Receive a weekly summary and discussion of the top papers of the week by leading researchers in the field.
Can we design the next generation of digital health communication programs by leveraging the power of artificial intelligence to segment target audiences, bolster impact and deliver differentiated services? A machine learning analysis of survey data from rural India.
In BMJ open
OBJECTIVES :
SETTING :
PARTICIPANTS :
CONCLUSIONS :
TRIAL REGISTRATION NUMBER :
Bashingwa Jean Juste Harrisson, Mohan Diwakar, Chamberlain Sara, Scott Kerry, Ummer Osama, Godfrey Anna, Mulder Nicola, Moodley Deshendran, LeFevre Amnesty Elizabeth
2023-Mar-17
community child health, information technology, public health
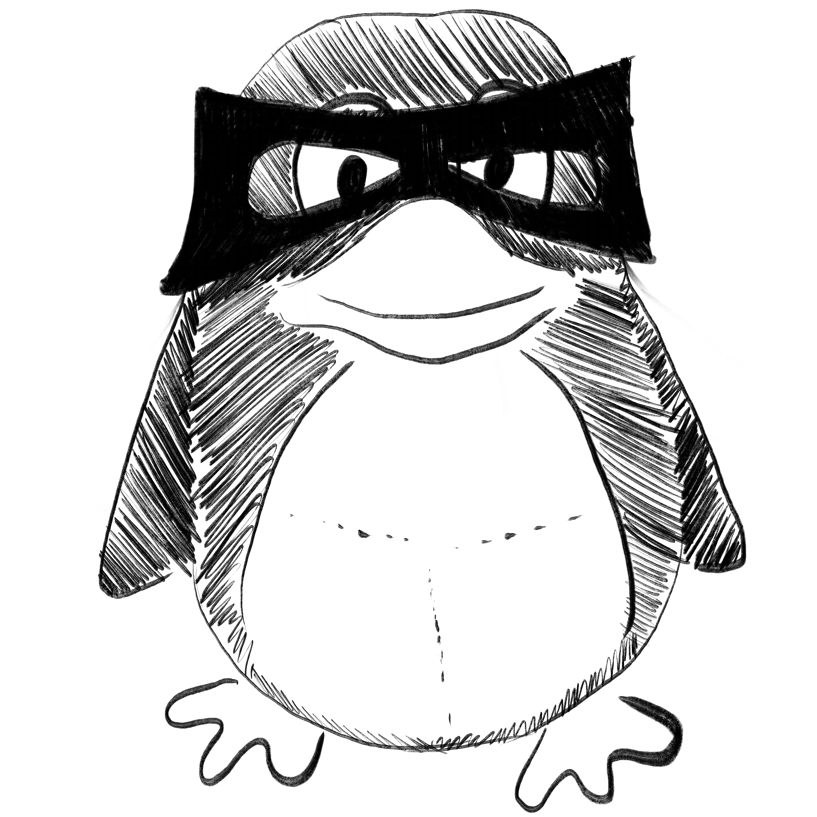
Machine learning approaches in diagnosing tuberculosis through biomarkers - A systematic review.
