Receive a weekly summary and discussion of the top papers of the week by leading researchers in the field.
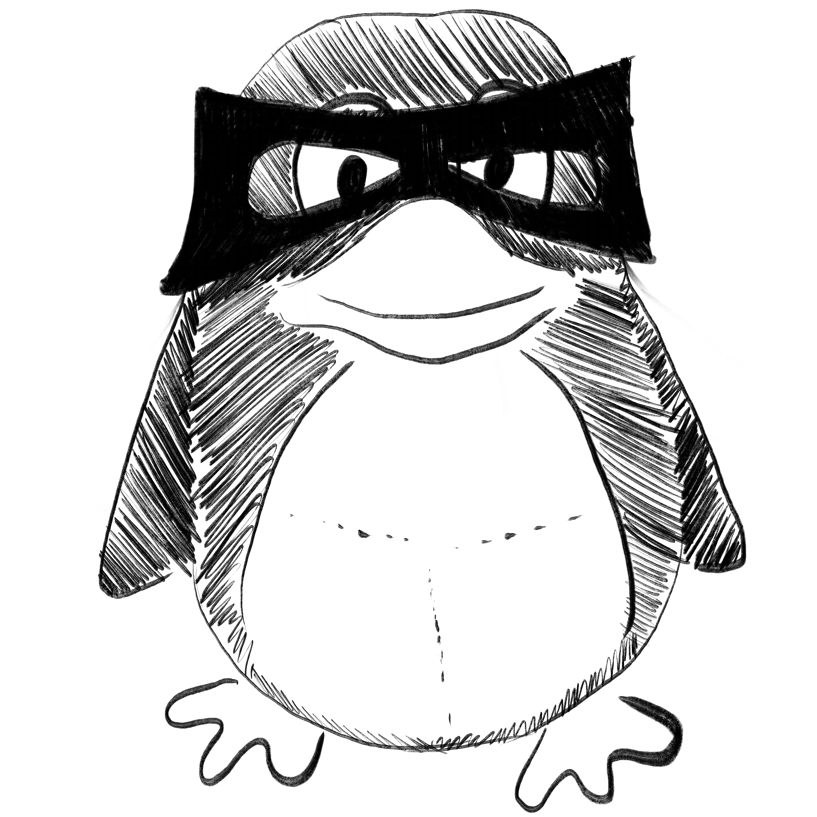
Machine learning-based automated sponge cytology for screening of oesophageal squamous cell carcinoma and adenocarcinoma of the oesophagogastric junction: a nationwide, multicohort, prospective study.
In The lancet. Gastroenterology & hepatology
BACKGROUND :
METHODS :
FINDINGS :
INTERPRETATION :
FUNDING :
Gao Ye, Xin Lei, Lin Han, Yao Bin, Zhang Tao, Zhou Ai-Jun, Huang Shu, Wang Jian-Hua, Feng Ya-Dong, Yao Sheng-Hua, Guo Yan, Dang Tong, Meng Xian-Mei, Yang Zeng-Zhou, Jia Wan-Qi, Pang Hui-Fang, Tian Xiao-Juan, Deng Bin, Wang Jun-Ping, Fan Wen-Chuan, Wang Jun, Shi Li-Hong, Yang Guan-Yu, Sun Chang, Wang Wei, Zang Jun-Cai, Li Song-Yang, Shi Rui-Hua, Li Zhao-Shen, Wang Luo-Wei
2023-Mar-14
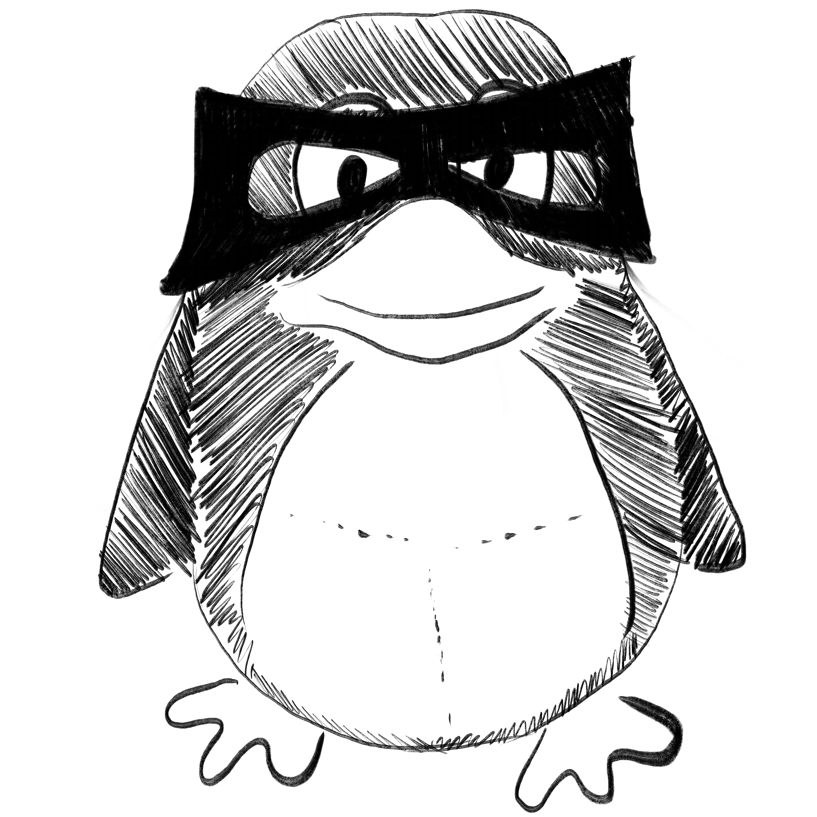
A gated temporal-separable attention network for EEG-based depression recognition.
