Receive a weekly summary and discussion of the top papers of the week by leading researchers in the field.
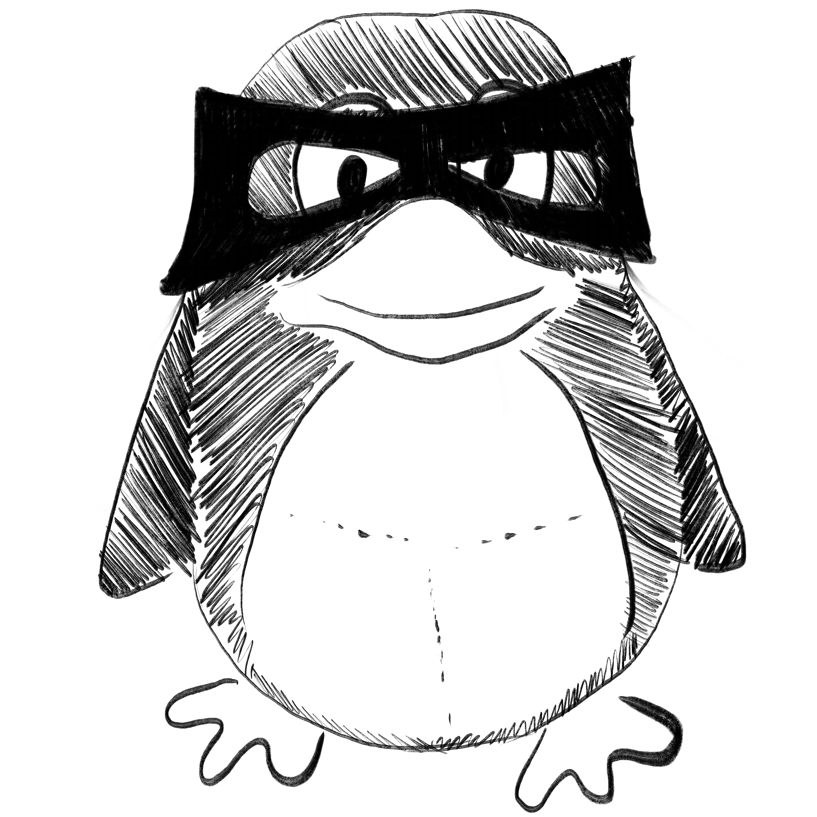
Pharmacists and pharmacy services in COVID-19 literature: A bibliometirc analysis.
In Exploratory research in clinical and social pharmacy
BACKGROUND :
OBJECTIVE :
METHODS :
RESULTS :
CONCLUSIONS :
Thabit Abrar K, Alsulmi Wajd S, Aljereb Nourah M, Khojah Omnia M, Almehdar Khadeja O, Cobo Manuel Jesús, Jose Jimmy, Vélez-Estévez Antonio
2023-Mar
Bibliometric analysis, COVID-19, COVID-19, Coronavirus disease of 2019, DOI, Digital object identifier, MeSH, Medical subject heading, Pharmacists, Pharmacy, SciMAT, Science Mapping Analysis Software Tool, WHO, World Health Organization
Deep learning-assisted diagnosis of femoral trochlear dysplasia based on magnetic resonance imaging measurements.
In World journal of clinical cases
BACKGROUND :
AIM :
METHODS :
RESULTS :
CONCLUSION :
Xu Sheng-Ming, Dong Dong, Li Wei, Bai Tian, Zhu Ming-Zhu, Gu Gui-Shan
2023-Mar-06
Artificial intelligence, Deep learning, Diagnosis, Femoral trochlear dysplasia, Magnetic resonance imaging
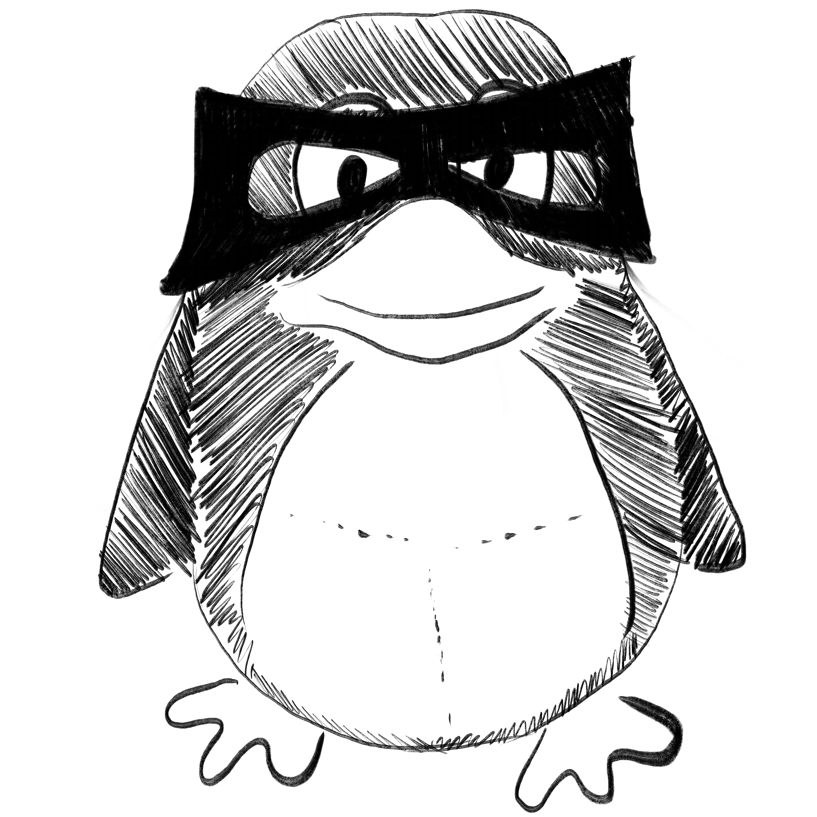
The softening of Chinese digital propaganda: Evidence from the People's Daily Weibo account during the pandemic.
