Receive a weekly summary and discussion of the top papers of the week by leading researchers in the field.
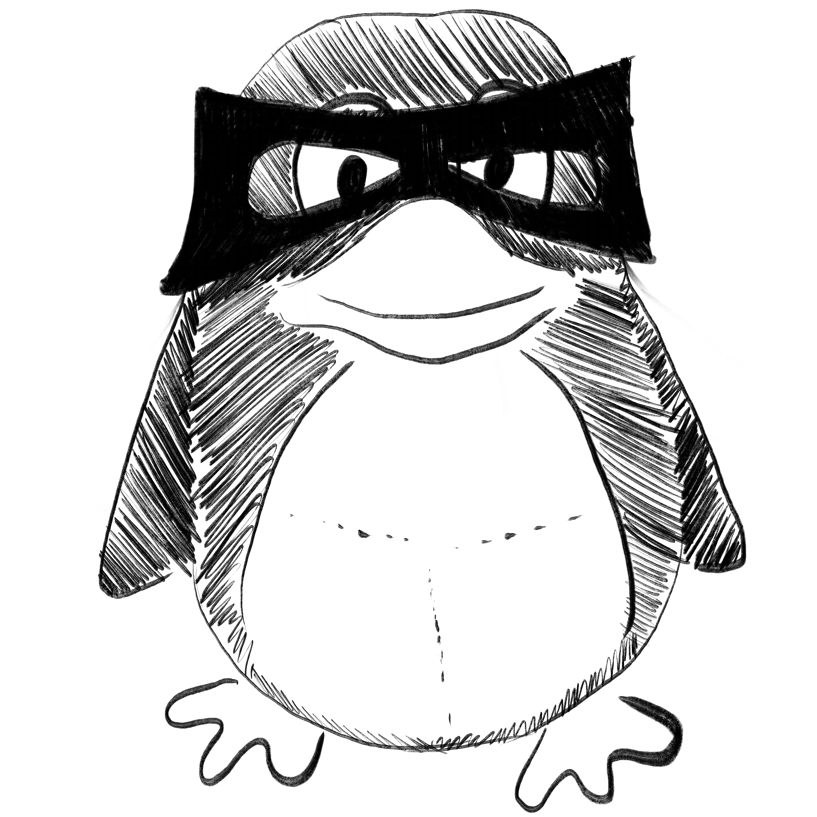
Identifying autophagy-related genes as potential targets for immunotherapy in tuberculosis.
In International immunopharmacology
PURPOSE :
METHODS :
RESULTS :
CONCLUSION :
Xiao Sifang, Zhou Ting, Pan Jianhua, Ma Xiaohua, Shi Guomin, Jiang Binyuan, Xiang Yan-Gen
2023-Mar-15
Autophagy, Immune cell infiltration, Macrophage, Sulforaphane, Tuberculosis
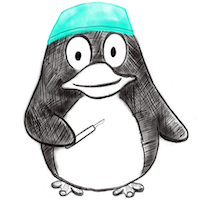
Thyroid Cytopathology Cancer Diagnosis from Smartphone Images Using Machine Learning.
