Receive a weekly summary and discussion of the top papers of the week by leading researchers in the field.
COVID-19Base v3: Update of the knowledgebase for drugs and biomedical entities linked to COVID-19.
In Frontiers in public health
Basit Syed Abdullah, Qureshi Rizwan, Musleh Saleh, Guler Reto, Rahman M Sohel, Biswas Kabir H, Alam Tanvir
2023
CORD-19, COVID-19, SARS-CoV-2, deep learning, machine learning
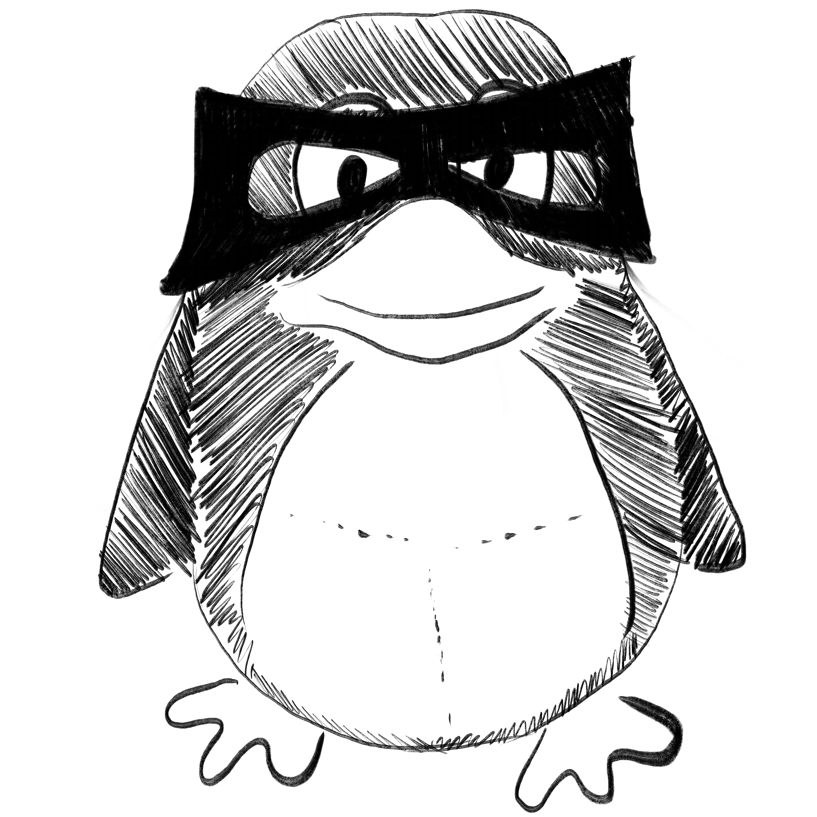
Smart Yoga Instructor for Guiding and Correcting Yoga Postures in Real Time.
In International journal of yoga
Kishore D Mohan, Bindu S, Manjunath Nandi Krishnamurthy
2022
Human posture recognition, Mediapipe, Yoga, pose detection and pose correction, real-time
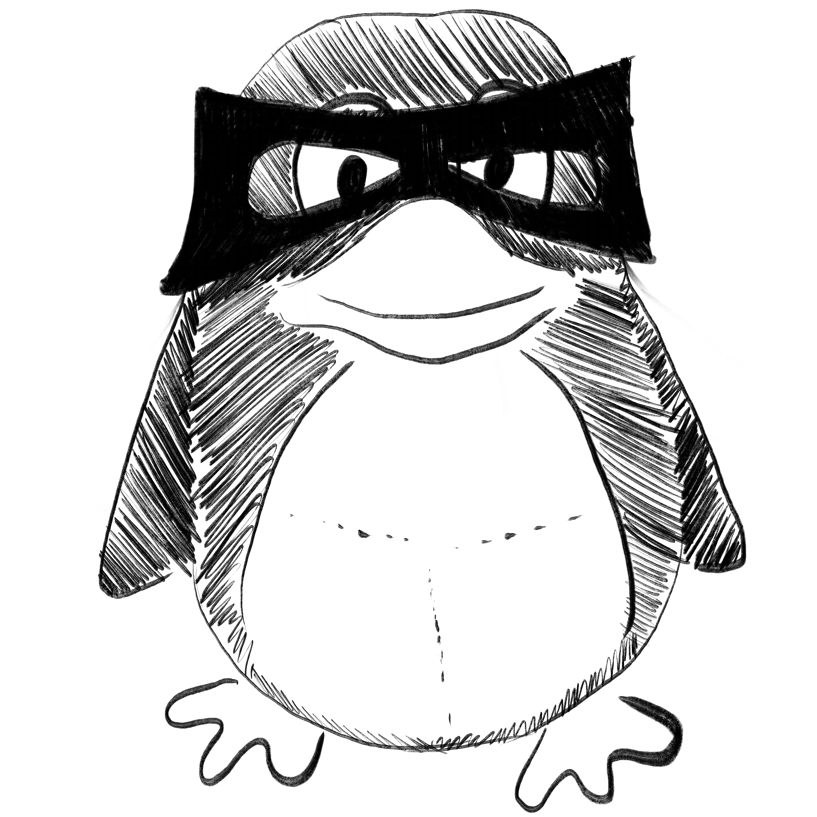
scAnnotate: an automated cell-type annotation tool for single-cell RNA-sequencing data.
