Receive a weekly summary and discussion of the top papers of the week by leading researchers in the field.
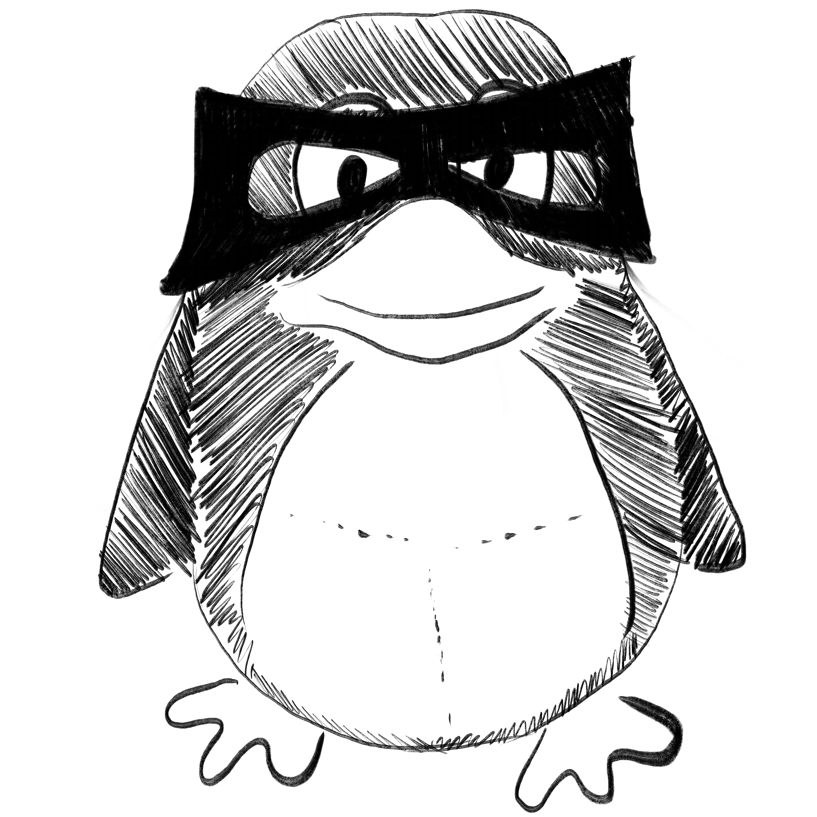
The nonalcoholic fatty liver risk in prediction of unfavorable outcome after stroke: A nationwide registry analysis.
In Computers in biology and medicine
Chen Keyang, Pan Yuesong, Xiang Xianglong, Meng Xia, Yao Dongxiao, Lin Li, Li Xiaokun, Wang Yongjun
2023-Feb-28
Data mining, Medical diagnosis, Non-alcoholic fatty liver disease, Stroke functional outcome, Stroke prognosis
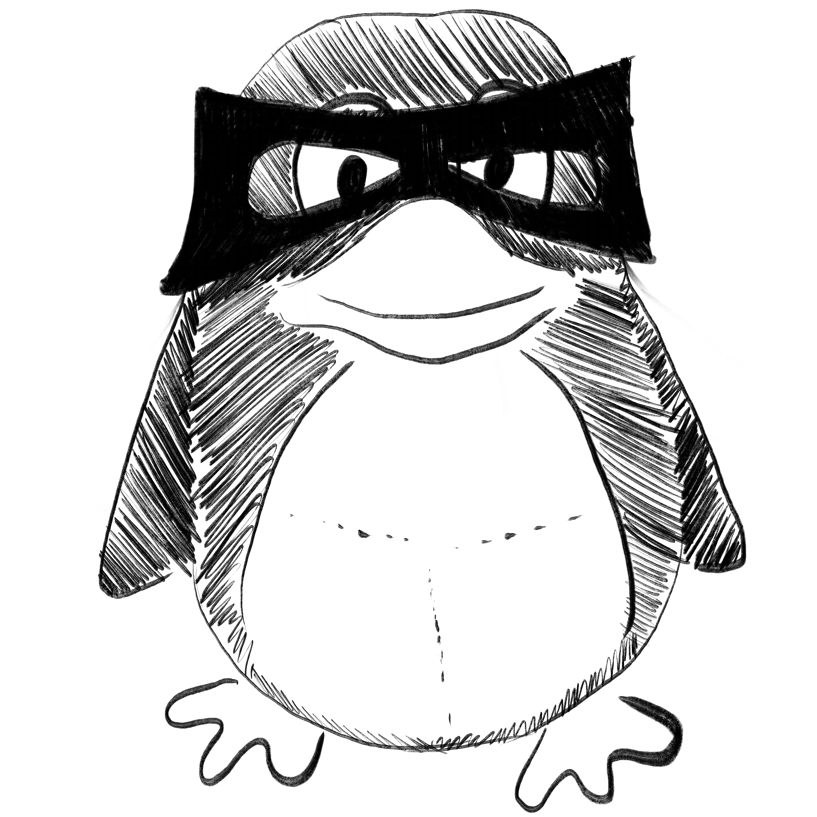
A review of deep learning-based multiple-lesion recognition from medical images: classification, detection and segmentation.
