Receive a weekly summary and discussion of the top papers of the week by leading researchers in the field.
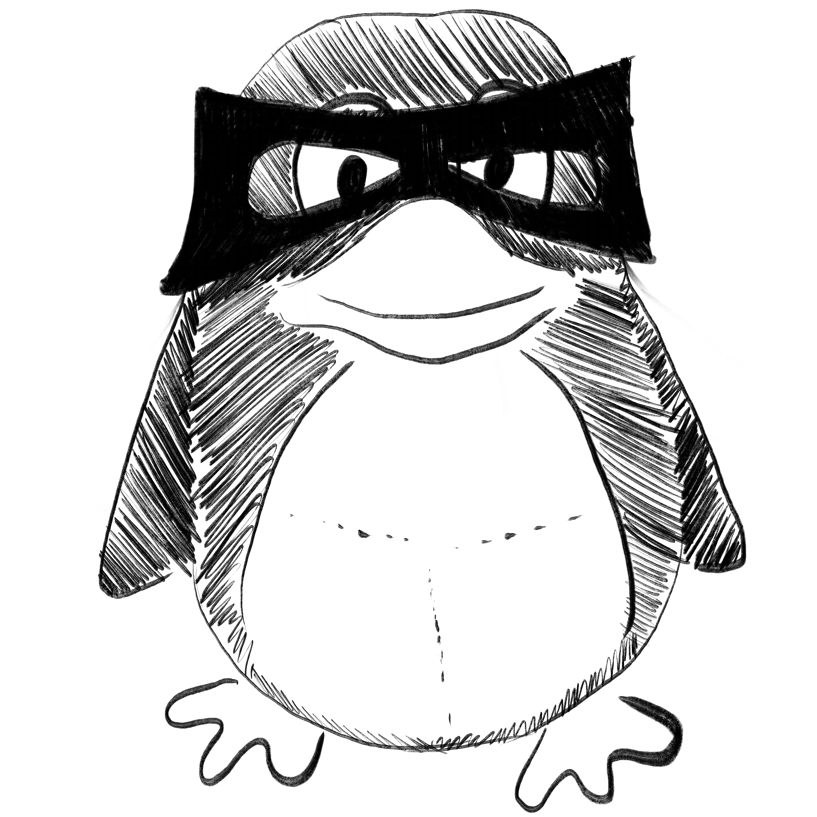
Grading the Severity of Damage to the Perijunctional Actomyosin Ring and Zonula Occludens-1 of the Corneal Endothelium by Ensemble Learning Methods.
In Journal of ocular pharmacology and therapeutics : the official journal of the Association for Ocular Pharmacology and Therapeutics
Shilpashree Palanahalli S, Ravi Tapanmitra, Thanuja M Y, Anupama Chalimeswamy, Ranganath Sudhir H, Suresh Kaggere V, Srinivas Sangly P
