Receive a weekly summary and discussion of the top papers of the week by leading researchers in the field.
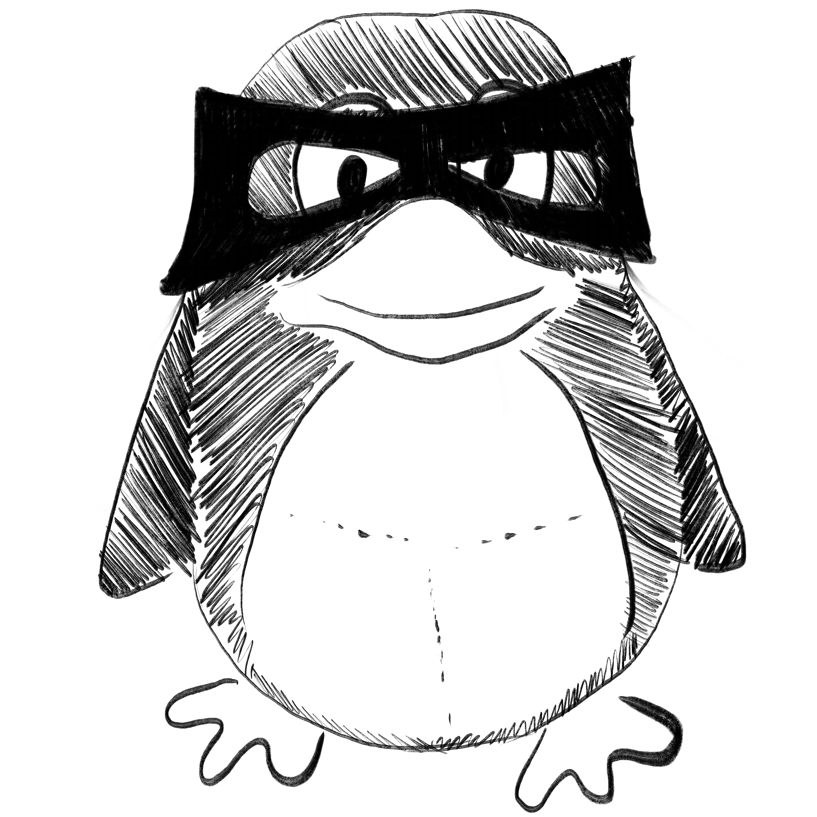
Thyroid autoimmunity and adverse pregnancy outcomes: A multiple center retrospective study.
In Frontiers in endocrinology ; h5-index 55.0
BACKGROUND :
OBJECTIVE :
METHODS :
RESULTS :
CONCLUSION :
Xu Yun, Chen Hui, Ren Meng, Gao Yu, Sun Kan, Wu Hongshi, Ding Rui, Wang Junhui, Li Zheqing, Liu Dan, Wang Zilian, Yan Li
2023
birth weight, dose dependent effect, gestational diabetes mellitus, maternal and fetal outcomes, pregnancy-induced hypertension, thyroid autoimmunity
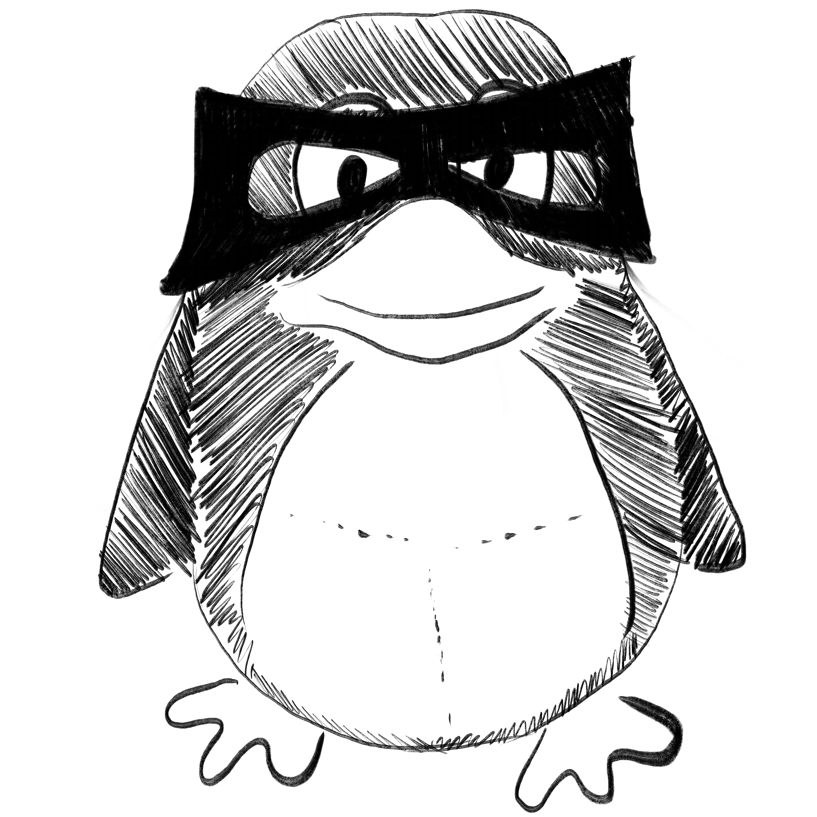
Deep learning-based EEG emotion recognition: Current trends and future perspectives.
In Frontiers in psychology ; h5-index 92.0
Wang Xiaohu, Ren Yongmei, Luo Ze, He Wei, Hong Jun, Huang Yinzhen
2023
deep learning, electroencephalogram, emotion recognition, human–computer interaction, survey