In Computers in biology and medicine
Yang Lijun, Wang Yixin, Zhu Xiangru, Yang Xiaohui, Zheng Chen
2023-Mar-11
Attention mechanism, Depression recognition, Electroencephalography (EEG), Gated recurrent unit, Temporal convolution network
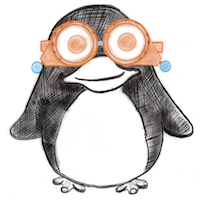
A new ultra-wide-field fundus dataset to diabetic retinopathy grading using hybrid preprocessing methods.
In Computers in biology and medicine
Liu Haomiao, Teng Lu, Fan Linhua, Sun Yabin, Li Huiying
2023-Mar-08
Convolutional neural network, Deep learning, Diabetic retinopathy grading, Ultra-wide-field, Vision transformer
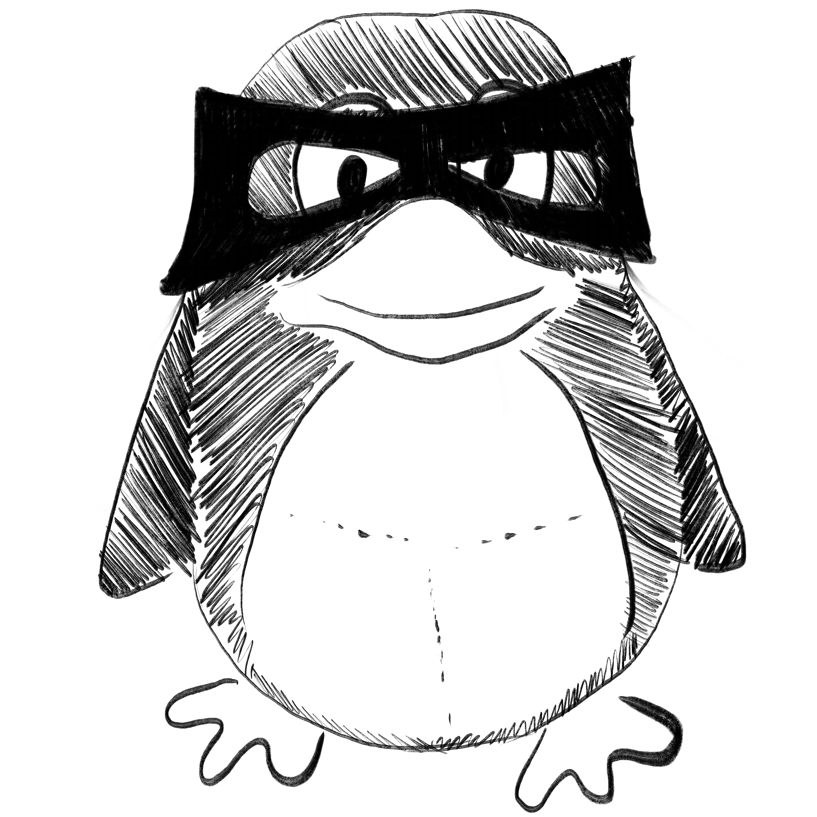
Identification of medicinal plant-based phytochemicals as a potential inhibitor for SARS-CoV-2 main protease (Mpro) using molecular docking and deep learning methods.
