Receive a weekly summary and discussion of the top papers of the week by leading researchers in the field.
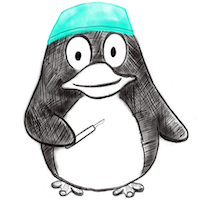
Derivation and Validation of a Machine Learning Algorithm for Predicting Venous Thromboembolism in Injured Children.
In Journal of pediatric surgery ; h5-index 38.0
BACKGROUND :
METHODS :
RESULTS :
HIGH-RISK PREDICTION :
CONCLUSION :
LEVEL OF EVIDENCE :
TYPE OF STUDY :
Papillon Stephanie C, Pennell Christopher P, Master Sahal A, Turner Evan M, Arthur L Grier, Grewal Harsh, Aronoff Stephen C
2023-Feb-18
Machine learning, Pediatric trauma, TQIP, Venous thromboembolism
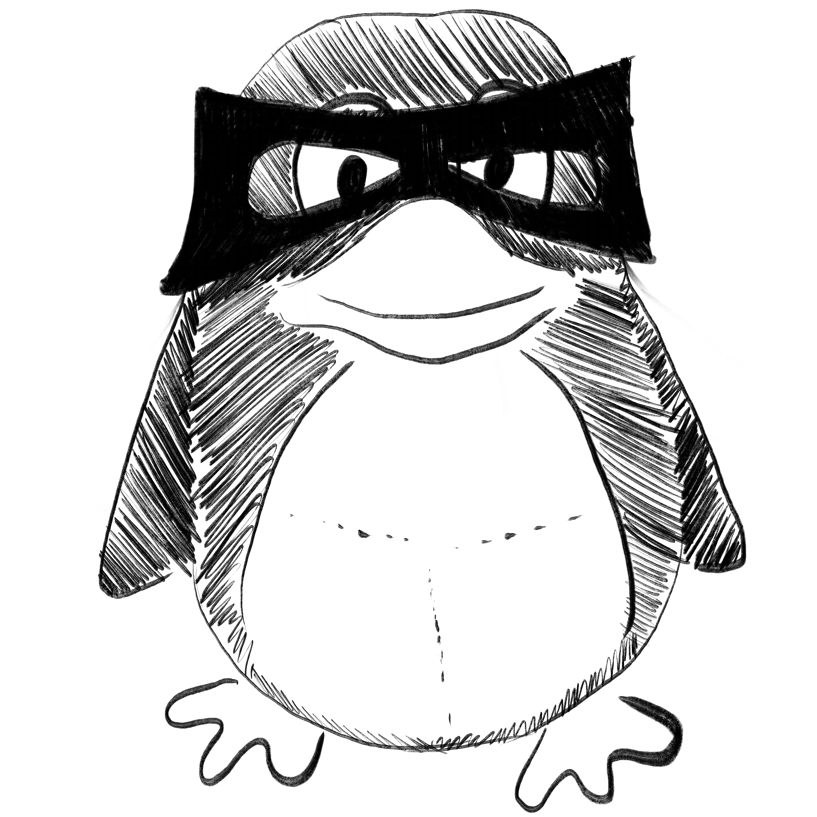
Artificial intelligence evaluation of COVID-19 restrictions and speech therapy effects on the autistic children's behavior.
