Receive a weekly summary and discussion of the top papers of the week by leading researchers in the field.
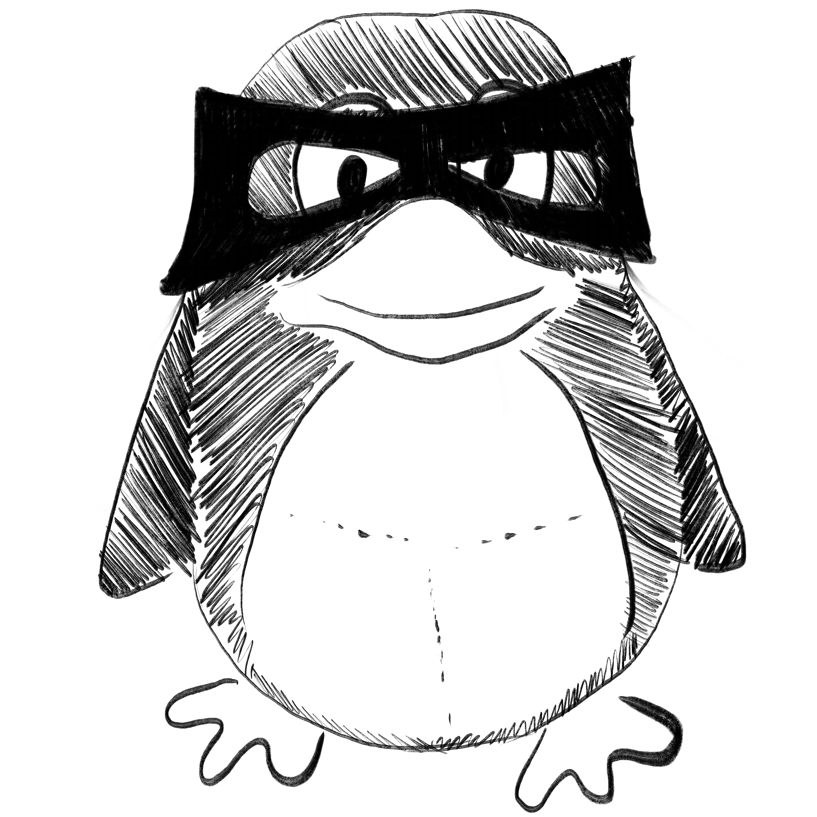
Tritium: Its relevance, sources and impacts on non-human biota.
In The Science of the total environment
Ferreira Maria F, Turner Andrew, Vernon Emily L, Grisolia Christian, Lebaron-Jacobs Laurence, Malard Veronique, Jha Awadhesh N
2023-Mar-13
Environment, Nuclear energy, Radiation dose, Risk assessment, Toxicity, Tritiated water (HTO), Tritium ((3)H)
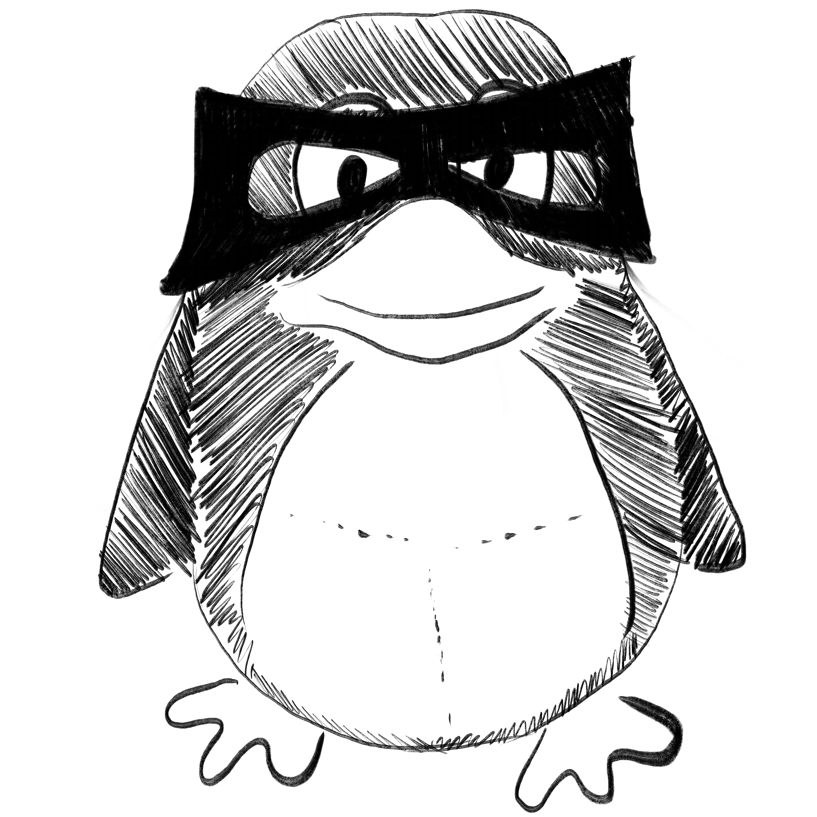
Formal autopoiesis: Solutions of the classical and extended functional closure equations.
