Receive a weekly summary and discussion of the top papers of the week by leading researchers in the field.
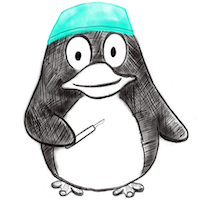
Identification of immune-related hub genes and analysis of infiltrated immune cells of idiopathic pulmonary artery hypertension.
In Frontiers in cardiovascular medicine
OBJECTIVES :
METHODS :
RESULTS :
CONCLUSION :
Chen Yubin, Ouyang Tianyu, Yin Yue, Fang Cheng, Tang Can-E, Jiang Longtan, Luo Fanyan
2023
biomarker, ceRNA network, idiopathic pulmonary artery hypertension, immune cells, immune gene, inflammation
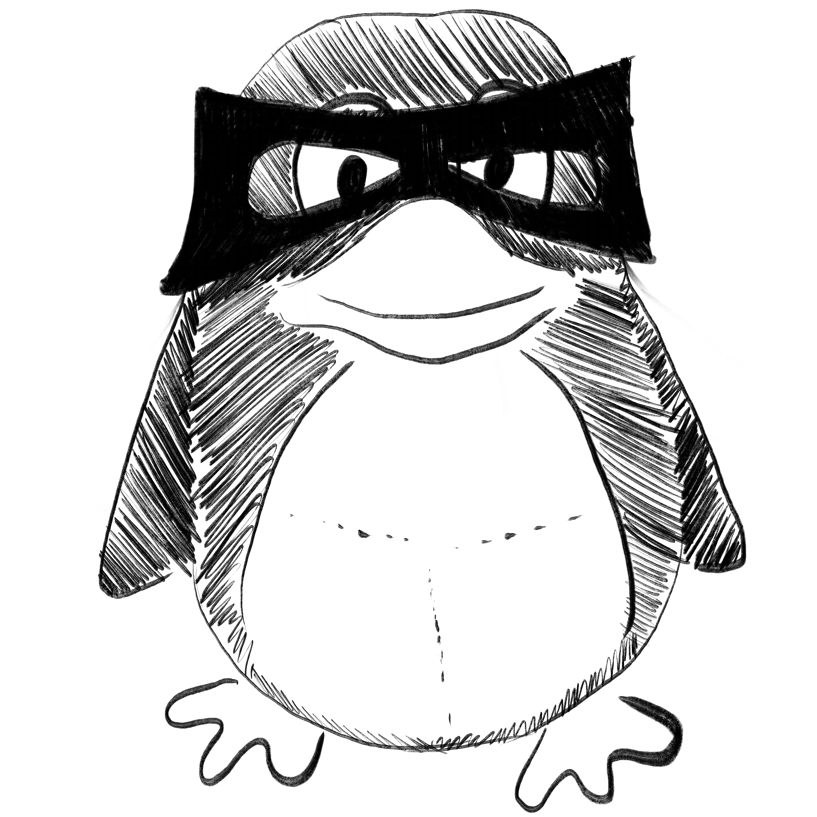
A Comprehensive Review of the Role of Artificial Intelligence in Obstetrics and Gynecology.
In Cureus
Malani Sagar N, Shrivastava Deepti, Raka Mayur S
2023-Feb
artificial intelligence in medicine, artificial neural networks, gynecology, obstetrics, postpartum period, ultrasonography
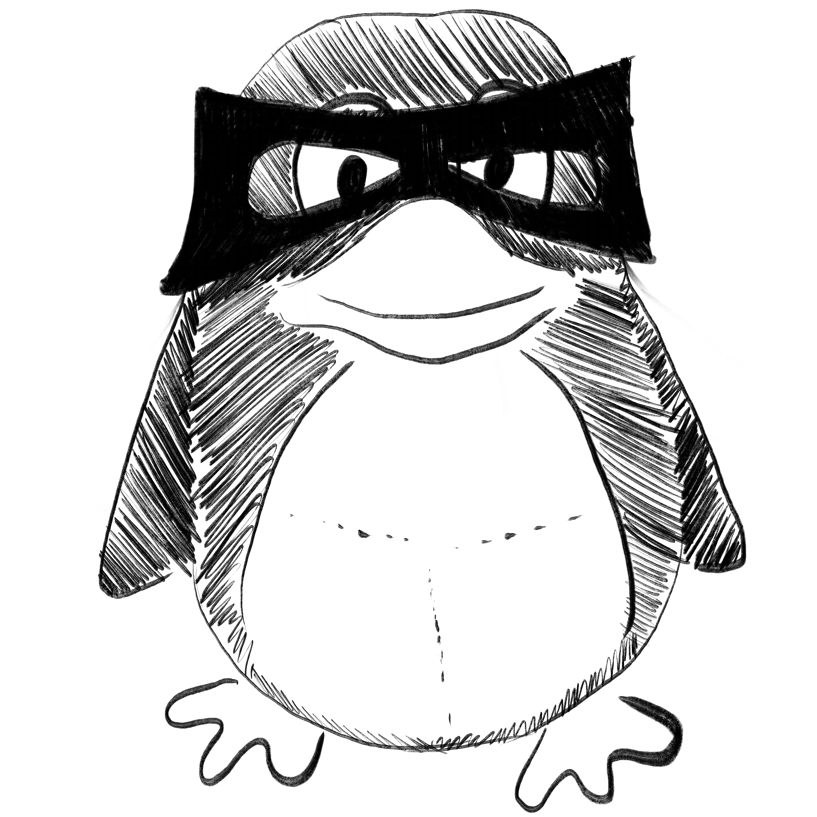
An architectural approach to modeling artificial general intelligence.
