Receive a weekly summary and discussion of the top papers of the week by leading researchers in the field.
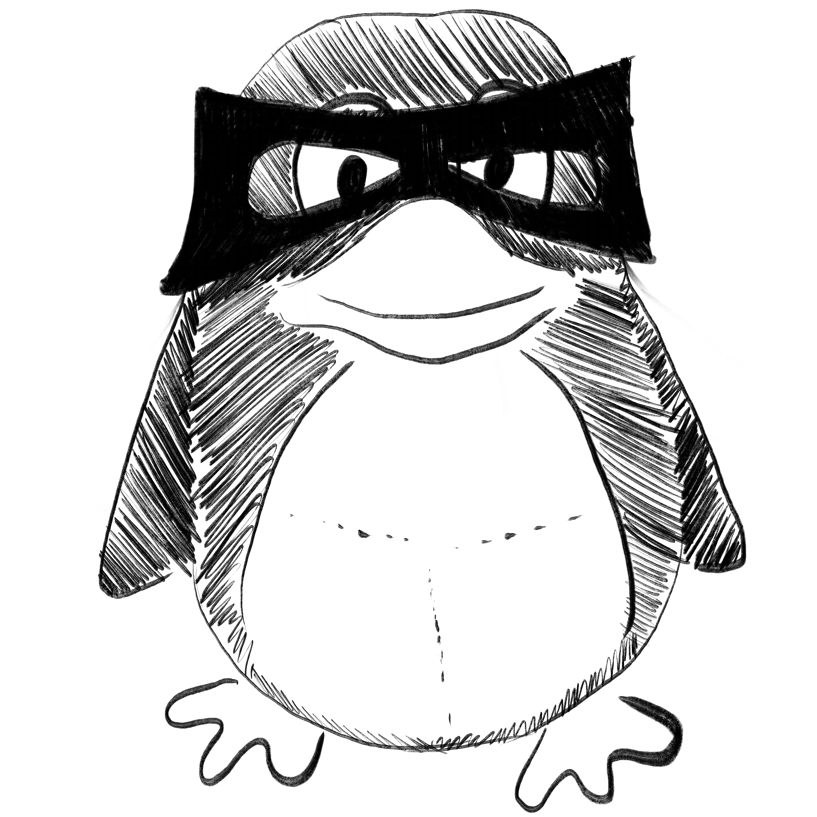
Investigating mental health outcomes of undergraduates and graduate students in Taiwan during the COVID-19 pandemic.
In Journal of American college health : J of ACH
Lin Ching-Hui, Lin Szu-Yin, Hu Bo-Hsien, Lo C Owen
2023-Jan-03
College students, Covid-19, mental health, postsecondary education
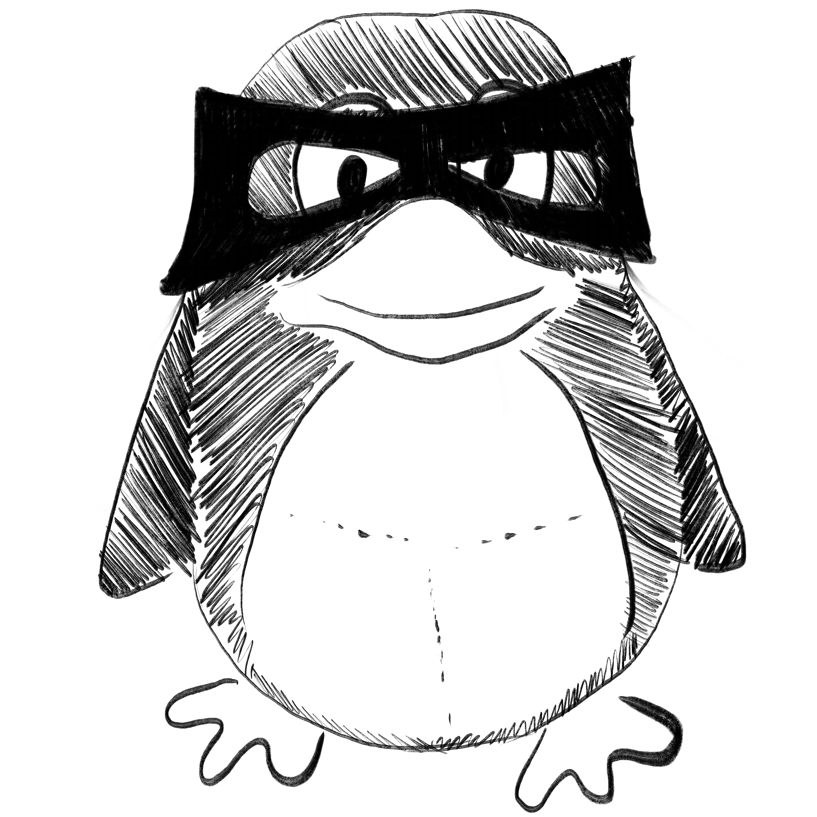
Users' Reactions on Announced Vaccines against COVID-19 Before Marketing in France: Analysis of Twitter posts.