In Computers in biology and medicine
Jiang Huiyan, Diao Zhaoshuo, Shi Tianyu, Zhou Yang, Wang Feiyu, Hu Wenrui, Zhu Xiaolin, Luo Shijie, Tong Guoyu, Yao Yu-Dong
2023-Mar-01
Classification, Deep learning, Detection, Medical image, Segmentation
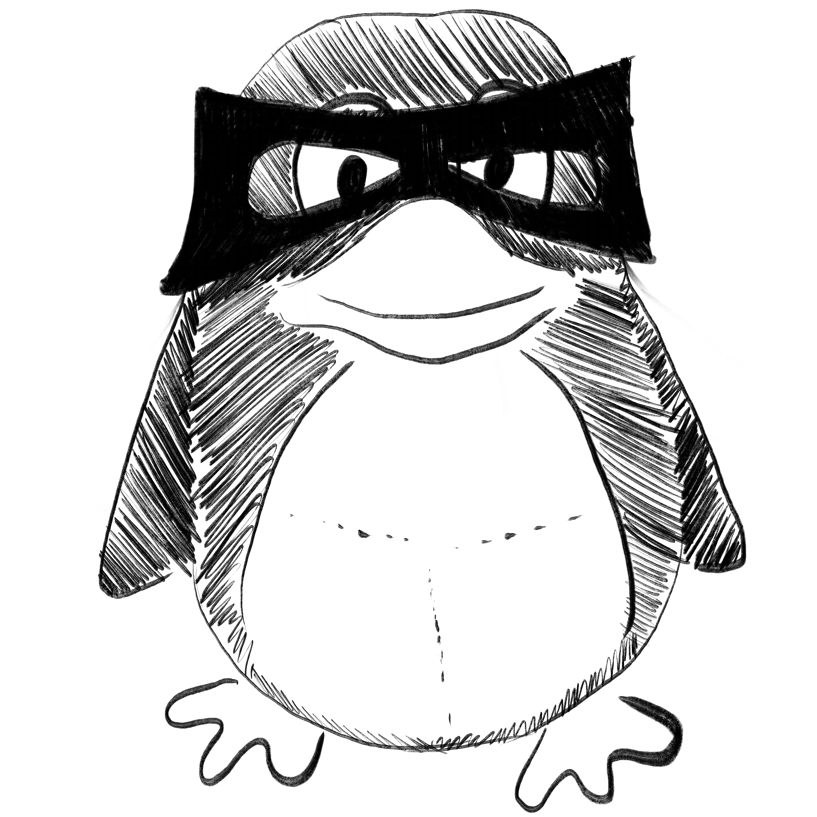
Identification of small open reading frames in plant lncRNA using class-imbalance learning.
In Computers in biology and medicine
Zhao Siyuan, Meng Jun, Wekesa Jael Sanyanda, Luan Yushi
2023-Mar-11
Class-imbalance learning, Ensemble learning, Feature selection, Hybrid resampling, lncRNA, sORFs
Machine-learning analysis of opioid use disorder informed by MOR, DOR, KOR, NOR and ZOR-based interactome networks.