In Heliyon
Slavin Boris B
2023-Mar
Architectural approach, Artificial intelligence, Artificial intelligence modeling, General artificial intelligence, Intelligence subjectivity
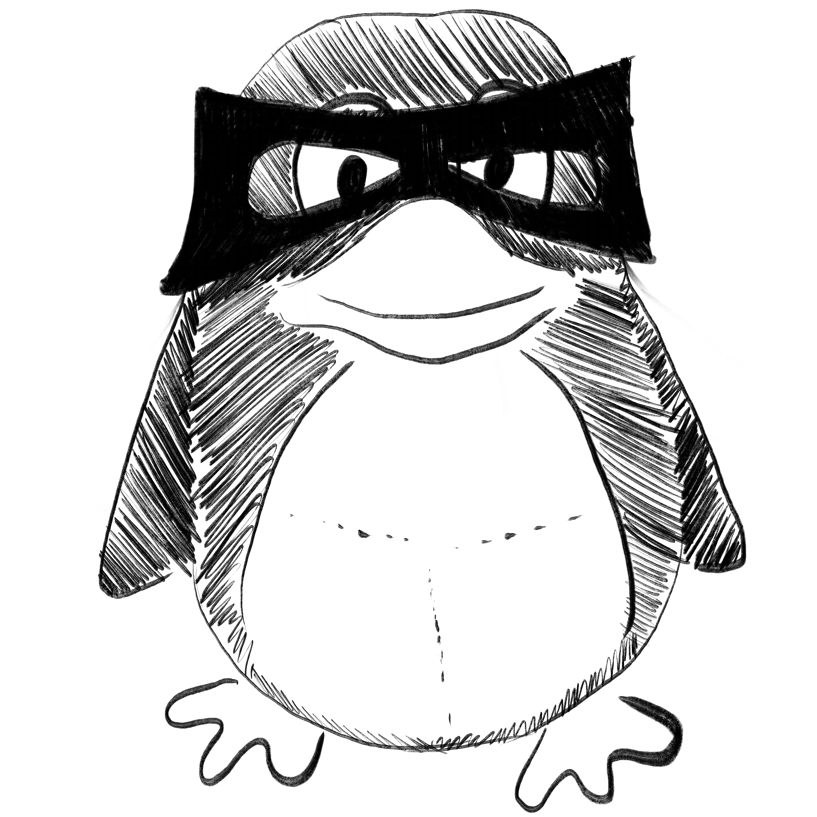
The softening of Chinese digital propaganda: Evidence from the People's Daily Weibo account during the pandemic.
In Frontiers in psychology ; h5-index 92.0
INTRODUCTION :
METHOD :
RESULTS :
DISCUSSION :
Zhang Chang, Zhang Dechun, Shao Hsuan Lei
2023
COVID-19, China, Weibo, propoganda, state-run media
Segmentation stability of human head and neck cancer medical images for radiotherapy applications under de-identification conditions: Benchmarking data sharing and artificial intelligence use-cases.
In Frontiers in oncology
BACKGROUND :
METHODS :
RESULTS :
CONCLUSION :
Sahlsten Jaakko, Wahid Kareem A, Glerean Enrico, Jaskari Joel, Naser Mohamed A, He Renjie, Kann Benjamin H, Mäkitie Antti, Fuller Clifton D, Kaski Kimmo
2023
MRI, anonymization, artificial intelligence (AI), autosegmentation, defacing, head and neck cancer, medical imaging, radiotherapy
Automatic quality assurance of radiotherapy treatment plans using Bayesian networks: A multi-institutional study.
In Frontiers in oncology
PURPOSE :
METHODS :
RESULTS :
CONCLUSION :
Kalendralis Petros, Luk Samuel M H, Canters Richard, Eyssen Denis, Vaniqui Ana, Wolfs Cecile, Murrer Lars, van Elmpt Wouter, Kalet Alan M, Dekker Andre, van Soest Johan, Fijten Rianne, Zegers Catharina M L, Bermejo Inigo
2023
AI, Bayesian network, plan review, quality assurance, radiotherapy
Weekly Summary
Receive a weekly summary and discussion of the top papers of the week by leading researchers in the field.