Receive a weekly summary and discussion of the top papers of the week by leading researchers in the field.
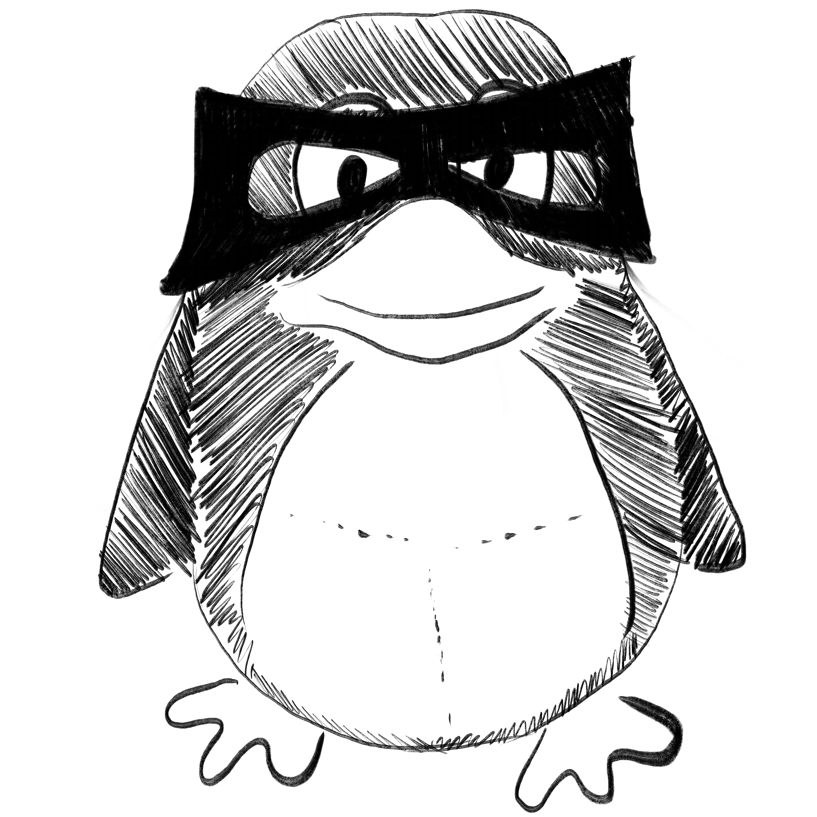
Artificial intelligence evaluation of COVID-19 restrictions and speech therapy effects on the autistic children's behavior.
In Scientific reports ; h5-index 158.0
Sabzevari Fereshteh, Amelirad Omid, Moradi Zohre, Habibi Mostafa
2023-Mar-15
Breast Cancer Histopathology Image based Gene Expression Prediction using Spatial Transcriptomics data and Deep Learning
ArXiv Preprint
Md Mamunur Rahaman, Ewan K. A. Millar, Erik Meijering
2023-03-17
Artificial intelligence-based computer-aided diagnosis system supports diagnosis of lymph node metastasis in esophageal squamous cell carcinoma: A multicenter study.
In Heliyon
BACKGROUND :
METHODS :
RESULTS :
CONCLUSIONS :
Zhang Shuai-Tong, Wang Si-Yun, Zhang Jie, Dong Di, Mu Wei, Xia Xue-Er, Fu Fang-Fang, Lu Ya-Nan, Wang Shuo, Tang Zhen-Chao, Li Peng, Qu Jin-Rong, Wang Mei-Yun, Tian Jie, Liu Jian-Hua
2023-Mar
18F-FDG PET/CT, 18-fluorine-fluorodeoxyglucose positron-emission tomography/computed tomography, AI, Artificial intelligence, AI-CAD, Artificial intelligence-based computer-aided diagnosis, Artificial intelligence, CI, Confidence interval, CT, Computed tomography, ESCC, Esophageal squamous cell carcinoma, Esophageal squamous cell carcinoma, LNM, Lymph node metastasis, Lymph node metastasis, OS, Overall survival, PET/CT, PFS, Progression-free survival, SD, Standard deviation, SLR, Ratio of the SUV value to liver uptake, SUV, Standardized uptake value, cN, Clinical N stage, nCRT, Neoadjuvant chemoradiotherapy, pN, Pathological N stage
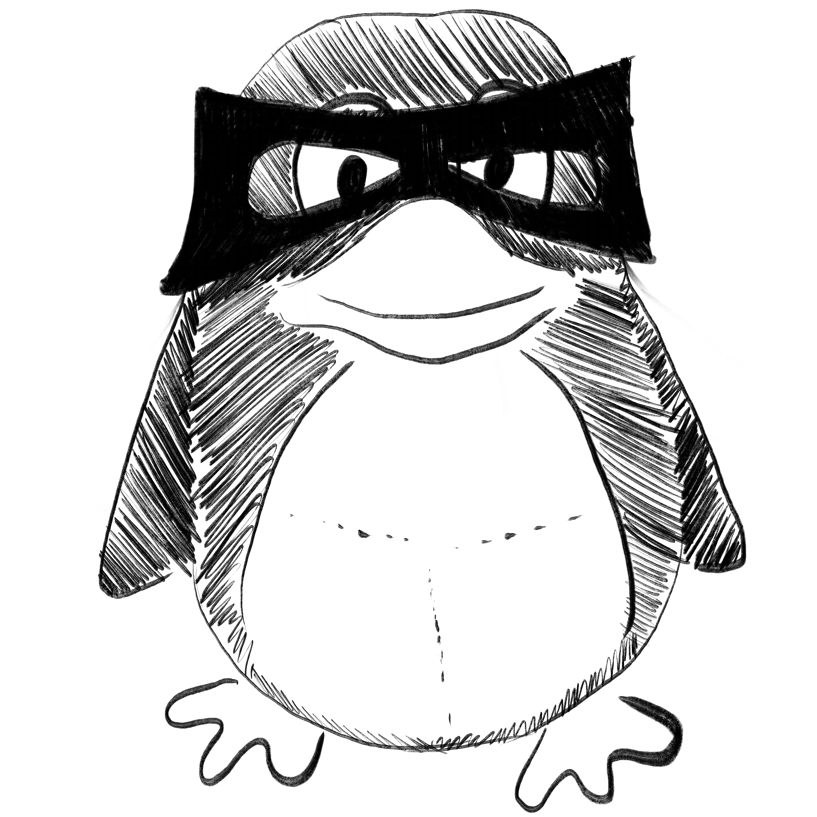
Identification and validation of metabolism-related hub genes in idiopathic pulmonary fibrosis.
