Receive a weekly summary and discussion of the top papers of the week by leading researchers in the field.
Characteristics of perivascular space dilatation in normal aging.
In Human brain mapping
Park Chang-Hyun, Shin Na-Young, Nam Yoonho, Yoon Uicheul, Ahn Kookjin, Lee Seung-Koo
2023-Mar-17
age estimation, machine learning, normal aging, perivascular space
Overcoming the challenges to implementation of artificial intelligence in pathology.
In Journal of the National Cancer Institute
Reis-Filho Jorge S, Kather Jakob Nikolas
2023-Mar-17
Artificial intelligence, deep learning, machine learning, oncology, pathology
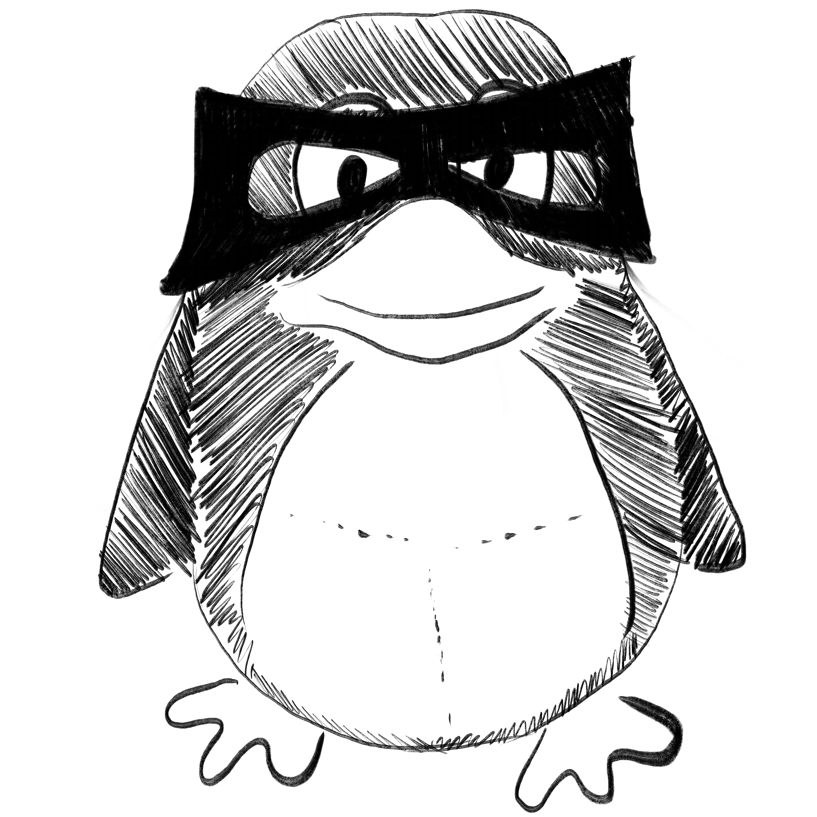
scMCs: a framework for single cell multi-omics data integration and multiple clusterings.
In Bioinformatics (Oxford, England)
MOTIVATION :
RESULTS :
AVAILABILITY :
SUPPLEMENTARY INFORMATION :
Ren Liangrui, Wang Jun, Li Zhao, Li Qingzhong, Yu Guoxian
2023-Mar-17
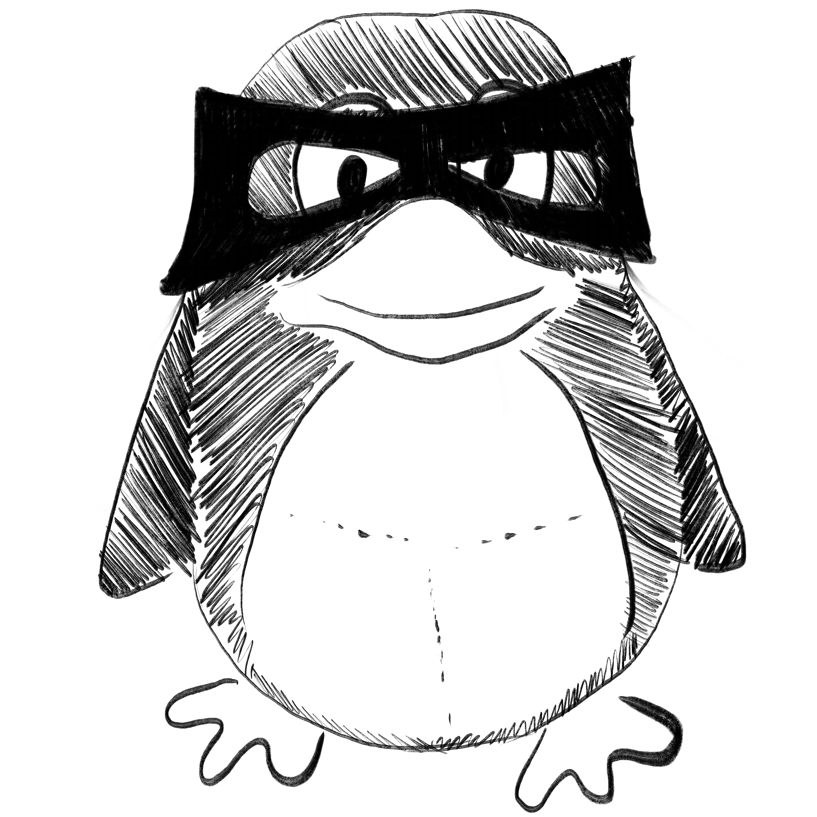
DeepOM: Single-molecule optical genome mapping via deep learning.
In Bioinformatics (Oxford, England)
MOTIVATION :
RESULTS :
AVAILABILITY AND IMPLEMENTATION :
SUPPLEMENTARY INFORMATION :
Nogin Yevgeni, Detinis Zur Tahir, Margalit Sapir, Barzilai Ilana, Alalouf Onit, Ebenstein Yuval, Shechtman Yoav
2023-Mar-17
Direct Estimation of Regional Lung Volume Change from Paired and Single CT Images using Residual Regression Neural Network.
In Medical physics ; h5-index 59.0
BACKGROUND :
PURPOSE :
METHODS :
RESULTS :
CONCLUSIONS :
Gerard Sarah E, Chaudhary Muhammad Fa, Herrmann Jacob, Christensen Gary E, Estépar Raúl San José, Reinhardt Joseph M, Hoffman Eric A
2023-Mar-17
computed tomography, deep learning, pulmonary
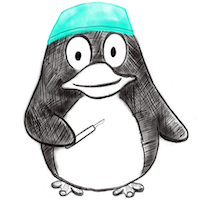
SERS spectroscopy with machine learning to analyze human plasma derived sEVs for coronary artery disease diagnosis and prognosis.
In Bioengineering & translational medicine
Huang Xi, Liu Bo, Guo Shenghan, Guo Weihong, Liao Ke, Hu Guoku, Shi Wen, Kuss Mitchell, Duryee Michael J, Anderson Daniel R, Lu Yongfeng, Duan Bin
2023-Mar
coronary artery disease, diagnostics, machine learning, small extracellular vesicles, spectrogram
Weekly Summary
Receive a weekly summary and discussion of the top papers of the week by leading researchers in the field.