Receive a weekly summary and discussion of the top papers of the week by leading researchers in the field.
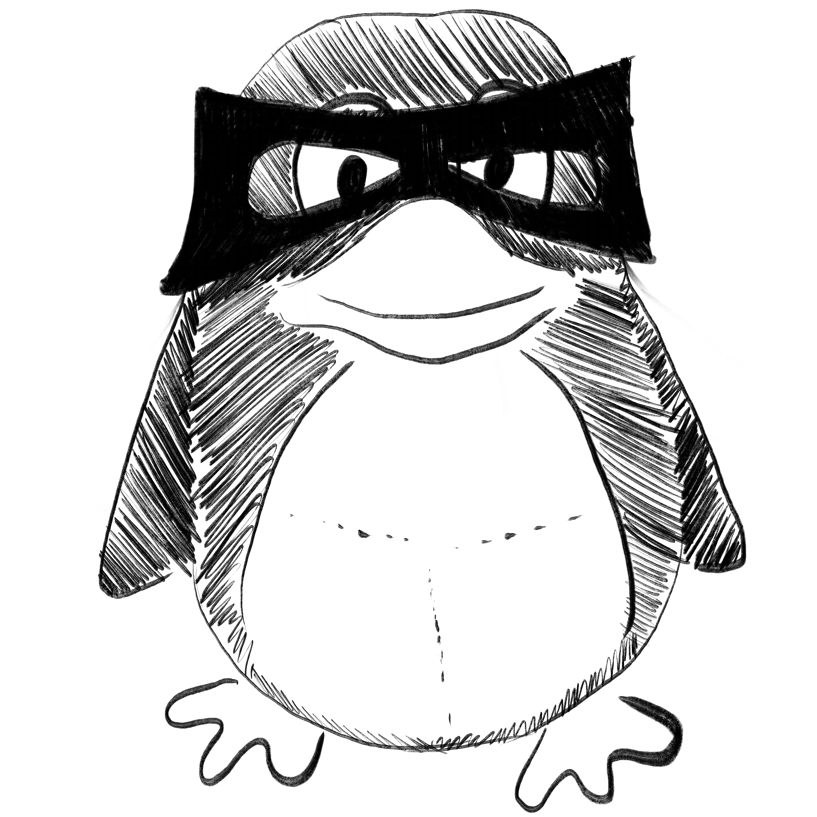
The Scope of In-Context Learning for the Extraction of Medical Temporal Constraints
ArXiv Preprint
Parker Seegmiller, Joseph Gatto, Madhusudan Basak, Diane Cook, Hassan Ghasemzadeh, John Stankovic, Sarah Preum
2023-03-16
MRI-based synthetic CT in the Detection of knee Osteoarthritis: Comparison with CT.
In Journal of orthopaedic research : official publication of the Orthopaedic Research Society
Arbabi Saeed, Foppen Wouter, Gielis Willem Paul, van Stralen Marijn, Jansen Mylène, Arbabi Vahid, de Jong Pim A, Weinans Harrie, Seevinck Peter
2023-Mar-15
CT, MRI, Neural Networks, Osteoarthritis, synthetic CT
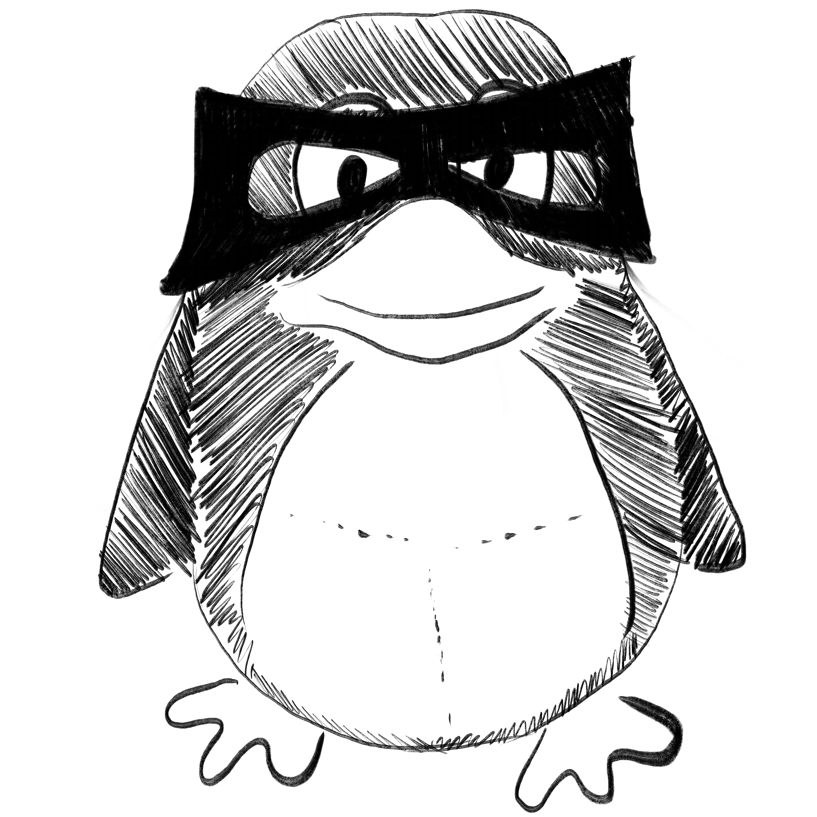
Mental wellness: The Jasper and Alice way.
