Receive a weekly summary and discussion of the top papers of the week by leading researchers in the field.
Deep Learning-Based Computer-Aided Detection System for Preoperative Chest Radiographs to Predict Postoperative Pneumonia.
In Academic radiology
RATIONALE AND OBJECTIVES :
RESULTS :
CONCLUSION :
Lee Taehee, Hwang Eui Jin, Park Chang Min, Goo Jin Mo
2023-Mar-15
Deep learning, Pneumonia, Postoperative complications, Preoperative risk screening, Thoracic radiography
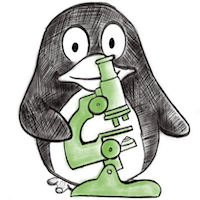
The use of artificial intelligence to detect students' sentiments and emotions in gross anatomy reflections.
In Anatomical sciences education
Rechowicz Krzysztof J, Elzie Carrie A
2023-Mar-17
dissection, gross anatomy, health professions education, machine learning, natural language processing, reflections, reflective writing, sentiment analysis
Modern Imaging of Aneurysmal Subarachnoid Hemorrhage.
In Radiologic clinics of North America
Levinson Simon, Pendharkar Arjun V, Gauden Andrew J, Heit Jeremy J
2023-May
AI, Aneurysm, Brain, CT, Hemorrhage, Imaging
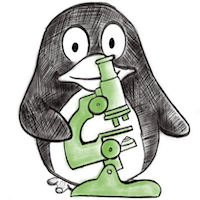
Noninferiority of Artificial Intelligence-Assisted Analysis of Ki-67 and Estrogen/Progesterone Receptor in Breast Cancer Routine Diagnostics.
In Modern pathology : an official journal of the United States and Canadian Academy of Pathology, Inc
Abele Niklas, Tiemann Katharina, Krech Till, Wellmann Axel, Schaaf Christian, Länger Florian, Peters Anja, Donner Andreas, Keil Felix, Daifalla Khalid, Mackens Marina, Mamilos Andreas, Minin Evgeny, Krümmelbein Michel, Krause Linda, Stark Maria, Zapf Antonia, Päpper Marc, Hartmann Arndt, Lang Tobias
2023-Mar
digital pathology, mammary carcinoma, surgical pathology
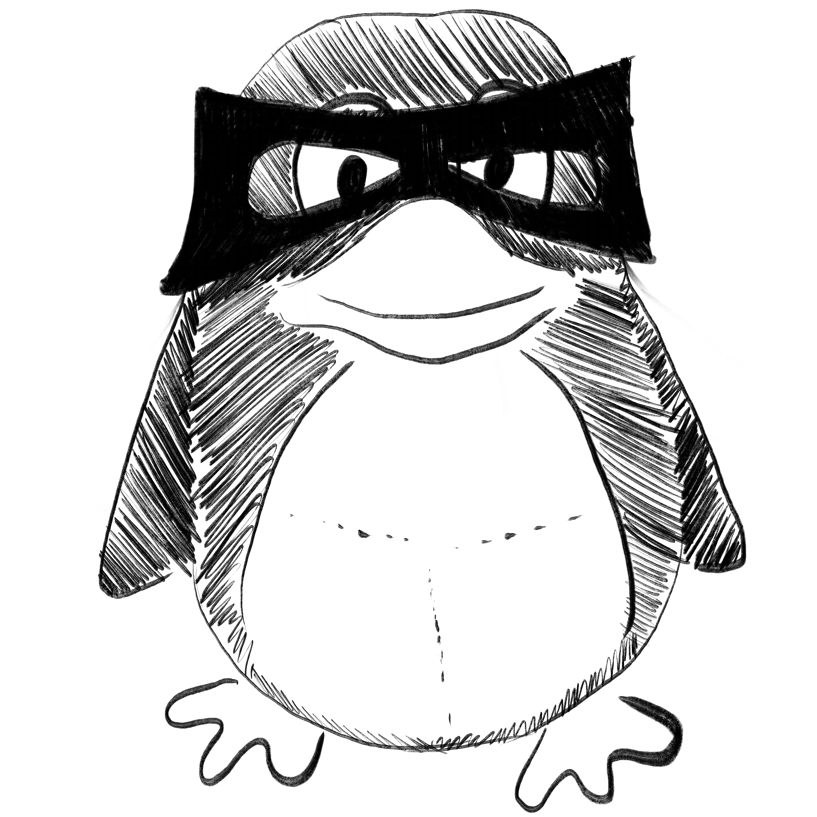
Glutamatergic and GABAergic receptor modulation present unique electrophysiological fingerprints in a concentration-dependent and region-specific manner.
In eNeuro
Gonzalez-Burgos Irene, Bainier Marie, Gross Simon, Schoenenberger Philipp, Ochoa-Martinez José A, Valencia Miguel, Redondo Roger L
2023-Mar-16
Diazepam, EEG, GABA, MK-801, NMDA, parametrization
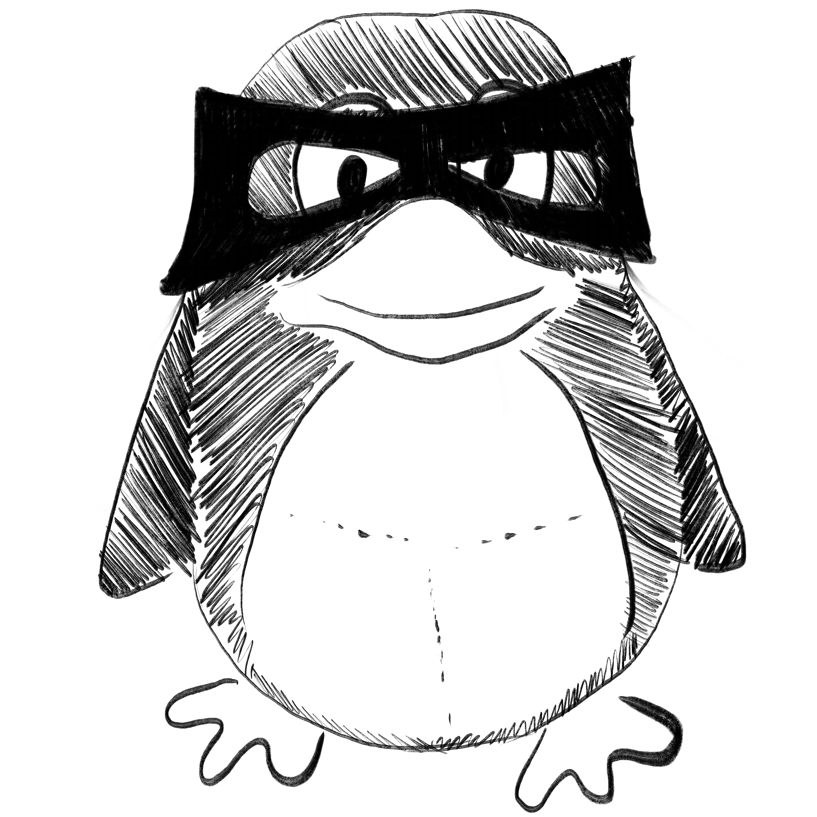
Review of Natural Language Processing in Pharmacology.
In Pharmacological reviews ; h5-index 63.0
Trajanov Dimitar, Trajkovski Vangel, Dimitrieva Makedonka, Dobreva Jovana, Jovanovik Milos, Klemen Matej, Žagar Aleš, Robnik-Šikonja Marko
2023-Mar-17
adverse drug reactions, drug analysis, drug discovery
Weekly Summary
Receive a weekly summary and discussion of the top papers of the week by leading researchers in the field.