In Frontiers in genetics ; h5-index 62.0
Zeng Youjie, Huang Jun, Guo Ren, Cao Si, Yang Heng, Ouyang Wen
2023
IPF, bioinformatics, biomarker, differentially expressed genes, gene expression omnibus, hub genes, metabolic, metabolism
Modeling contrast-to-noise ratio from list mode reconstructions of 68Ga DOTATATE PET/CT: predicting detectability of hepatic metastases in shorter acquisition PET reconstructions.
In American journal of nuclear medicine and molecular imaging
BACKGROUND :
METHODS :
RESULTS :
CONCLUSIONS :
Silosky Michael, Xing Fuyong, Wehrend John, Litwiller Daniel V, Metzler Scott D, Chin Bennett B
2023
68Ga DOTATATE, PET/CT, artificial intelligence, contrast-to-noise, detectability
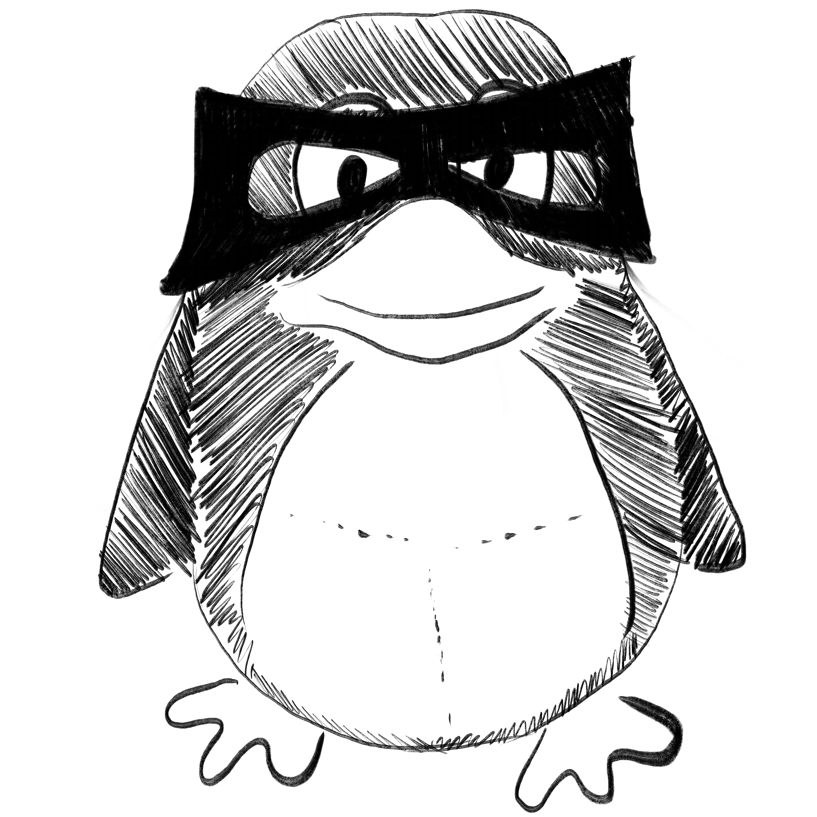
Human circulating small non-coding RNA signature as a non-invasive biomarker in clinical diagnosis of acute myeloid leukaemia.
In Theranostics
Xia Lin, Guo Huanping, Wu Xiao, Xu Yinying, Zhao Pan, Yan Bingbing, Zeng Yunjing, He Yundi, Chen Dan, Gale Robert Peter, Zhang Yunfang, Zhang Xi
2023
alternative noninvasive biomarker, human circulating sncRNA, rsRNA, tsRNA, ysRNA
Weekly Summary
Receive a weekly summary and discussion of the top papers of the week by leading researchers in the field.