Receive a weekly summary and discussion of the top papers of the week by leading researchers in the field.
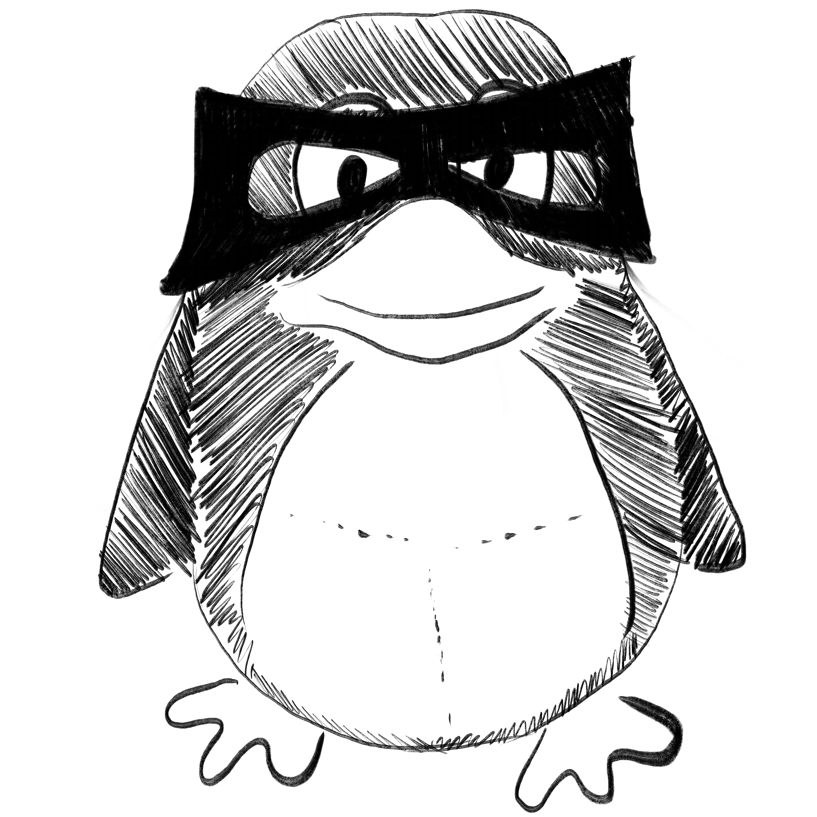
Identifying populations at ultra-high risk of suicide using a novel machine learning method.
In Comprehensive psychiatry
BACKGROUND :
METHODS :
FINDINGS :
INTERPRETATION :
Berkelmans Guus, Schweren Lizanne, Bhulai Sandjai, van der Mei Rob, Gilissen Renske
2023-Mar-01
Interactions, Machine learning, Population data, Risk factors, Suicide
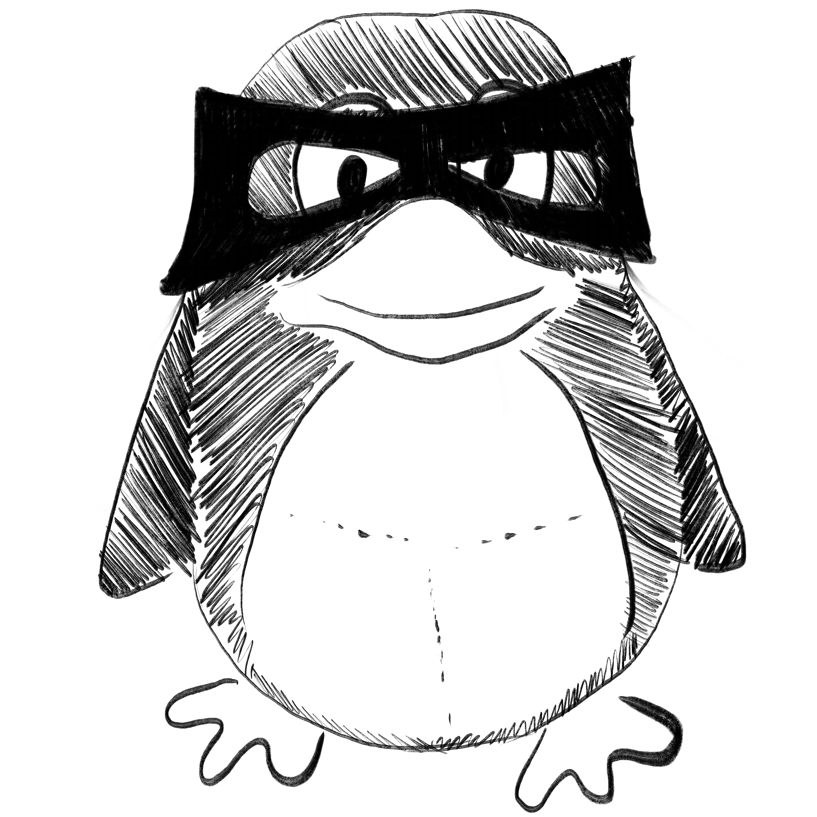
Reduced corticolimbic habituation to negative stimuli characterizes bipolar depressed suicide attempters.
