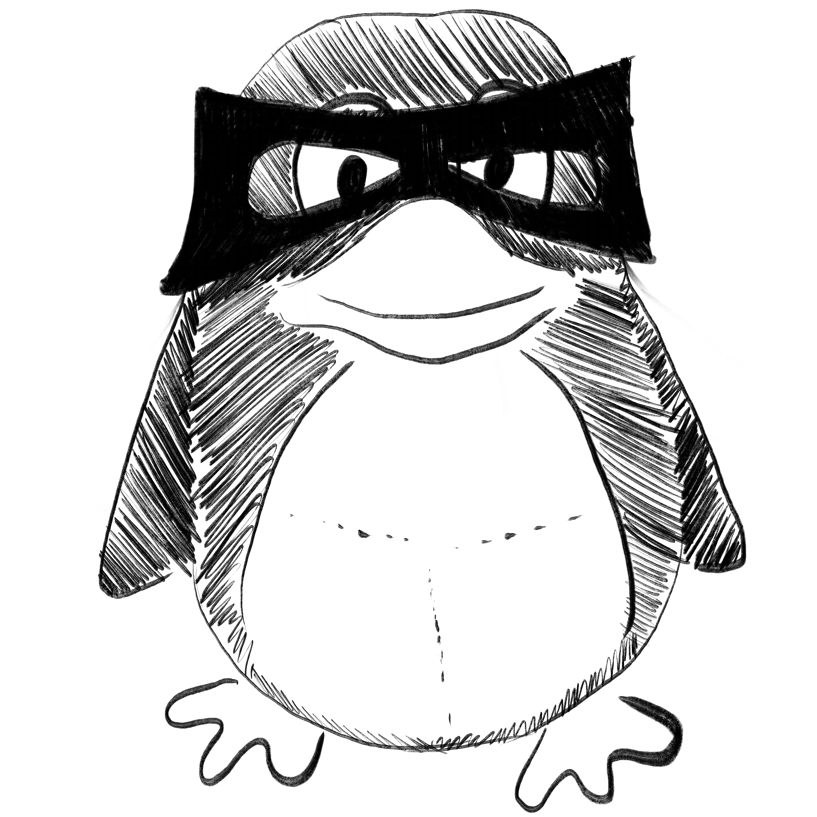
Generative Adversarial Network for Personalized Art Therapy in Melanoma Disease Management
ArXiv Preprint
Lennart Jütte, Ning Wand, Bernhard Roth
2023-03-16
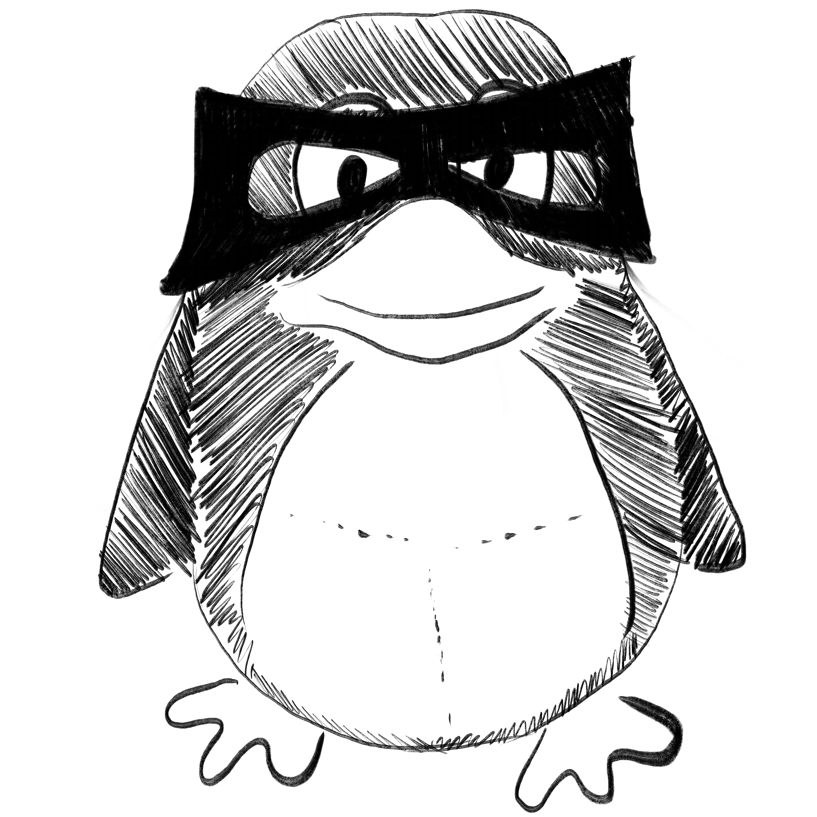
Edge computing on TPU for brain implant signal analysis.