In Bioinformatics advances
MOTIVATION :
RESULTS :
AVAILABILITY AND IMPLEMENTATION :
SUPPLEMENTARY INFORMATION :
Ji Xiangling, Tsao Danielle, Bai Kailun, Tsao Min, Xing Li, Zhang Xuekui
2023
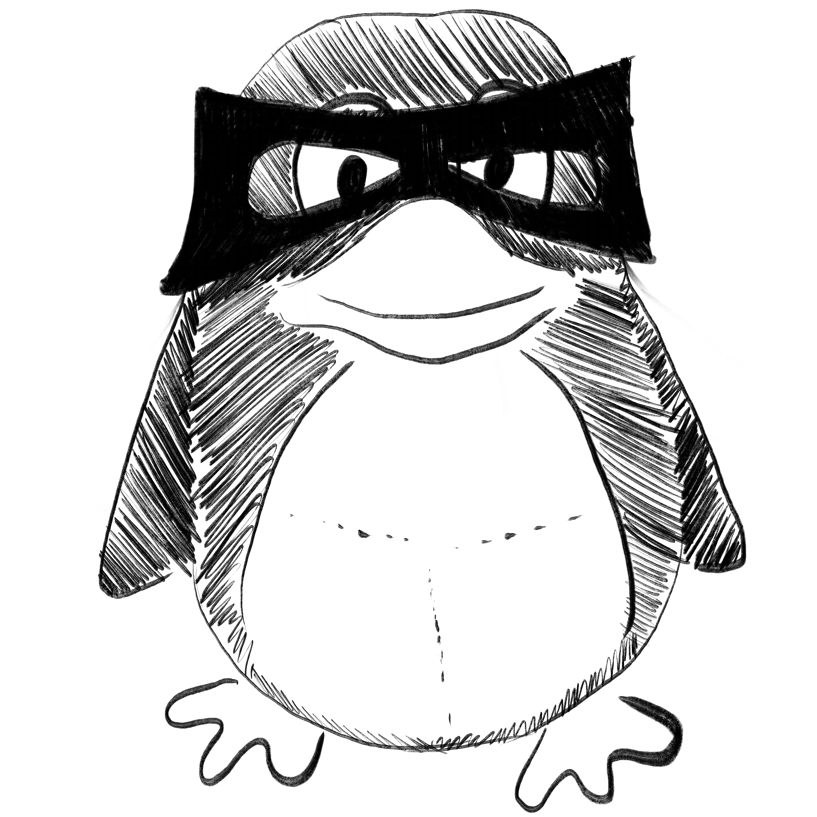
Multiplexed DNA-functionalized graphene sensor with artificial intelligence-based discrimination performance for analyzing chemical vapor compositions.
