Receive a weekly summary and discussion of the top papers of the week by leading researchers in the field.
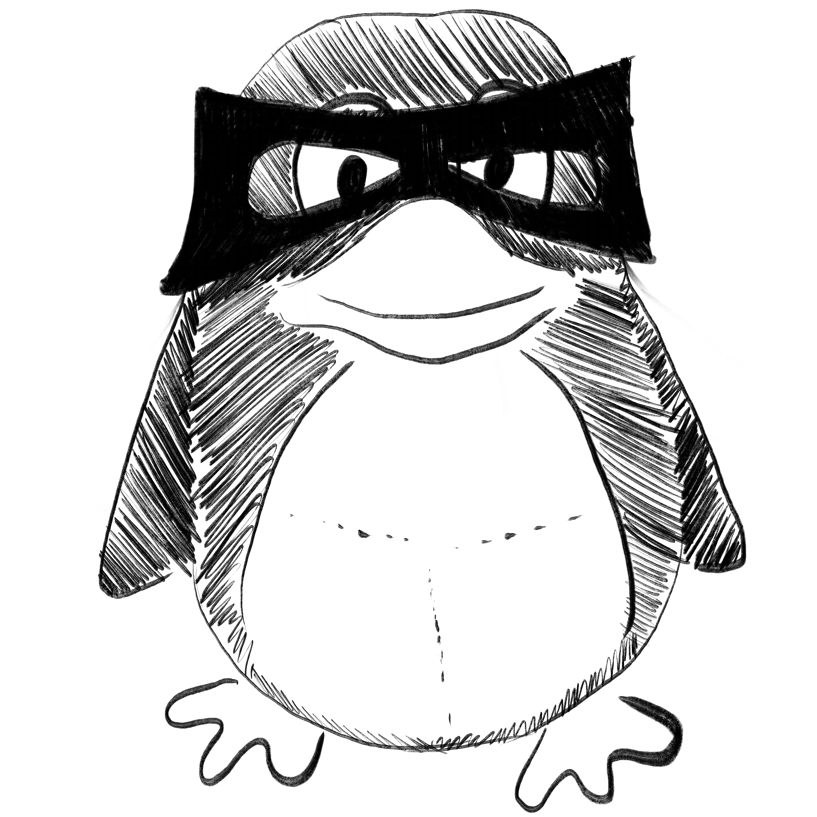
Deep Survival Analysis With Clinical Variables for COVID-19.
In IEEE journal of translational engineering in health and medicine
OBJECTIVE :
METHODS AND PROCEDURES :
RESULTS :
CONCLUSION :
CLINICAL IMPACT :
Chaddad Ahmad, Hassan Lama, Katib Yousef, Bouridane Ahmed
2023
CNN, COVID-19, clinical variables
Deep-learning-based ensemble method for fully automated detection of renal masses on magnetic resonance images.
In Journal of medical imaging (Bellingham, Wash.)
PURPOSE :
APPROACH :
RESULTS :
CONCLUSIONS :
Anush Agarwal, Rohini Gaikar, Nicola Schieda, WalaaEldin Elfaal Mohamed, Eranga Ukwatta
2023-Mar
U-Net, computer aided detection, deep learning, magnetic resonance imaging, renal masses
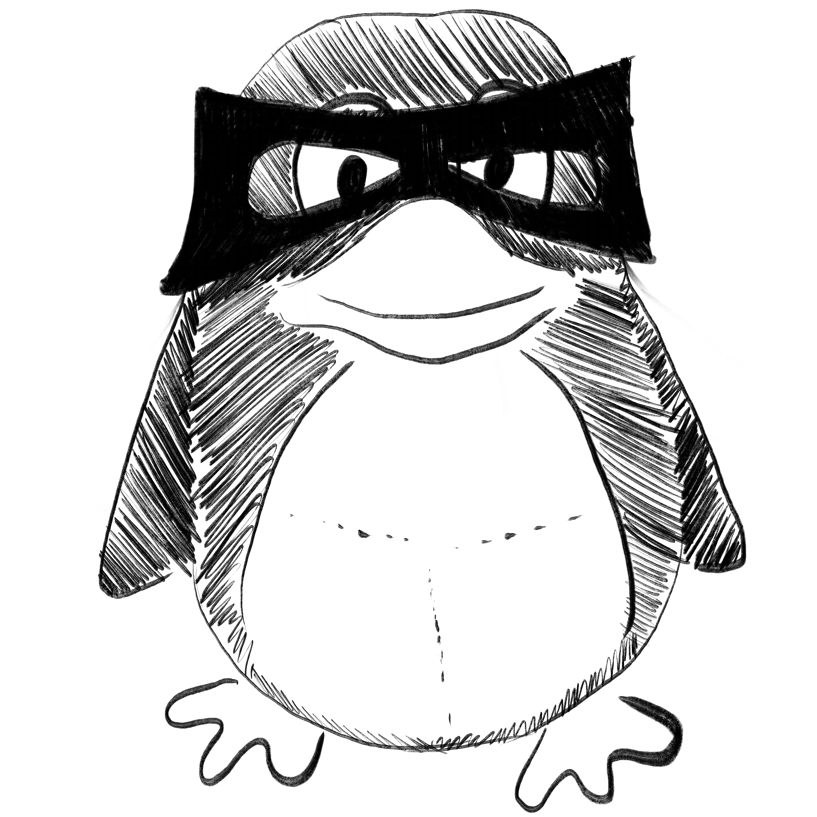
Intelligent antepartum fetal monitoring via deep learning and fusion of cardiotocographic signals and clinical data.