In Scientific reports ; h5-index 158.0
Sabzevari Fereshteh, Amelirad Omid, Moradi Zohre, Habibi Mostafa
2023-Mar-15
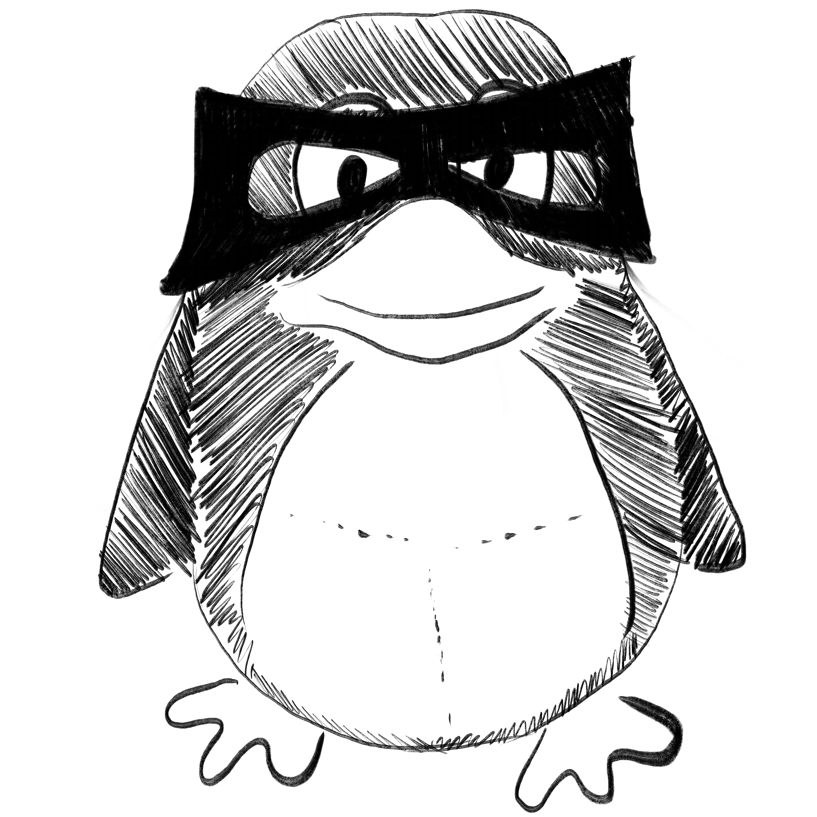
Research on Supply Chain Financial Risk Prevention Based on Machine Learning.
In Computational intelligence and neuroscience
Lei Yang, Qiaoming Hou, Tong Zhao
2023
Hospital Length of Stay Prediction Based on Multi-modal Data towards Trustworthy Human-AI Collaboration in Radiomics
ArXiv Preprint
Hubert Baniecki, Bartlomiej Sobieski, Przemysław Bombiński, Patryk Szatkowski, Przemysław Biecek
2023-03-17
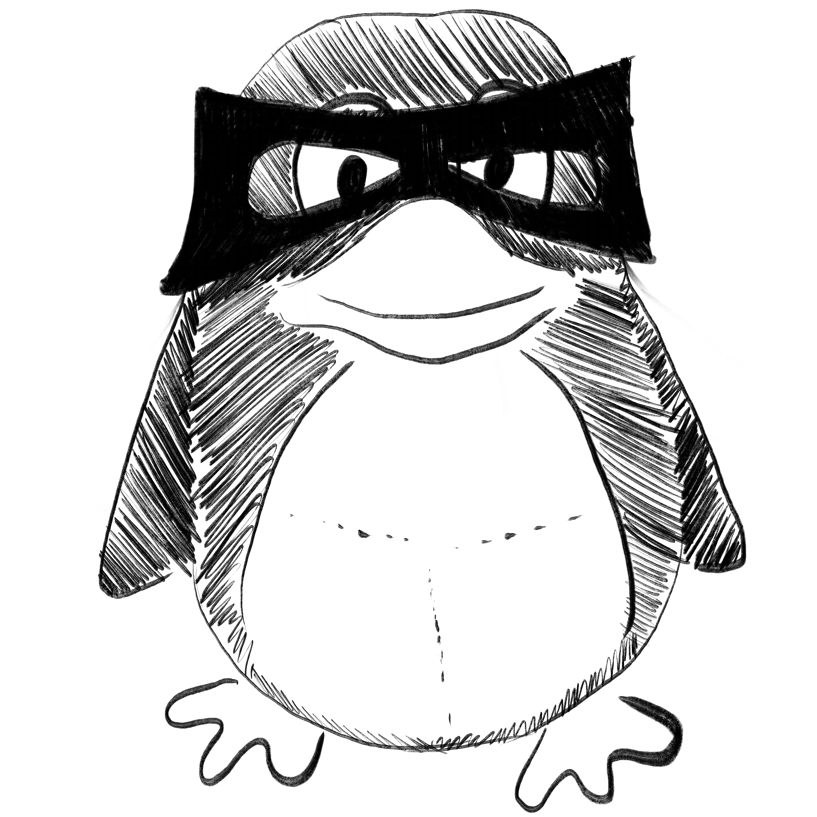
Predicting Workplace Violence in the Emergency Department Based on Electronic Health Record Data.