In Computers in biology and medicine
Hossain Alomgir, Rahman Md Ekhtiar, Rahman Md Siddiqur, Nasirujjaman Khondokar, Matin Mohammad Nurul, Faruqe Md Omar, Rabbee Muhammad Fazle
2023-Mar-11
Catechin gallate, Deep learning, Main protease, Molecular docking, SARS-CoV-2
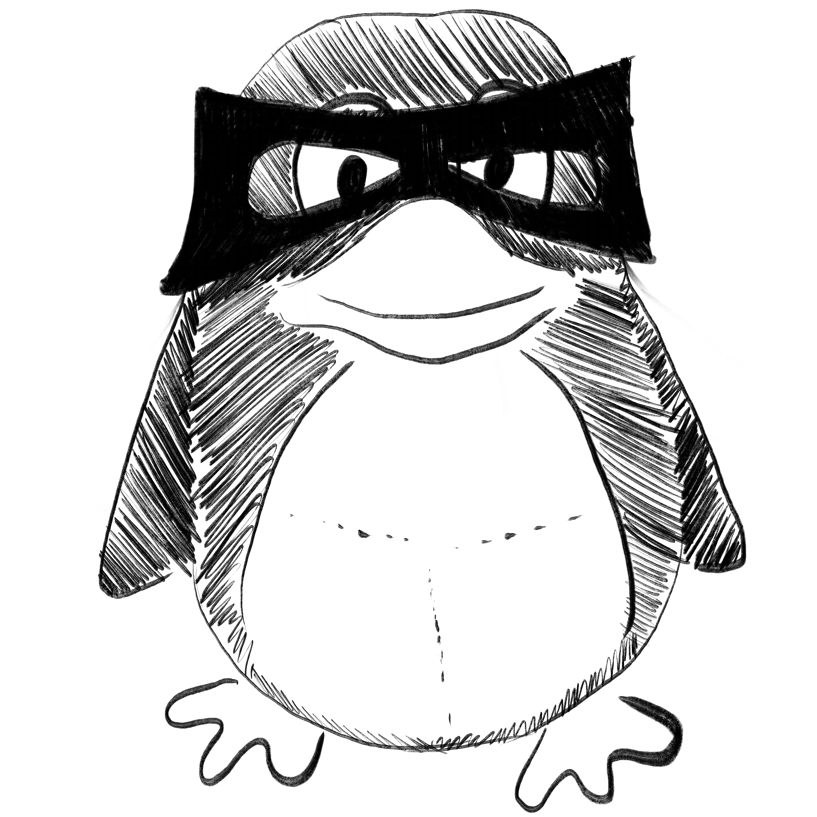
Upscaling dispersivity for conservative solute transport in naturally fractured media.
In Water research
Jia Sida, Dai Zhenxue, Zhou Zhichao, Ling Hui, Yang Zhijie, Qi Linlin, Wang Zihao, Zhang Xiaoying, Thanh Hung Vo, Soltanian Mohamad Reza
2023-Mar-15
Dispersivity, Fracture, Lagrangian-based model, Random walk particle tracking, Sensitivity analysis, Transport experiment
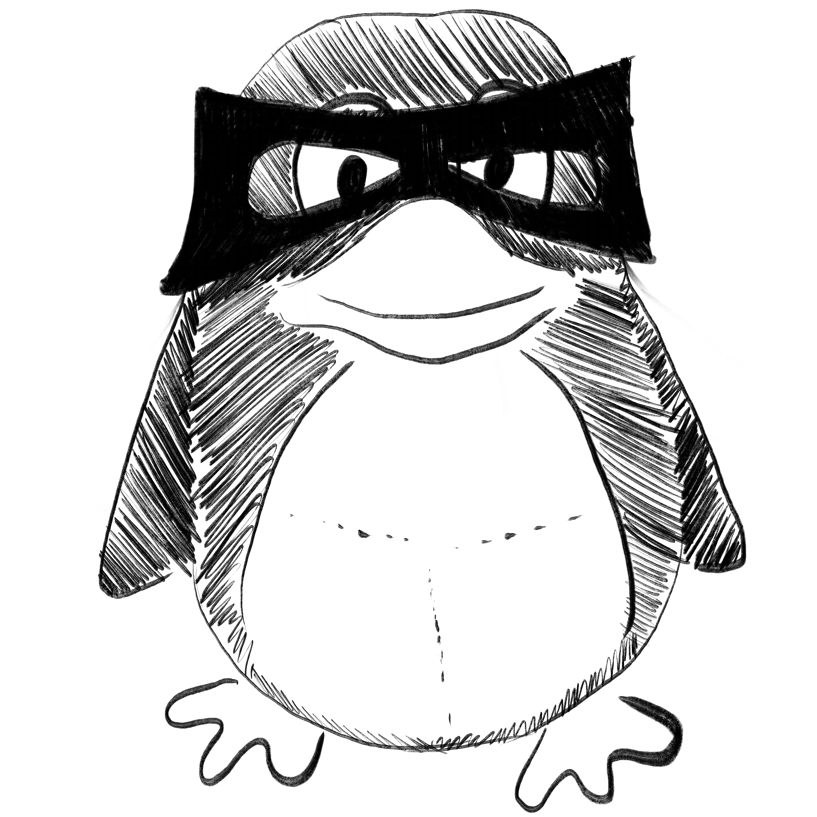
Feature selection for driving style and skill clustering using naturalistic driving data and driving behavior questionnaire.
In Accident; analysis and prevention
Chen Yao, Wang Ke, Lu Jian John
2023-Mar-15
Cluster analysis, Driving behavior, Driving behavior questionnaire, Driving skill, Driving style, Feature selection
Weekly Summary
Receive a weekly summary and discussion of the top papers of the week by leading researchers in the field.