In Bio Systems
Chastain Erick
2023-Mar-13
(M,R)-systems, Autopoiesis, Machine learning, Recursion in biology, Systems biology, Theoretical biology
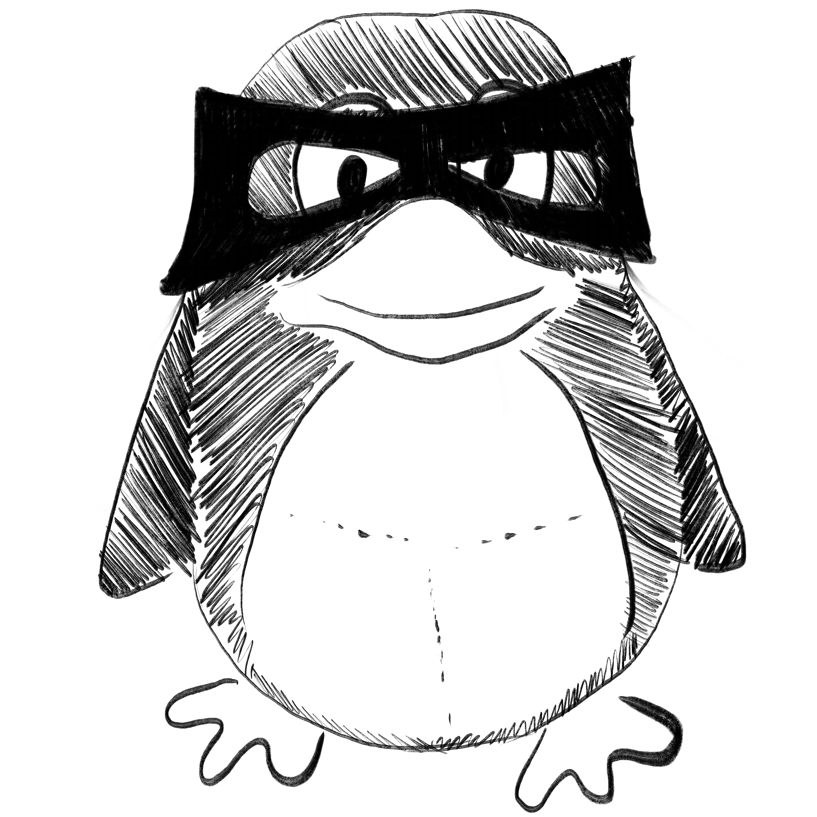
EXPLORE: a novel deep learning-based analysis method for exploration behaviour in object recognition tests.