In Journal of medical Internet research ; h5-index 88.0
BACKGROUND :
OBJECTIVE :
METHODS :
RESULTS :
CONCLUSIONS :
Dupuy-Zini Alexandre, Audeh Bissan, Gérardin Christel, Duclos Catherine, Gagneux-Brunon Amandine, Bousquet Cedric
2022-Aug-09
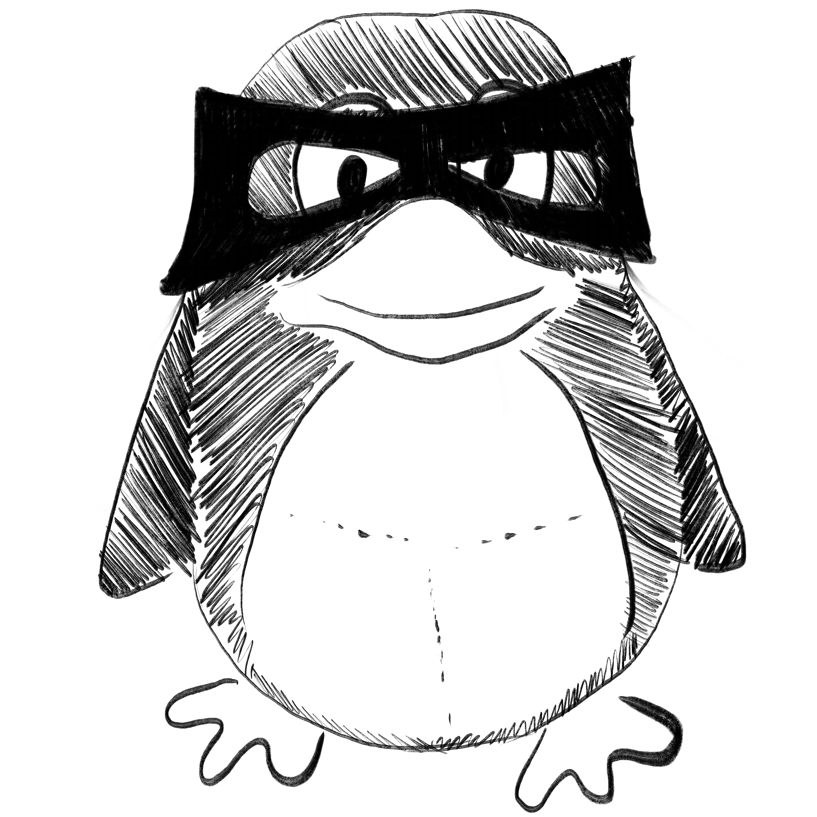
Embedding-based link predictions to explore latent comorbidity of chronic diseases.
In Health information science and systems
PURPOSE :
METHODS :
RESULTS :
CONCLUSION :
Lu Haohui, Uddin Shahadat
2023-Dec
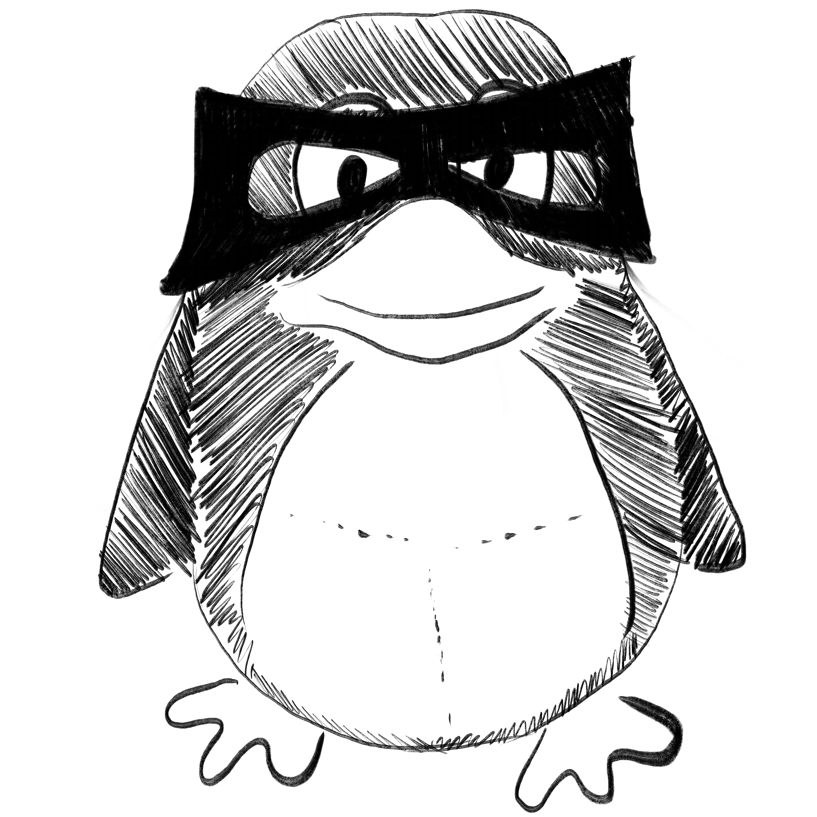
COVID-19 Detection: A Systematic Review of Machine and Deep Learning-Based Approaches Utilizing Chest X-Rays and CT Scans.
