Receive a weekly summary and discussion of the top papers of the week by leading researchers in the field.
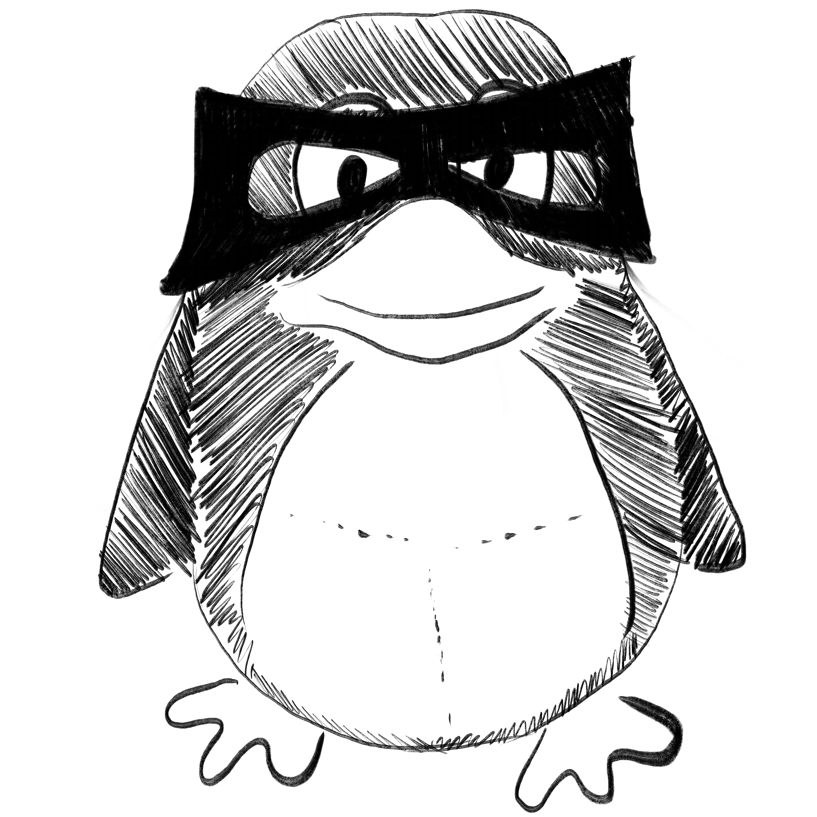
Combining distance and anatomical information for deep-learning based dose distribution predictions for nasopharyngeal cancer radiotherapy planning.
In Frontiers in oncology
PURPOSE :
MATERIALS AND METHODS :
RESULTS :
CONCLUSIONS :
Chen Xinyuan, Zhu Ji, Yang Bining, Chen Deqi, Men Kuo, Dai Jianrong
2023
anatomical information, deep-learning, dose-prediction, minimum distance, radiotherapy treatment planning
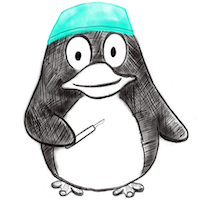
MRI-based pre-Radiomics and delta-Radiomics models accurately predict the post-treatment response of rectal adenocarcinoma to neoadjuvant chemoradiotherapy.
In Frontiers in oncology
OBJECTIVES :
METHODS :
RESULTS :
CONCLUSION :
Wang Likun, Wu Xueliang, Tian Ruoxi, Ma Hongqing, Jiang Zekun, Zhao Weixin, Cui Guoqing, Li Meng, Hu Qinsheng, Yu Xiangyang, Xu Wengui
2023
MRI, machine learning, neoadjuvant chemoradiotherapy, radiomics, rectal adenocarcinoma
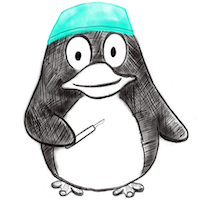
Current practises and the future of robotic surgical training.
In The surgeon : journal of the Royal Colleges of Surgeons of Edinburgh and Ireland
INTRODUCTION :
METHODS :
TRAINING PROGRAMMES :
OPERATIVE PERFORMANCE ASSESSMENT :
FUTURE ADVANCES :
CONCLUSION :
Sinha Ankit, West Alexander, Vasdev Nikhil, Sooriakumaran Prasanna, Rane Abhay, Dasgupta Prokar, McKirdy Michael
2023-Mar-15
Education, Laparoscopic, Surgical training, Training & assessment
Characterizing Female Firearm Suicide Circumstances: A Natural Language Processing and Machine Learning Approach.