In Progress in biophysics and molecular biology
Balakrishnan Vimala, Kehrabi Yousra, Ramanathan Ghayathri, Paul Scott Arjay, Tiong Chiong Kian
2023-Mar-15
Biomarker, Diagnostic, Machine learning, Systematic review, Tuberculosis
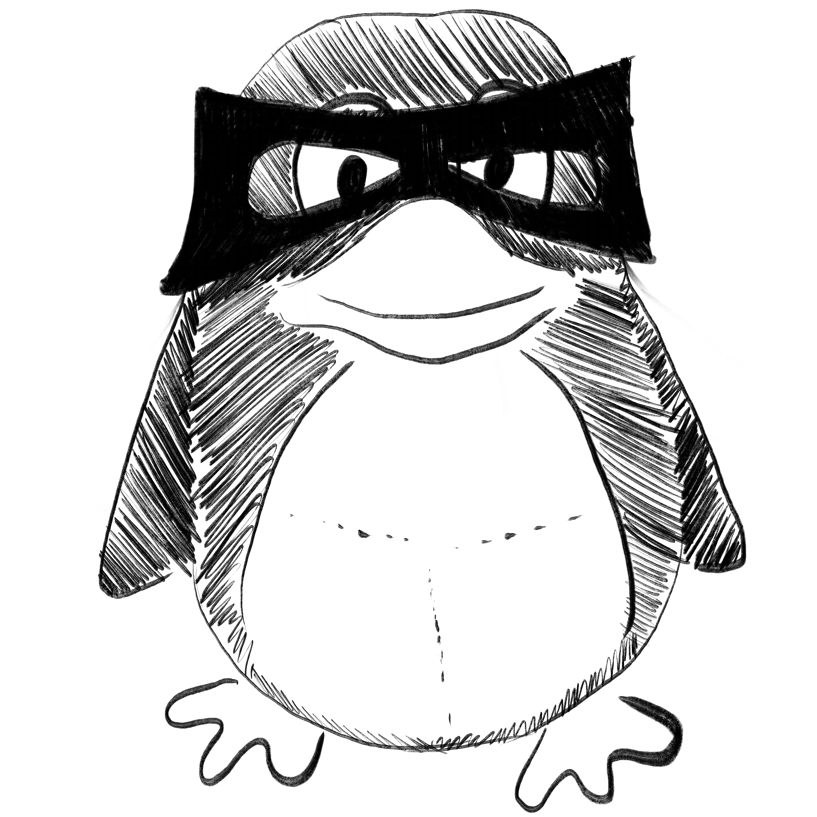
Organophosphate esters in soils of Beijing urban parks: Occurrence, potential sources, and probabilistic health risks.
In The Science of the total environment
Tian Y X, Wang Y, Chen H Y, Ma J, Liu Q Y, Qu Y J, Sun H W, Wu L N, Li X L
2023-Mar-15
Concentration prediction, Influencing factors, OPEs, Probabilistic health risk, Source identification
Assessment of the implementation context in preparation for a clinical study of machine-learning algorithms to automate the classification of digital cervical images for cervical cancer screening in resource-constrained settings.
In Frontiers in health services
INTRODUCTION :
METHODS :
RESULTS :
CONCLUSION :
Castor Delivette, Saidu Rakiya, Boa Rosalind, Mbatani Nomonde, Mutsvangwa Tinashe E M, Moodley Jennifer, Denny Lynette, Kuhn Louise
2022
Automated Visual Evaluation, Automated Visual Evaluation of the cervix, CFIR, CFIR framework, cervical cancer, digital cervical-cancer screening implementation assessment cervical cancer, implementation science
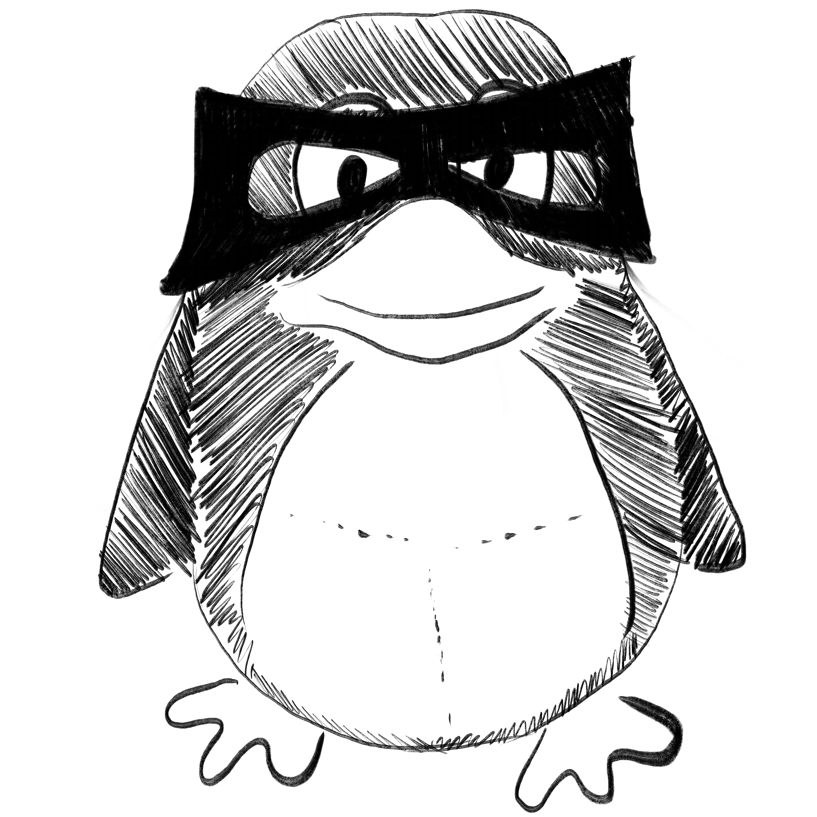
Characteristics Prediction of Hydrothermal Biochar Using Data Enhanced Interpretable Machine Learning.