In Psychiatry research. Neuroimaging
Vai Benedetta, Calesella Federico, Lenti Claudia, Fortaner-Uyà Lidia, Caselani Elisa, Fiore Paola, Breit Sigrid, Poletti Sara, Colombo Cristina, Zanardi Raffaella, Benedetti Francesco
2023-Mar-11
Bipolar disorder, Depression, Habituation, Machine learning, Suicide
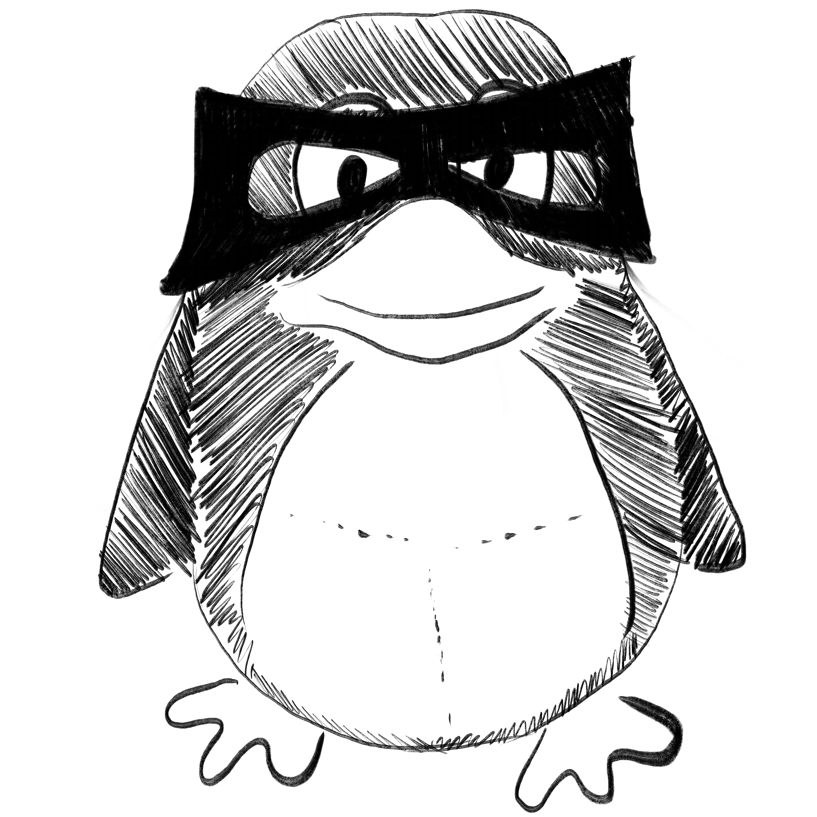
Constructing discriminative feature space for LncRNA-protein interaction based on deep autoencoder and marginal fisher analysis.
In Computers in biology and medicine
Teng Zhixia, Zhang Yiran, Dai Qiguo, Wu Chengyan, Li Dan
2023-Feb-28
Autoencoder, Feature extraction, LncRNA, Marginal fisher analysis, Protein
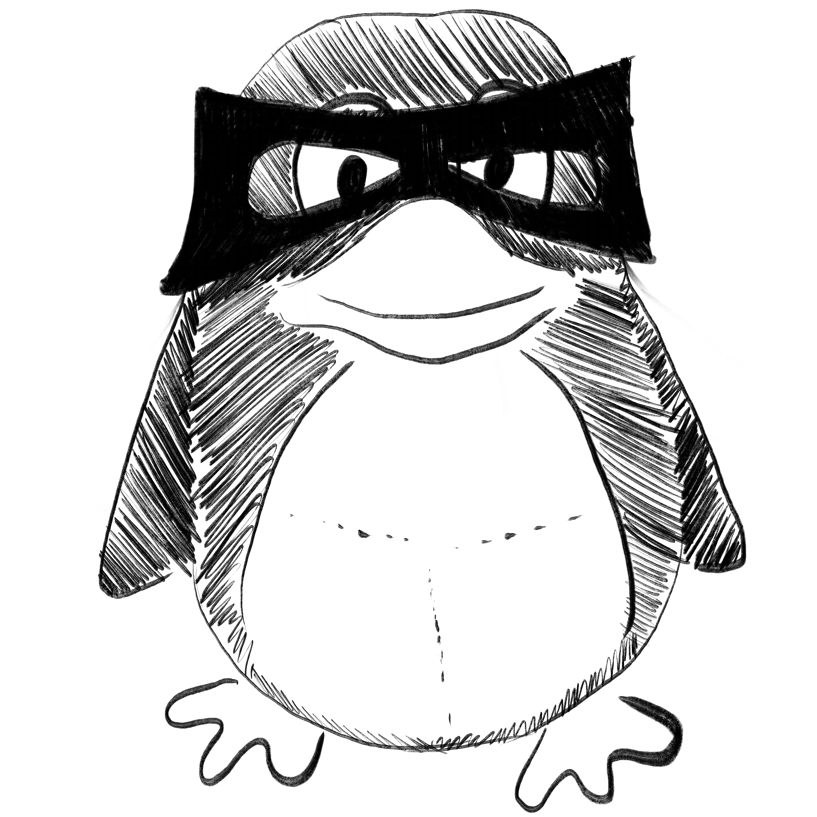
Newly reconstructed Arctic surface air temperatures for 1979-2021 with deep learning method.