In American journal of preventive medicine ; h5-index 75.0
INTRODUCTION :
METHODS :
RESULTS :
CONCLUSIONS :
Goldstein Evan V, Mooney Stephen J, Takagi-Stewart Julian, Agnew Brianna F, Morgan Erin R, Haviland Miriam J, Zhou Weipeng, Prater Laura C
2023-Mar-16
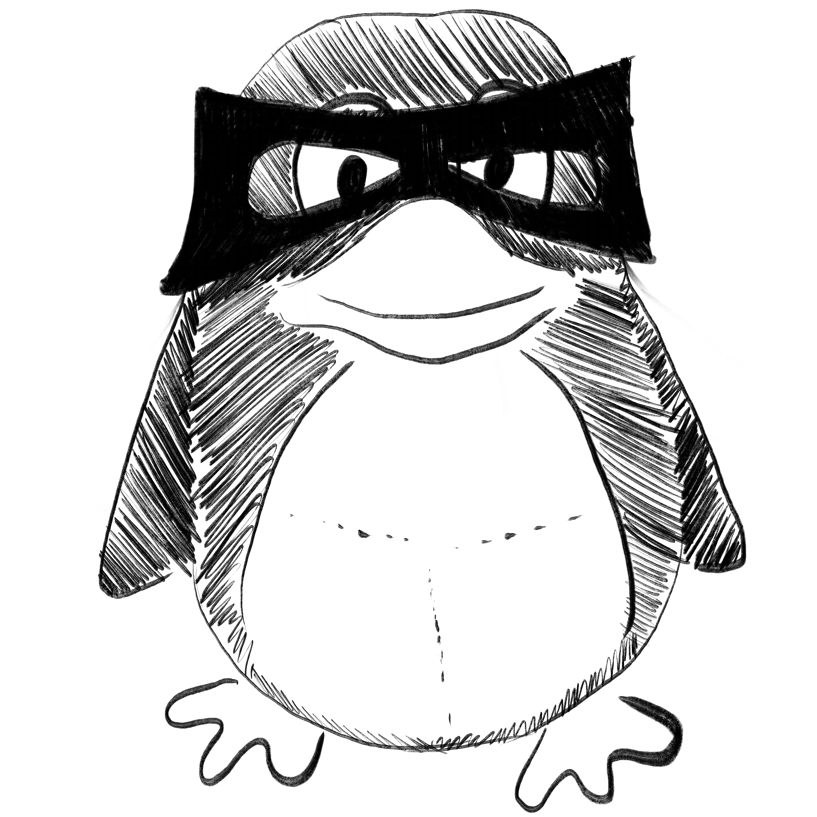
Realizing the potential of artificial intelligence in healthcare: Learning from intervention, innovation, implementation and improvement sciences.
In Frontiers in health services
INTRODUCTION :
AIM :
UTILIZING KNOWLEDGE FROM THE FOUR FIELDS :
CONCLUSION :
Nilsen Per, Reed Julie, Nair Monika, Savage Carl, Macrae Carl, Barlow James, Svedberg Petra, Larsson Ingrid, Lundgren Lina, Nygren Jens
2022
artificial intelligence, implementation, improvement, innovation, intervention
Clinical Implementation of an Artificial Intelligence Algorithm for Magnetic Resonance-Derived Measurement of Total Kidney Volume.
In Mayo Clinic proceedings
OBJECTIVE :
PATIENTS AND METHODS :
RESULTS :
CONCLUSION :
Potretzke Theodora A, Korfiatis Panagiotis, Blezek Daniel J, Edwards Marie E, Klug Jason R, Cook Cole J, Gregory Adriana V, Harris Peter C, Chebib Fouad T, Hogan Marie C, Torres Vicente E, Bolan Candice W, Sandrasegaran Kumaresan, Kawashima Akira, Collins Jeremy D, Takahashi Naoki, Hartman Robert P, Williamson Eric E, King Bernard F, Callstrom Matthew R, Erickson Bradley J, Kline Timothy L
2023-Mar-15
Weekly Summary
Receive a weekly summary and discussion of the top papers of the week by leading researchers in the field.