In Health information science and systems
PURPOSE :
METHODS :
RESULTS :
CONCLUSION :
Cao Zhen, Wang Guoqiang, Xu Ling, Li Chaowei, Hao Yuexing, Chen Qinqun, Li Xia, Liu Guiqing, Wei Hang
2023-Dec
Antepartum fetal monitoring, Cardiotocographic signal, Clinical data, Convolutional neural network, Multimodal feature fusion
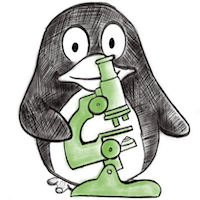
USC-ENet: a high-efficiency model for the diagnosis of liver tumors combining B-mode ultrasound and clinical data.
In Health information science and systems
PURPOSE :
METHODS :
RESULTS AND CONCLUSION :
Zhao Tingting, Zeng Zhiyong, Li Tong, Tao Wenjing, Yu Xing, Feng Tao, Bu Rui
2023-Dec
B-mode ultrasound, EfficientNet, Feature fusion, Liver tumor
COVID-19Base v3: Update of the knowledgebase for drugs and biomedical entities linked to COVID-19.
In Frontiers in public health
Basit Syed Abdullah, Qureshi Rizwan, Musleh Saleh, Guler Reto, Rahman M Sohel, Biswas Kabir H, Alam Tanvir
2023
CORD-19, COVID-19, SARS-CoV-2, deep learning, machine learning
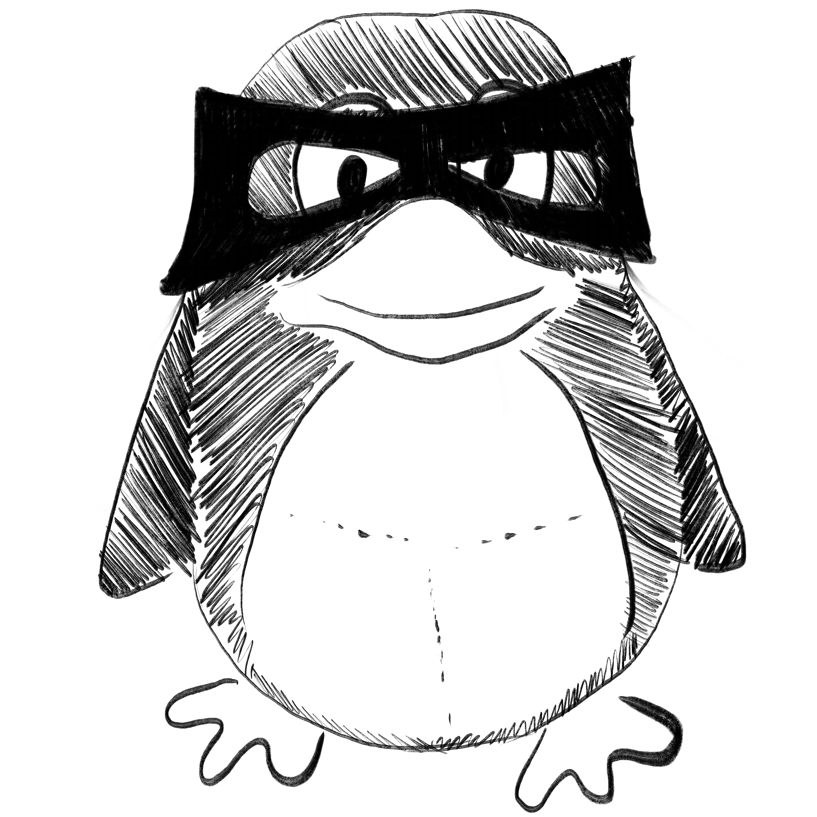
Structures, band gaps, and formation energies of highly stable phases of inorganic ABX3 halides: A = Li, Na, K, Rb, Cs, Tl; B = Be, Mg, Ca, Ge, Sr, Sn, Pb; and X = F, Cl, Br, I.
In RSC advances
Alqahtani Saad M, Alsayoud Abduljabar Q, Alharbi Fahhad H
2023-Mar-14
Weekly Summary
Receive a weekly summary and discussion of the top papers of the week by leading researchers in the field.