In Journal of medical imaging and radiation sciences
George Visser Koch Gerhardus
2023-Mar-13
Addressing the Challenges of Implementing Artificial Intelligence Tools in Clinical Practice: Principles From Experience.
In Journal of the American College of Radiology : JACR
Bizzo Bernardo C, Dasegowda Giridhar, Bridge Christopher, Miller Benjamin, Hillis James M, Kalra Mannudeep K, Durniak Kimberly, Stout Markus, Schultz Thomas, Alkasab Tarik, Dreyer Keith J
2023-Mar
Artificial intelligence, deployment, implementation, machine learning, radiology
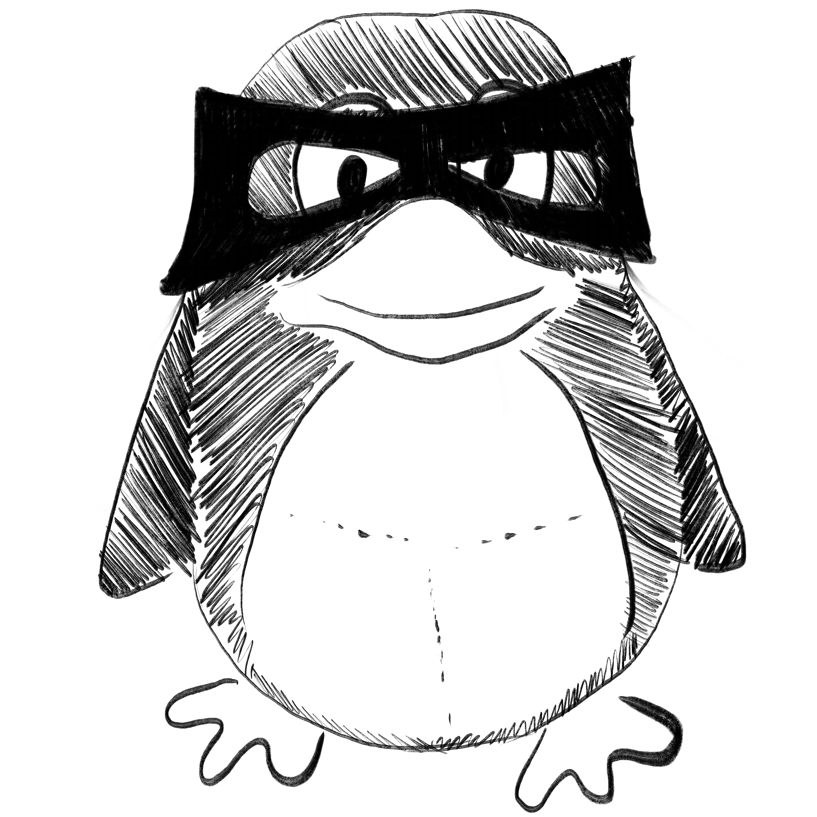
The forces generated by agonist muscles during isometric contractions arise from motor unit synergies.
In The Journal of neuroscience : the official journal of the Society for Neuroscience
Del Vecchio Alessandro, Marconi Germer Carina, Kinfe Thomas Mehari, Nuccio Stefano, Hug François, Eskofier Bjoern, Farina Dario, Enoka Roger Maro
2023-Mar-15
Outcomes of ST elevation myocardial infarction in patients with cancer; a nationwide study.
In European heart journal. Quality of care & clinical outcomes
AIMS :
METHODS :
RESULTS :
CONCLUSION :
Dafaalla Mohamed, Abdel-Qadir Husam, Gale Chris P, Sun Louise, López-Fernández Teresa, Miller Robert J H, Wojakowski Wojtek, Nolan James, Rashid Muhammad, Mamas Mamas A
2023-Mar-15
Weekly Summary
Receive a weekly summary and discussion of the top papers of the week by leading researchers in the field.