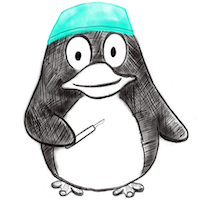
Identification of ferroptosis-related genes in ulcerative colitis: a diagnostic model with machine learning.
In Annals of translational medicine
BACKGROUND :
METHODS :
RESULTS :
CONCLUSIONS :
Qian Rui, Tang Min, Ouyang Zichen, Cheng Honghui, Xing Sizhong
2023-Feb-28
Ulcerative colitis, bioinformatic analysis, diagnostic model, ferroptosis
CCTCOVID: COVID-19 detection from chest X-ray images using Compact Convolutional Transformers.
In Frontiers in public health
Marefat Abdolreza, Marefat Mahdieh, Hassannataj Joloudari Javad, Nematollahi Mohammad Ali, Lashgari Reza
2023
COVID-19, Compact Convolutional Transformers, Convolutional Neural Networks, deep learning, vision transformers
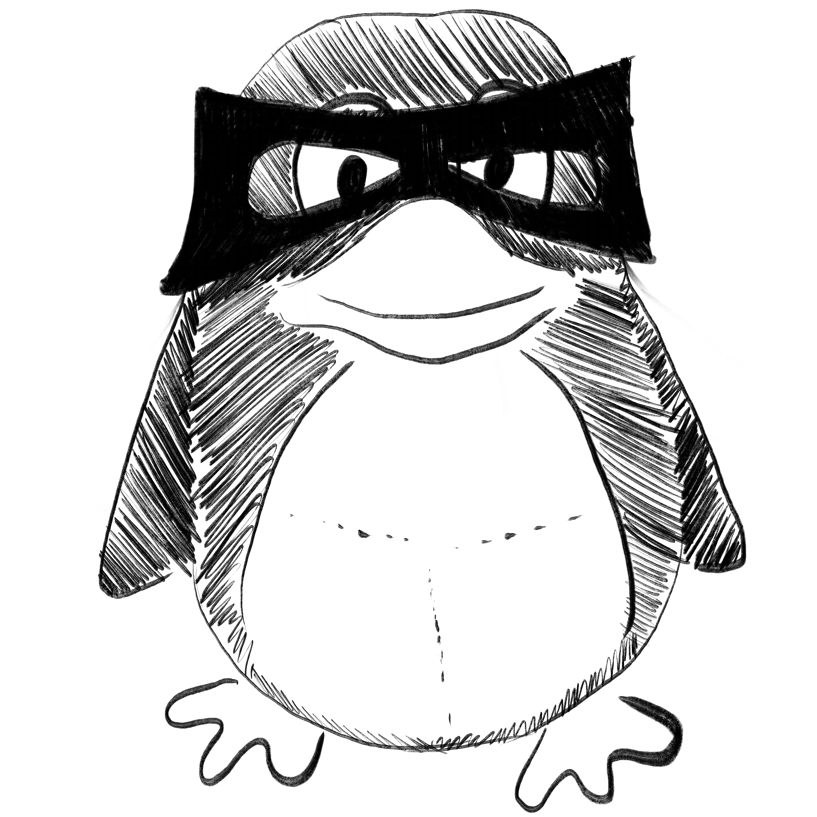
promor: a comprehensive R package for label-free proteomics data analysis and predictive modeling.