In Computers in biology and medicine
Feng Hongsong, Elladki Rana, Jiang Jian, Wei Guo-Wei
2023-Mar-08
Cross-prediction, Machine-learning, Opioid receptor, Opioid use disorder, Repurposing, Side effect
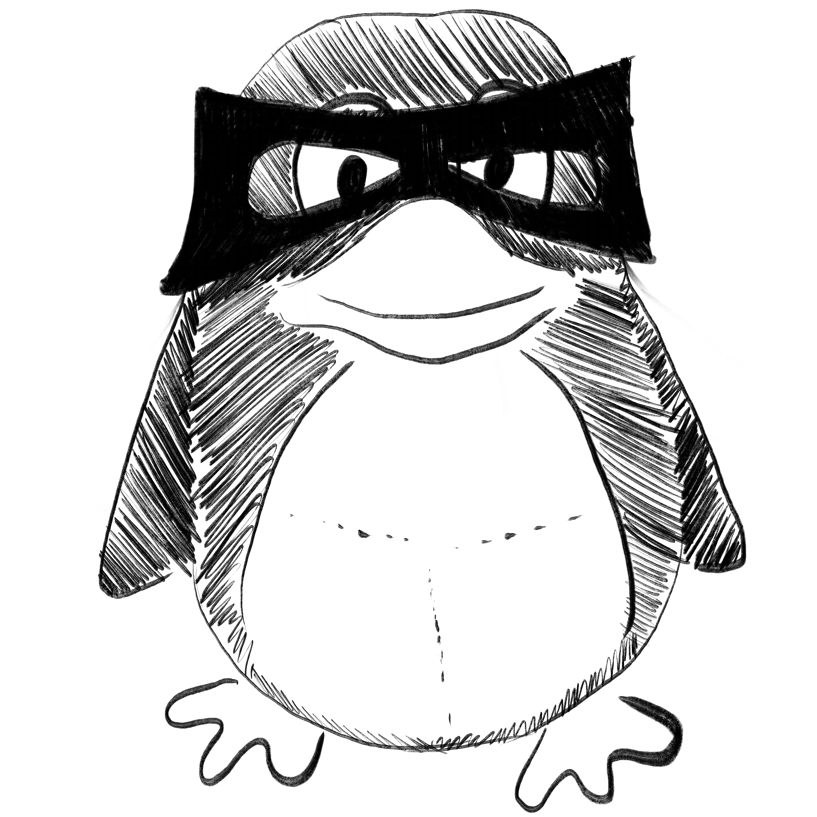
Attention is not all you need: the complicated case of ethically using large language models in healthcare and medicine.
In EBioMedicine
Harrer Stefan
2023-Mar-14
AI ethics, AI trustworthiness, Augmented human intelligence, Foundation models, Generative artificial intelligence, Information management, Large language models
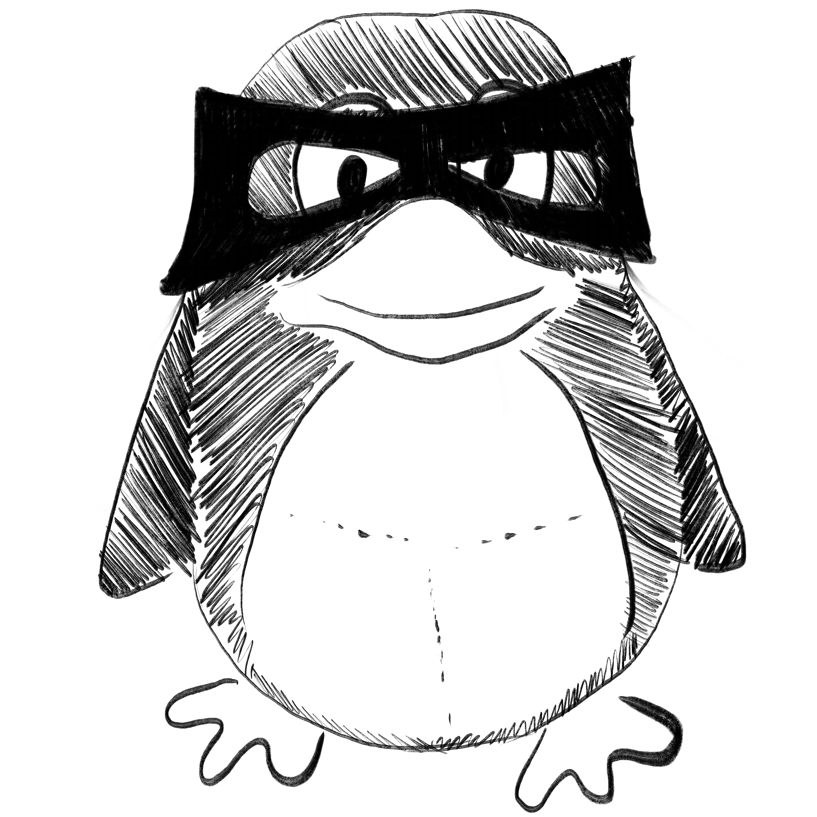
BCM-DTI: A fragment-oriented method for drug-target interaction prediction using deep learning.
In Computational biology and chemistry
Dou Liang, Zhang Zhen, Liu Dan, Qian Ying, Zhang Qian
2023-Mar-05
Deep learning, Drug–target interaction, Fragment
Weekly Summary
Receive a weekly summary and discussion of the top papers of the week by leading researchers in the field.