In Scientific data
Ma Ziqi, Huang Jianbin, Zhang Xiangdong, Luo Yong, Ding Minghu, Wen Jun, Jin Weixin, Qiao Chen, Yin Yifu
2023-Mar-15
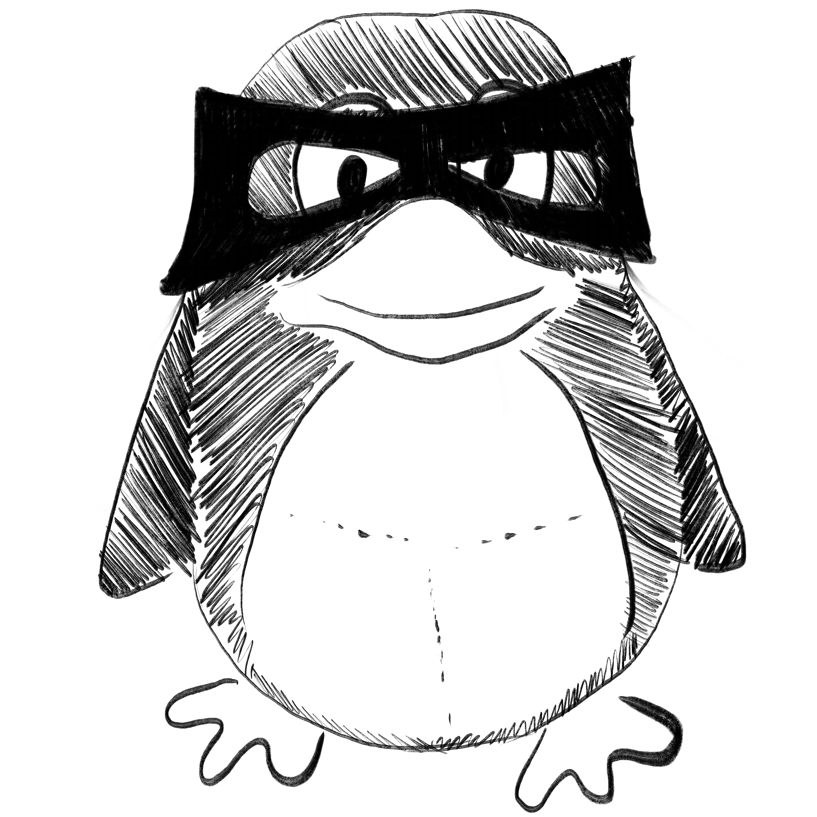
Multi-weight susceptible-infected model for predicting COVID-19 in China.
In Neurocomputing
Zhang Jun, Zheng Nanning, Liu Mingyu, Yao Dingyi, Wang Yusong, Wang Jianji, Xin Jingmin
2023-May-14
COVID-19 prediction, Data processing, Epidemic model, Multi-weight susceptible-infected model
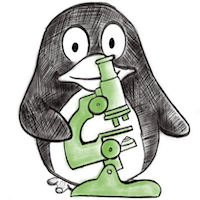
GNNFormer: A Graph-based Framework for Cytopathology Report Generation
ArXiv Preprint
Yang-Fan Zhou, Kai-Lang Yao, Wu-Jun Li
2023-03-17
Weekly Summary
Receive a weekly summary and discussion of the top papers of the week by leading researchers in the field.