In Neural networks : the official journal of the International Neural Network Society
Rokai János, Ulbert István, Márton Gergely
2023-Feb-28
Brain–computer interface, Deep learning, Edge device, Electrophysiology, Feature extraction, Spike sorting
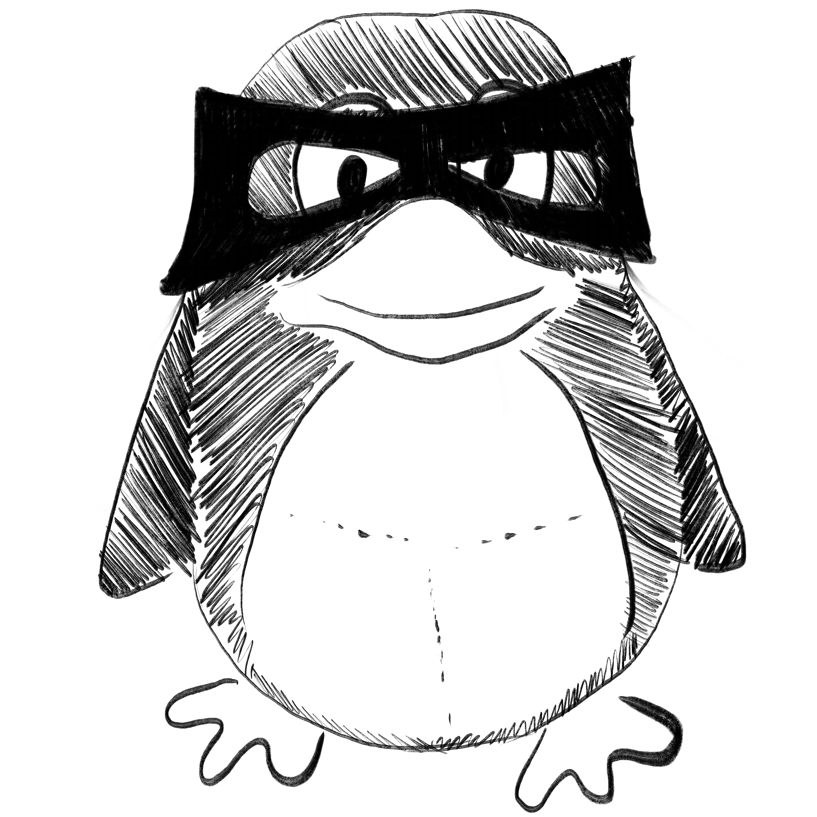
Global distribution of marine microplastics and potential for biodegradation.
In Journal of hazardous materials
Chen Bingfeng, Zhang Zhenyan, Wang Tingzhang, Hu Hang, Qin Guoyan, Lu Tao, Hong Wenjie, Hu Jun, Penuelas Josep, Qian Haifeng
2023-Mar-11
Biodegradation potential, Global microplastic distribution, Machine learning, Metagenome, Microplastic
Weekly Summary
Receive a weekly summary and discussion of the top papers of the week by leading researchers in the field.