In Microsystems & nanoengineering
Hwang Yun Ji, Yu Heejin, Lee Gilho, Shackery Iman, Seong Jin, Jung Youngmo, Sung Seung-Hyun, Choi Jongeun, Jun Seong Chan
2023
Computational nanotechnology, Electronic properties and materials, Nanosensors
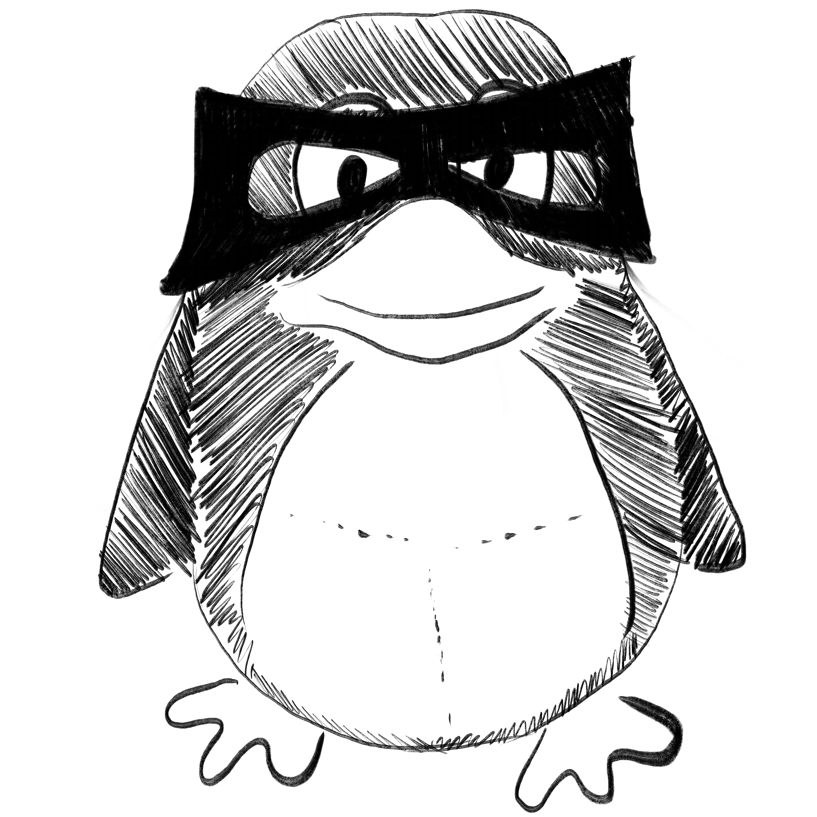
Astrocyte structural heterogeneity in the mouse hippocampus.
In Glia
Viana João Filipe, Machado João Luís, Abreu Daniela Sofia, Veiga Alexandra, Barsanti Sara, Tavares Gabriela, Martins Manuella, Sardinha Vanessa Morais, Guerra-Gomes Sónia, Domingos Cátia, Pauletti Alberto, Wahis Jérôme, Liu Chen, Calì Corrado, Henneberger Christian, Holt Matthew G, Oliveira João Filipe
2023-Mar-22
astrocyte, dorsal, hippocampus, morphology, skeleton, ventral
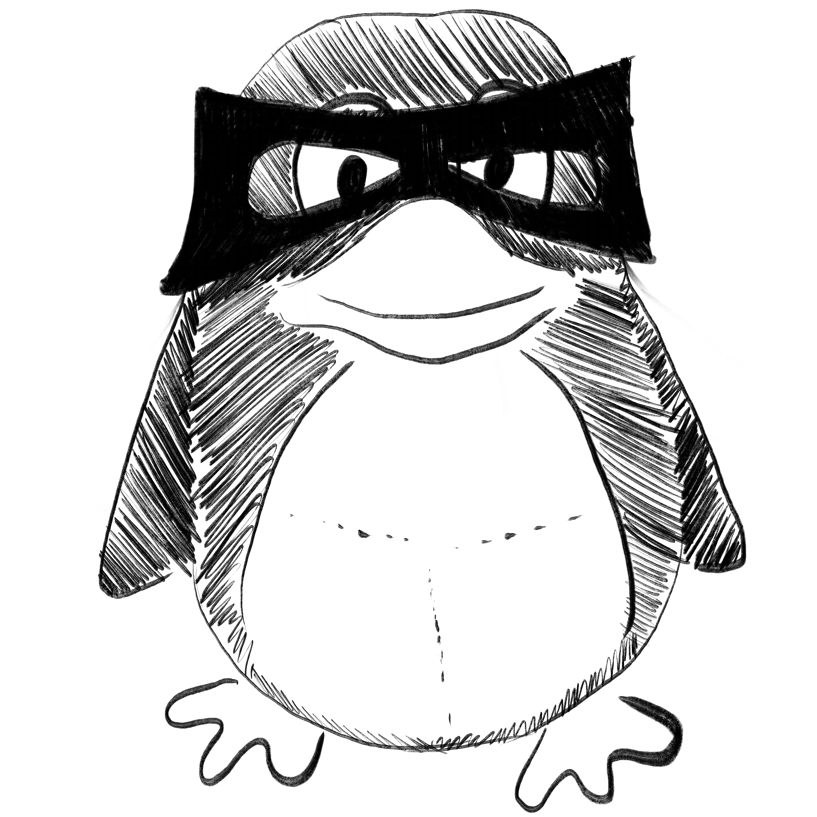
Artificial intelligence's interpretation of the neuroanatomical aspect of Peter Paul Rubens's copy of "The Battle of Anghiari" by Leonardo da Vinci.
In Perception
Keshelava Grigol
2023-Mar-22
Leonardo da Vinci, Renaissance, The Battle of Anghiari, artificial intelligence, brain anatomy
Weekly Summary
Receive a weekly summary and discussion of the top papers of the week by leading researchers in the field.