In Modern pathology : an official journal of the United States and Canadian Academy of Pathology, Inc
Assaad Serge, Dov David, Davis Richard, Kovalsky Shahar, Lee Walter T, Kahmke Russel, Rocke Daniel, Cohen Jonathan, Henao Ricardo, Carin Lawrence, Range Danielle Elliott
2023-Feb-13
artificial intelligence, cytopathology, fine needle aspiration, machine learning, mobile imaging, thyroid
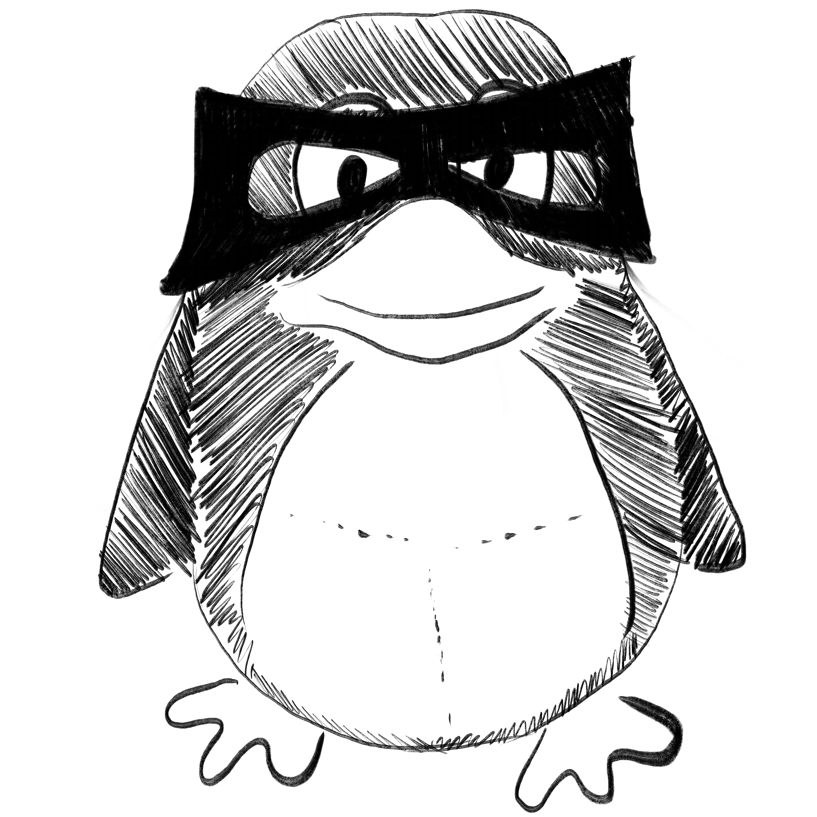
Cost-effectiveness analysis of heart rate characteristics monitoring to improve survival for very low birth weight infants.
In Frontiers in health services
INTRODUCTION :
METHODS :
RESULTS :
CONCLUSION :
King William E, Carlo Waldemar A, O’Shea T Michael, Schelonka Robert L
2022
artificial intelligence (AI), cost-effectiveness analysis (CEA), heart rate characteristics (HRC), incremental cost-effectiveness ratio (ICER), neonatal intensive care unit (NICU), newborn infant, randomized controlled trial (RCT), very low birth weight (VLBW)
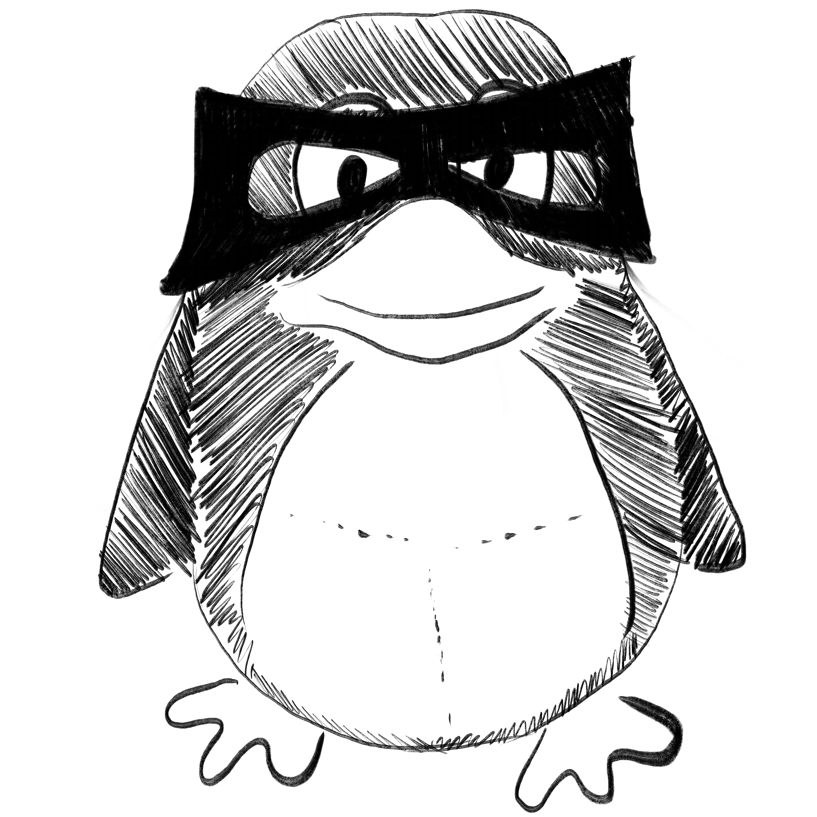
Integration- and separation-aware adversarial model for cerebrovascular segmentation from TOF-MRA.