2023-Mar-17
ZO-1, barrier function, corneal endothelium, machine learning, perijunctional actomyosin ring, tight junctions
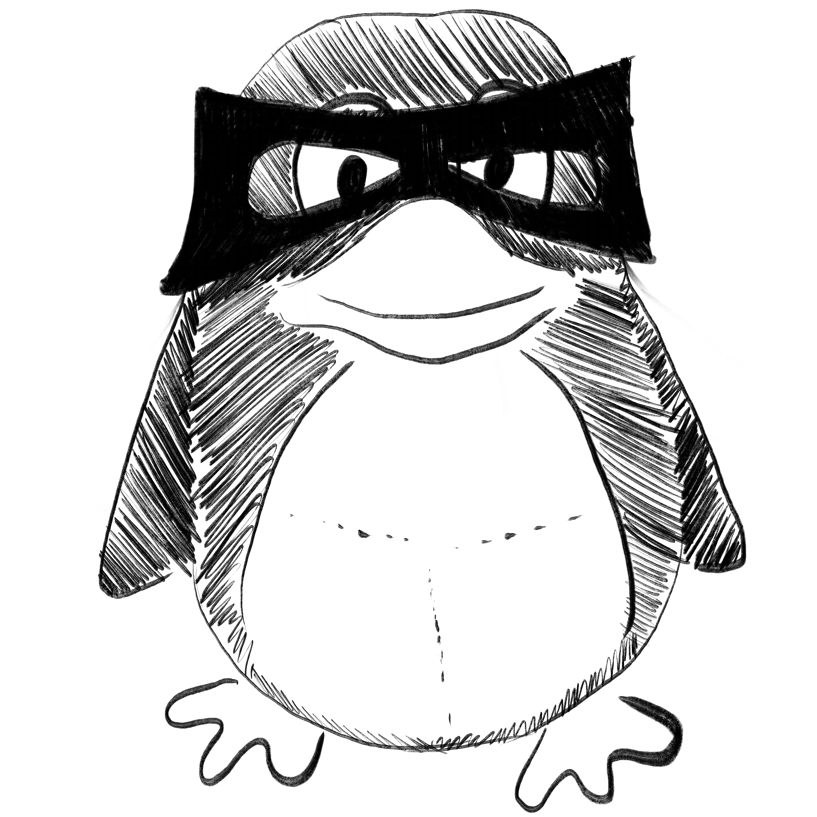
Deep-Learning-Assisted Underwater 3D Tactile Tensegrity.
In Research (Washington, D.C.)
Xu Peng, Zheng Jiaxi, Liu Jianhua, Liu Xiangyu, Wang Xinyu, Wang Siyuan, Guan Tangzhen, Fu Xianping, Xu Minyi, Xie Guangming, Wang Zhong Lin
2023
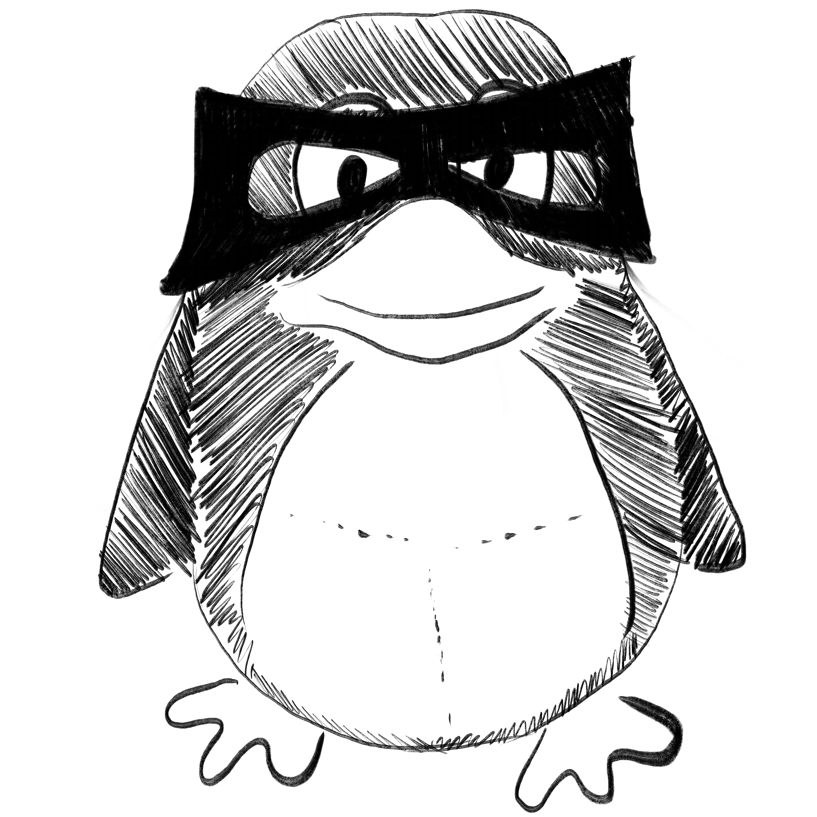
Rational Design of Organelle-Targeted Fluorescent Probes: Insights from Artificial Intelligence.
In Research (Washington, D.C.)