In Scientific reports ; h5-index 158.0
IbaƱez Victor, Bohlen Laurens, Manuella Francesca, Mansuy Isabelle, Helmchen Fritjof, Wahl Anna-Sophia
2023-Mar-14
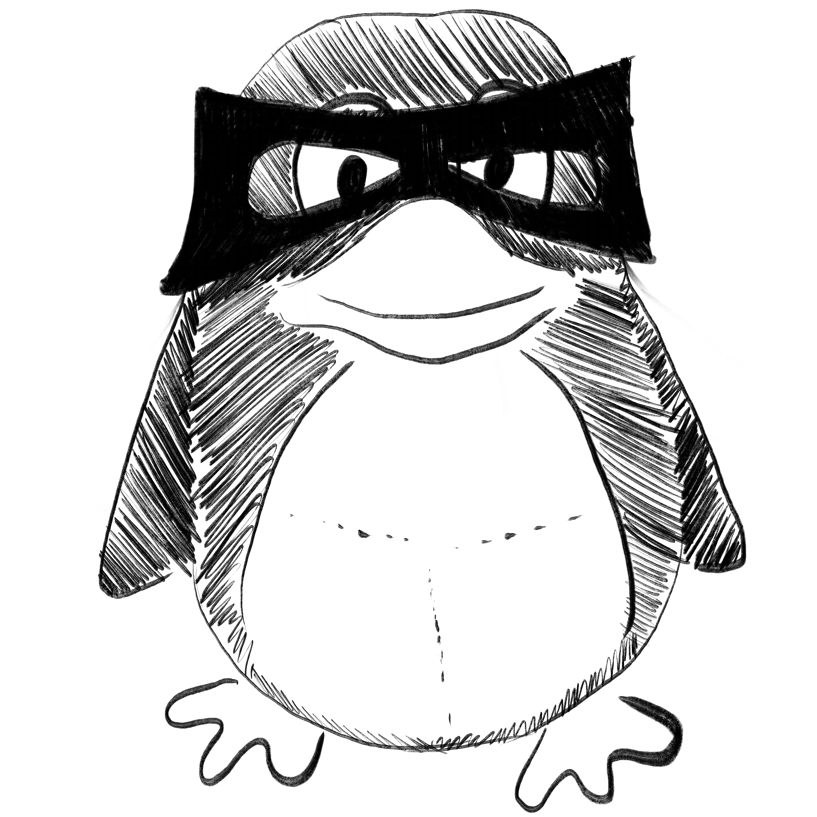
Improving Automated Hemorrhage Detection in Sparse-view Computed Tomography via Deep Convolutional Neural Network based Artifact Reduction
ArXiv Preprint
Johannes Thalhammer, Manuel Schultheiss, Tina Dorosti, Tobias Lasser, Franz Pfeiffer, Daniela Pfeiffer, Florian Schaff
2023-03-16
Prognosis prediction for glioblastoma multiforme patients using machine learning approaches: development of the clinically applicable model.
In Radiotherapy and oncology : journal of the European Society for Therapeutic Radiology and Oncology
BACKGROUND AND PURPOSE :
MATERIALS AND METHODS :
RESULTS :
CONCLUSION :
Kim Yeseul, Hwan Kim Kyung, Park Junyoung, In Yoon Hong, Sung Wonmo
2023-Mar-13
Glioblastoma multiforme, cox proportional hazards, machine learning, prognosis prediction, random survival forest, survival support vector machine, web-based prediction tool
Machine-learning-based head impact subtyping based on the spectral densities of the measurable head kinematics.
In Journal of sport and health science
BACKGROUND :
METHODS :
RESULTS :
CONCLUSION :
Zhan Xianghao, Li Yiheng, Liu Yuzhe, Cecchi Nicholas J, Raymond Samuel J, Zhou Zhou, Alizadeh Hossein Vahid, Ruan Jesse, Barbat Saeed, Tiernan Stephen, Gevaert Olivier, Zeineh Michael M, Grant Gerald A, Camarillo David B
2023-Mar-13
Classification, Contact sports, Head impacts, Impact kinematics, Traumatic brain injury
Weekly Summary
Receive a weekly summary and discussion of the top papers of the week by leading researchers in the field.