In Bioresource technology
Chen Chao, Wang Zhi, Ge Yadong, Liang Rui, Hou Donghao, Tao Junyu, Yan Beibei, Zheng Wandong, Velichkova Rositsa, Chen Guanyi
2023-Mar-15
Correlation Analysis, Feature Analysis, Hydrothermal Carbonization, Random Forest
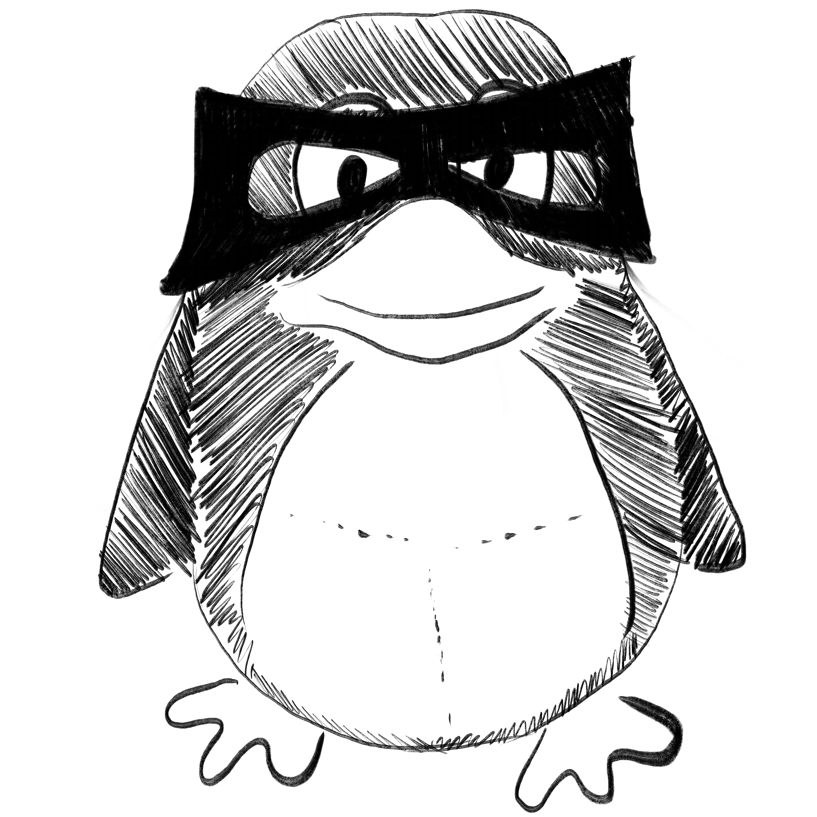
Optimization of depth of filler media in horizontal flow constructed wetlands for maximizing removal rate coefficients of targeted pollutant(s).
In Bioresource technology
Singh Saurabh, Soti Abhishek, Mohan Kulshreshtha Niha, Kumar Nikhil, Brighu Urmila, Bhushan Gupta Akhilendra, Bezbaruah Achintya N
2023-Mar-15
Grey Wolf Optimization (GWO), horizontal flow constructed wetlands (HFCWs), major functional microorganisms, optimum depth, volumetric removal rate coefficients
Weekly Summary
Receive a weekly summary and discussion of the top papers of the week by leading researchers in the field.