Dong Jie, Qian Jie, Yu Kunqian, Huang Shuai, Cheng Xiang, Chen Fei, Jiang Hualiang, Zeng Wenbin
2023
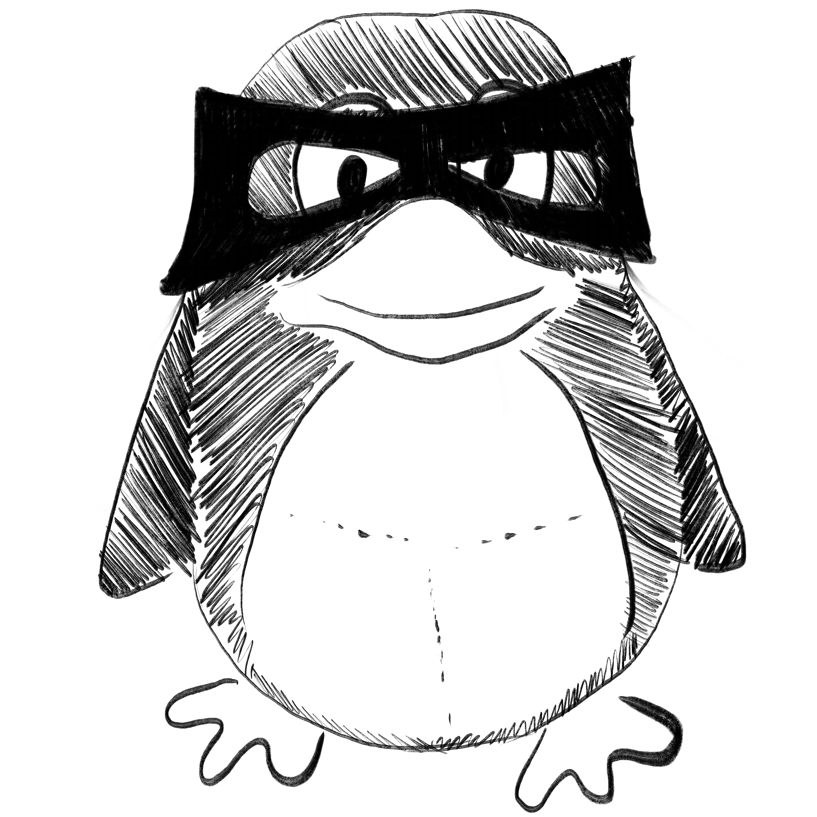
Trends and Potential of Machine Learning and Deep Learning in Drug Study at Single-Cell Level.
In Research (Washington, D.C.)
Qi Ren, Zou Quan
2023
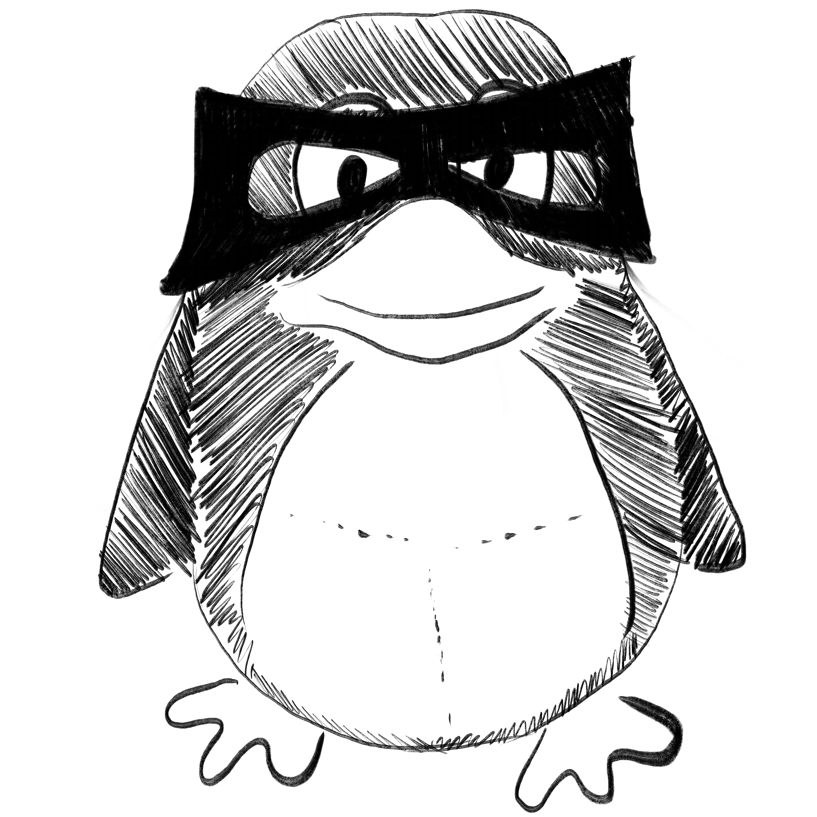
Semi-Self-Supervised Learning for Semantic Segmentation in Images with Dense Patterns.