In Frontiers in psychology ; h5-index 92.0
INTRODUCTION :
METHOD :
RESULTS :
DISCUSSION :
Zhang Chang, Zhang Dechun, Shao Hsuan Lei
2023
COVID-19, China, Weibo, propoganda, state-run media
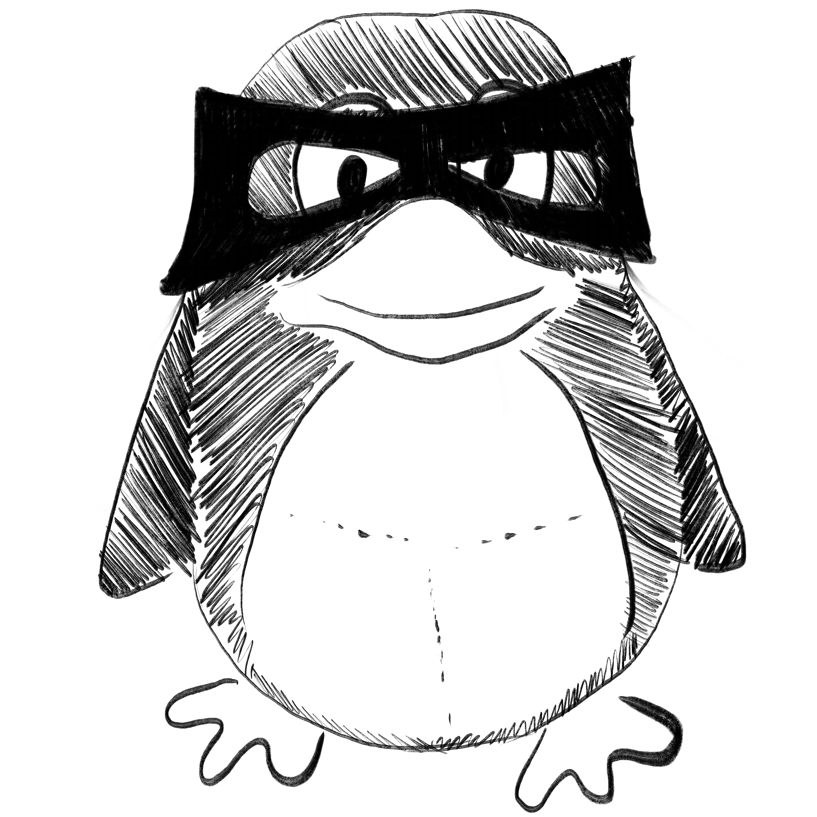
Pharmacists and pharmacy services in COVID-19 literature: A bibliometirc analysis.