In Computer methods and programs in biomedicine
PURPOSE :
METHODS :
RESULTS :
CONCLUSION :
Chen Cheng, Zhou Kangneng, Lu Tong, Ning Huansheng, Xiao Ruoxiu
2023-Mar-11
Adversarial model, Cerebrovascular segmentation, Deep learning, TOF-MRA
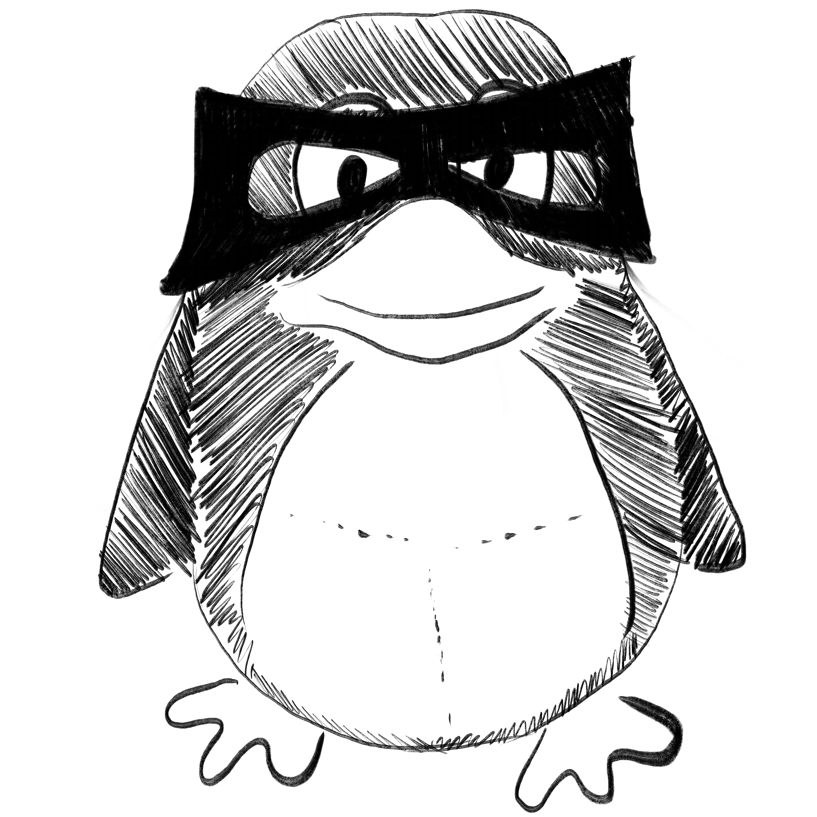
Integrating machine learning with linguistic features: A universal method for extraction and normalization of temporal expressions in Chinese texts.
In Computer methods and programs in biomedicine
BACKGROUND AND OBJECTIVE :
METHODS :
RESULTS :
CONCLUSIONS :
Wang Shunli, Li Rui, Wu Huayi
2023-Mar-11
Extraction and normalization of temporal expressions, Linguistic features, Online medical conversation, Temporal reasoning
Long-Term Performance of an Image-Based Short-Term Risk Model for Breast Cancer.
In Journal of clinical oncology : official journal of the American Society of Clinical Oncology
PURPOSE :
METHODS :
RESULTS :
CONCLUSION :
Eriksson Mikael, Czene Kamila, Vachon Celine, Conant Emily F, Hall Per
2023-Mar-17
Weekly Summary
Receive a weekly summary and discussion of the top papers of the week by leading researchers in the field.