In Plant phenomics (Washington, D.C.)
Najafian Keyhan, Ghanbari Alireza, Sabet Kish Mahdi, Eramian Mark, Shirdel Gholam Hassan, Stavness Ian, Jin Lingling, Maleki Farhad
2023
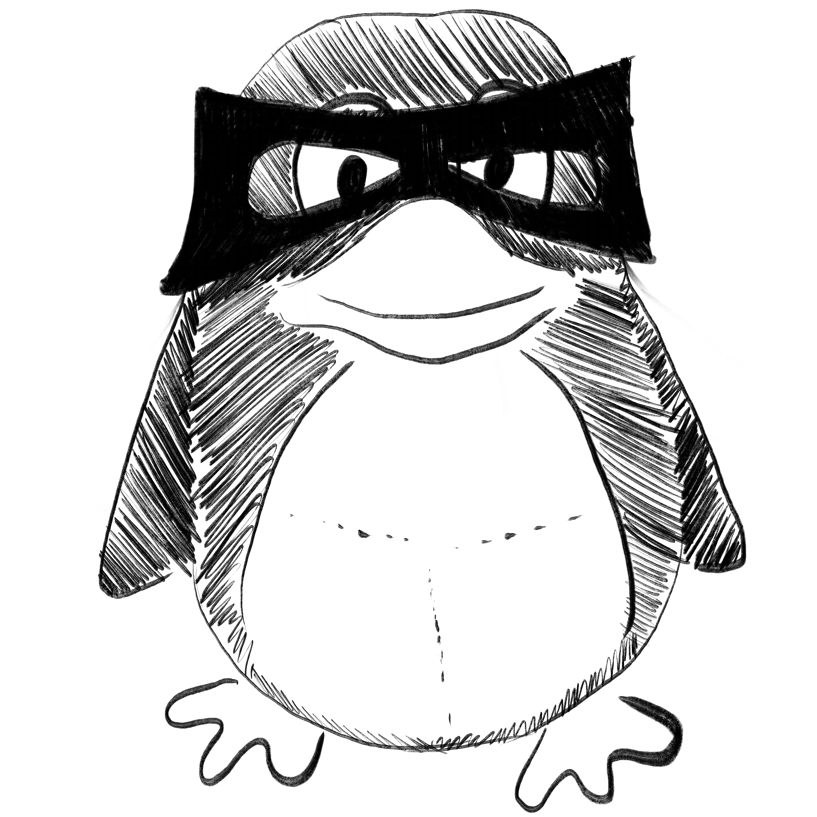
An Effective Integrated Machine Learning Framework for Identifying Severity of Tomato Yellow Leaf Curl Virus and Their Experimental Validation.
In Research (Washington, D.C.)
Bupi Nattanong, Sangaraju Vinoth Kumar, Phan Le Thi, Lal Aamir, Vo Thuy Thi Bich, Ho Phuong Thi, Qureshi Muhammad Amir, Tabassum Marjia, Lee Sukchan, Manavalan Balachandran
2023
Weekly Summary
Receive a weekly summary and discussion of the top papers of the week by leading researchers in the field.