In Exploratory research in clinical and social pharmacy
BACKGROUND :
OBJECTIVE :
METHODS :
RESULTS :
CONCLUSIONS :
Thabit Abrar K, Alsulmi Wajd S, Aljereb Nourah M, Khojah Omnia M, Almehdar Khadeja O, Cobo Manuel Jesús, Jose Jimmy, Vélez-Estévez Antonio
2023-Mar
Bibliometric analysis, COVID-19, COVID-19, Coronavirus disease of 2019, DOI, Digital object identifier, MeSH, Medical subject heading, Pharmacists, Pharmacy, SciMAT, Science Mapping Analysis Software Tool, WHO, World Health Organization
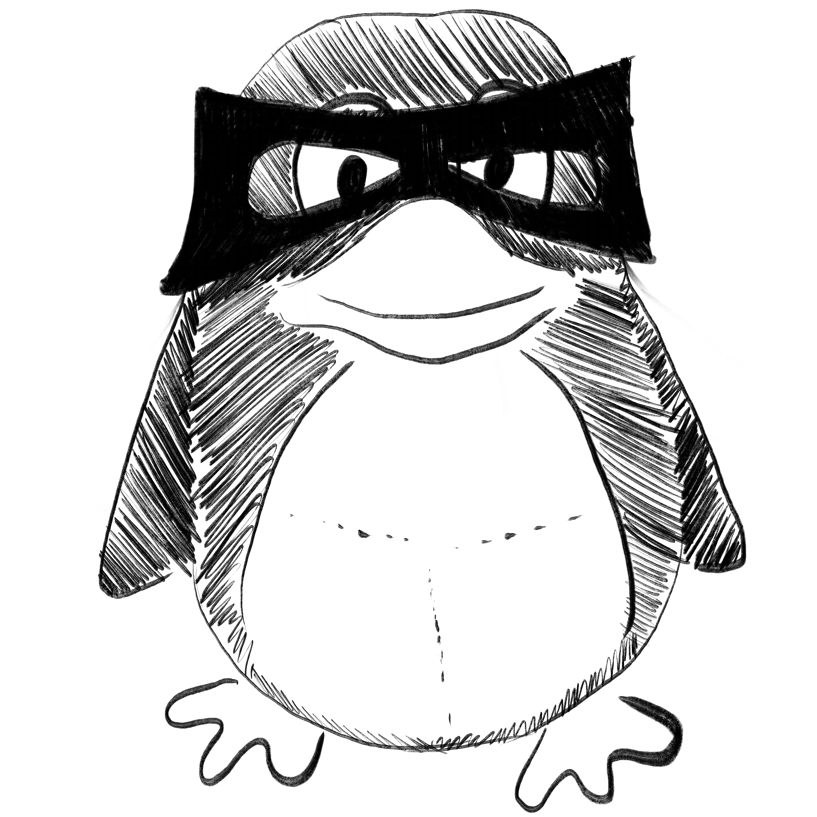
Risk factors and digital interventions for anxiety disorders in college students: Stakeholder perspectives.
In World journal of clinical cases
Liu Xin-Qiao, Guo Yu-Xin, Xu Yi
2023-Mar-06
Anxiety disorders, Artificial intelligence, Big data, College students, Digital intervention, Stakeholders
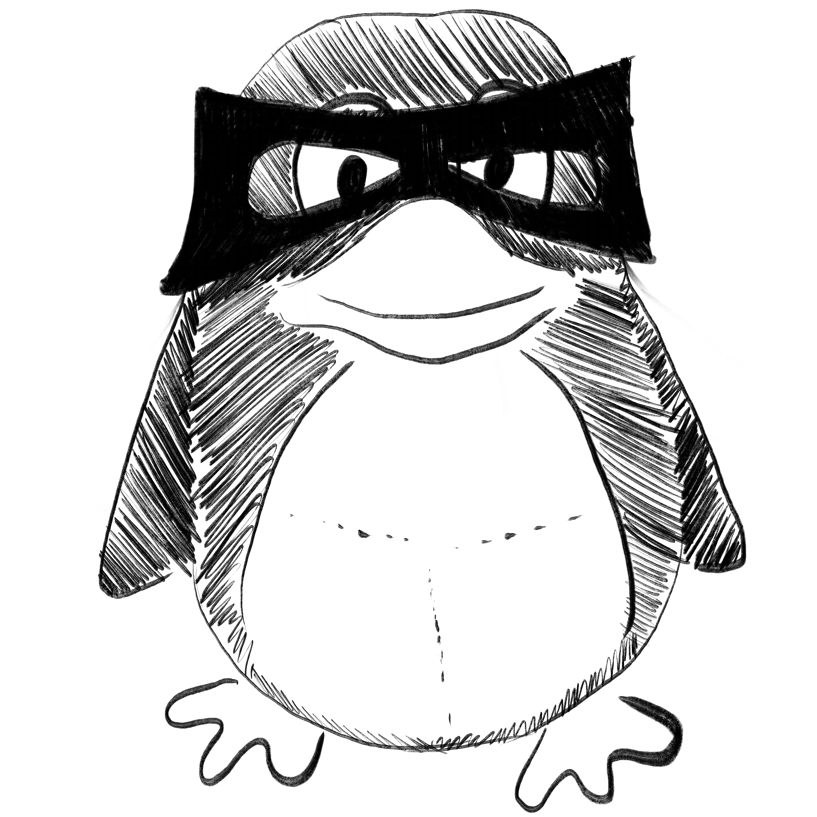
Large-scale transient peri-ictal perfusion magnetic resonance imaging abnormalities detected by quantitative image analysis.
In Brain communications
Köstner Manuel, Rebsamen Michael, Radojewski Piotr, Rummel Christian, Jin Baudouin, Meier Raphael, Ahmadli Uzeyir, Schindler Kaspar, Wiest Roland
2023
epilepsy, ictal, perfusion MRI, quantitative MRI, transient peri-ictal perfusion abnormalities TPMA
Weekly Summary
Receive a weekly summary and discussion of the top papers of the week by leading researchers in the field.