In Bioinformatics advances
SUMMARY :
AVAILABILITY AND IMPLEMENTATION :
SUPPLEMENTARY INFORMATION :
Ranathunge Chathurani, Patel Sagar S, Pinky Lubna, Correll Vanessa L, Chen Shimin, Semmes O John, Armstrong Robert K, Combs C Donald, Nyalwidhe Julius O
2023
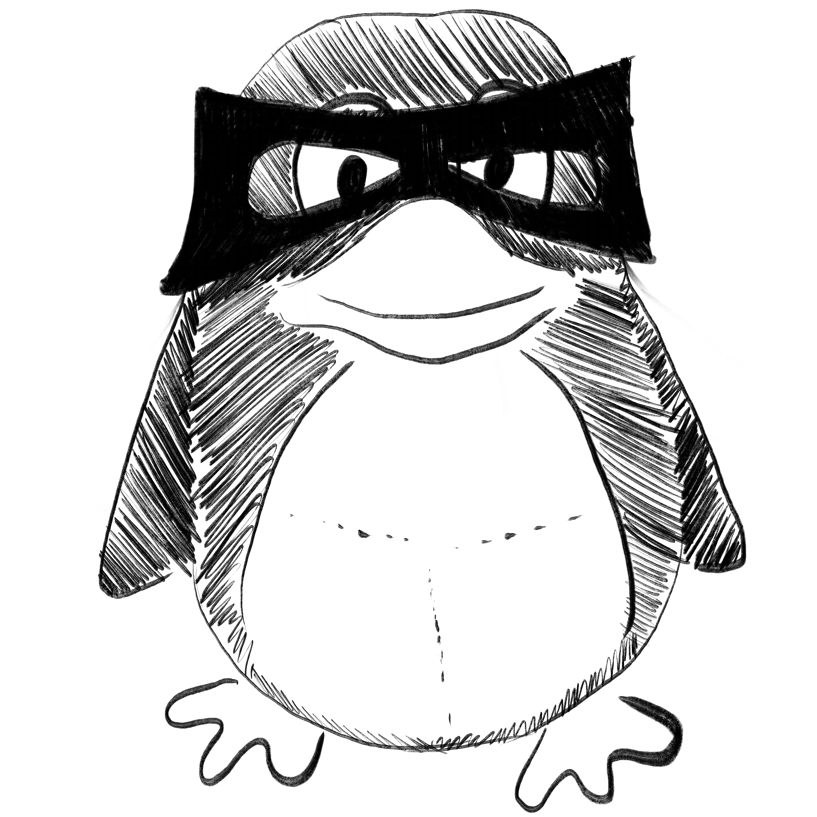
Machine learning-based clustering to identify the combined effect of the DNA fragmentation index and conventional semen parameters on in vitro fertilization outcomes.
In Reproductive biology and endocrinology : RB&E
BACKGROUND :
METHODS :
RESULTS :
CONCLUSIONS :
Peng Tianwen, Liao Chen, Ye Xin, Chen Zhicong, Li Xiaomin, Lan Yu, Fu Xin, An Geng
2023-Mar-15
Coexposure, IVF outcomes, K-means clustering, Routine semen parameters, Sperm DNA fragmentation index
Weekly Summary
Receive a weekly summary and discussion of the top papers of the week by leading researchers in the field.