In Journal of emergency nursing
INTRODUCTION :
METHODS :
RESULTS :
DISCUSSION :
Lee Hyungbok, Yun Heeje, Choi Minjin, Kim Hyeoneui
2023-Mar-14
Electronic health record, Emergency department, Machine learning, Predictive modeling, Workplace aggression
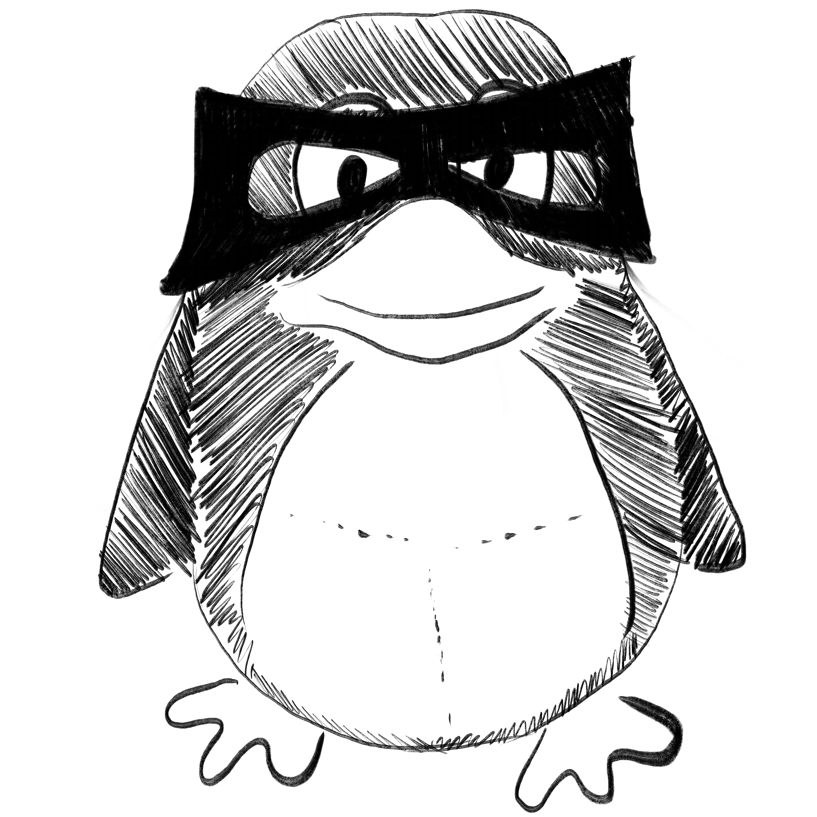
Non-invasive evaluation of embryos using mid-infrared attenuated total reflection spectrometry of incubation medium: a preliminary study.
In Reproductive biomedicine online ; h5-index 47.0
RESEARCH QUESTION :
DESIGN :
RESULTS :
CONCLUSIONS :
Aslih Nardin, Dekel Ben Zion, Malonek Dov, Michaeli Medeia, Polotov Diana, Shalom-Paz Einat
2022-Dec-21
IVF, Mid-infrared attenuated total reflection spectrometry, Morphokinetics, Spectrometry
Weekly Summary
Receive a weekly summary and discussion of the top papers of the week by leading researchers in the field.