In Cognitive computation
Bhatele Kirti Raj, Jha Anand, Tiwari Devanshu, Bhatele Mukta, Sharma Sneha, Mithora Muktasha R, Singhal Stuti
2022-Dec-29
COVID-19, CT scan, Chest X-ray, Deep transfer learning, Machine learning
Machine Learning and Nomogram Prognostic Modeling for 2-Year Head and Neck Cancer-Specific Survival Using Electronic Health Record Data: A Multisite Study.
In JCO clinical cancer informatics
PURPOSE :
MATERIALS AND METHODS :
RESULTS :
CONCLUSION :
Kotevski Damian P, Smee Robert I, Vajdic Claire M, Field Matthew
2023-Jan
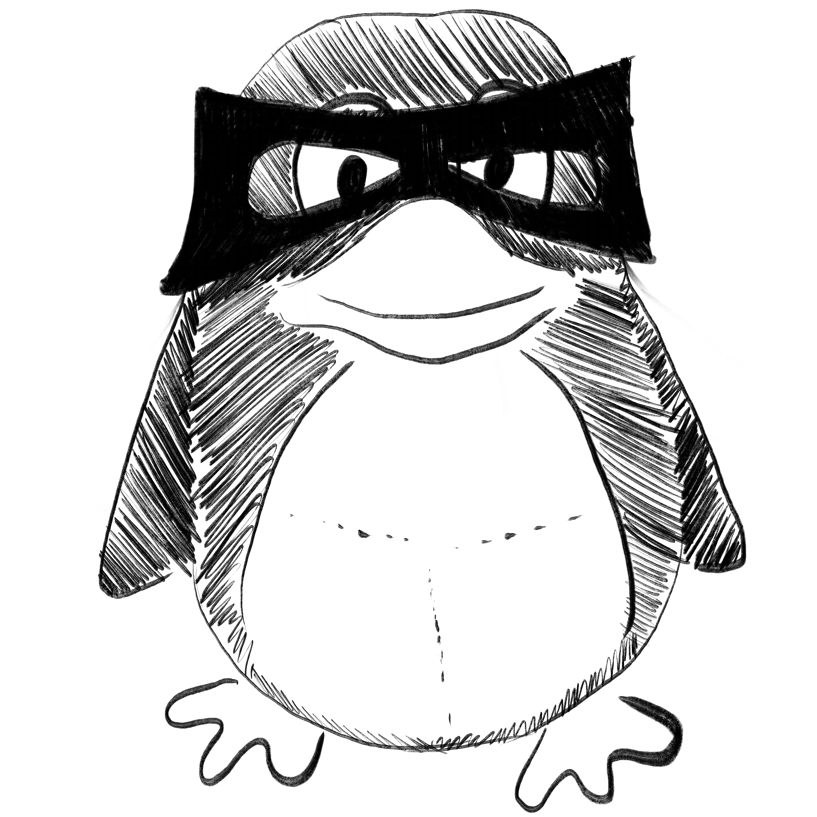
Strategies for the content determination of capsaicin and the identification of adulterated pepper powder using a hand-held near-infrared spectrometer.
In Food research international (Ottawa, Ont.)
Wu Sijun, Wang Long, Zhou Guoming, Liu Chao, Ji Zhongrui, Li Zheng, Li Wenlong
2023-Jan
Adulteration, Capsaicin, Ensemble preprocessing, Grey wolf optimizer, Hand-held near-infrared spectrometer, Machine learning, Pepper powder
Weekly Summary
Receive a weekly summary and discussion of the top papers of the week by leading researchers in the field.