Receive a weekly summary and discussion of the top papers of the week by leading researchers in the field.
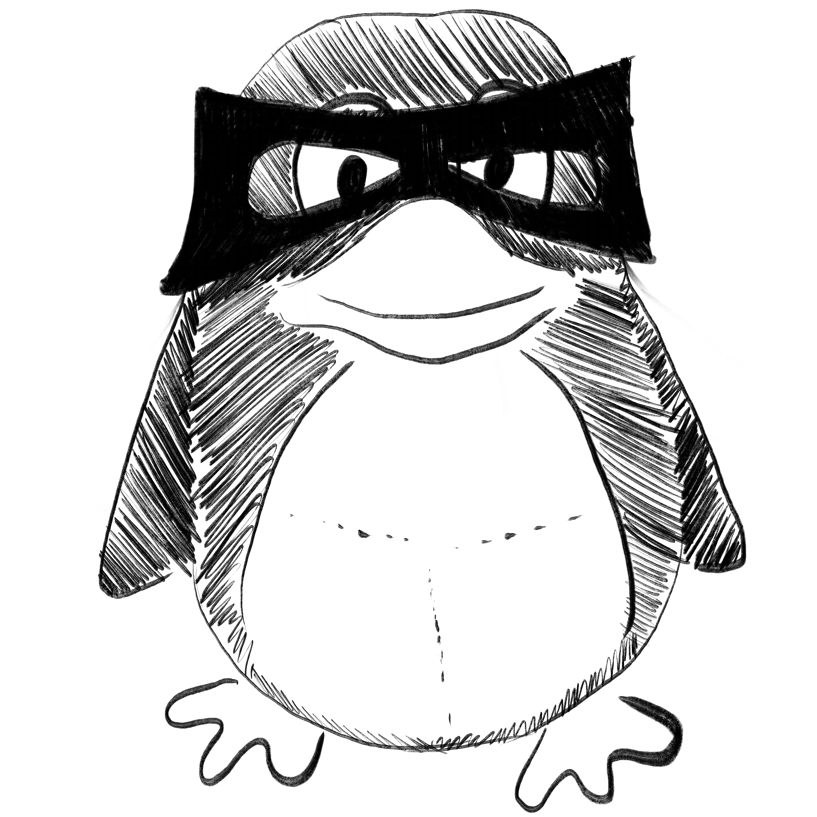
AllenDigger, a Tool for Spatial Expression Data Visualization, Spatial Heterogeneity Delineation, and Single-Cell Registration Based on the Allen Brain Atlas.
In The journal of physical chemistry. A
Wang Mengdi, Zhuo Liangchen, Ma Wenji, Wu Qian, Zhuo Yan, Wang Xiaoqun
2023-Mar-16
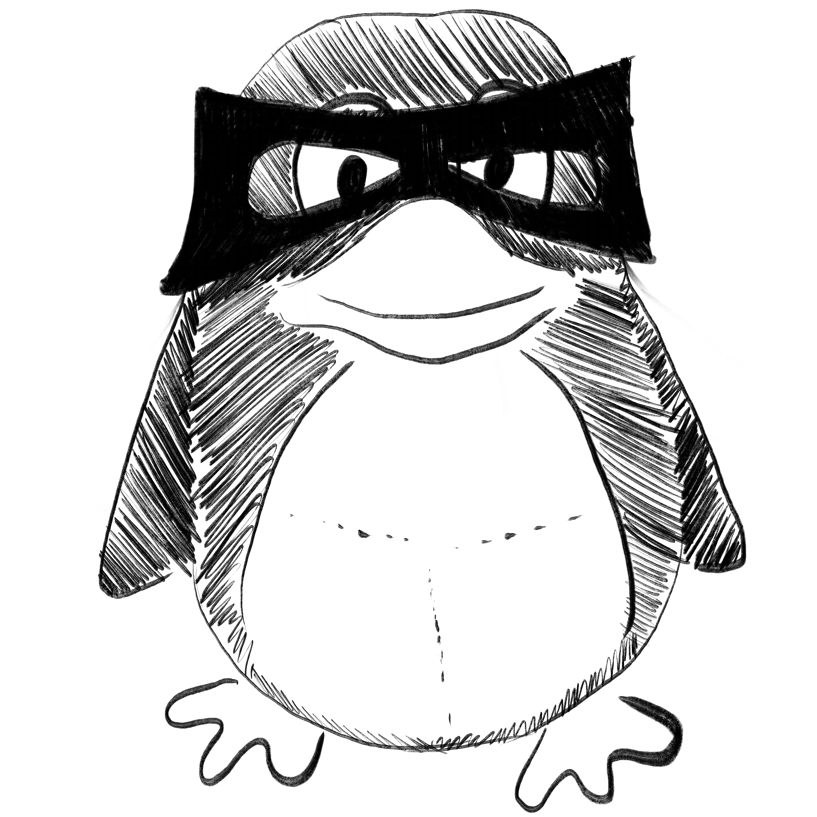
Non-invasive monitoring of microbial triterpenoid production using nonlinear microscopy techniques.
In Frontiers in bioengineering and biotechnology
Dianat Mariam, Münchberg Ute, Blank Lars M, Freier Erik, Ebert Birgitta E
2023
CARS microscopy, baker’s yeast, lipids, metabolic engineering, natural compounds, second harmonic generation
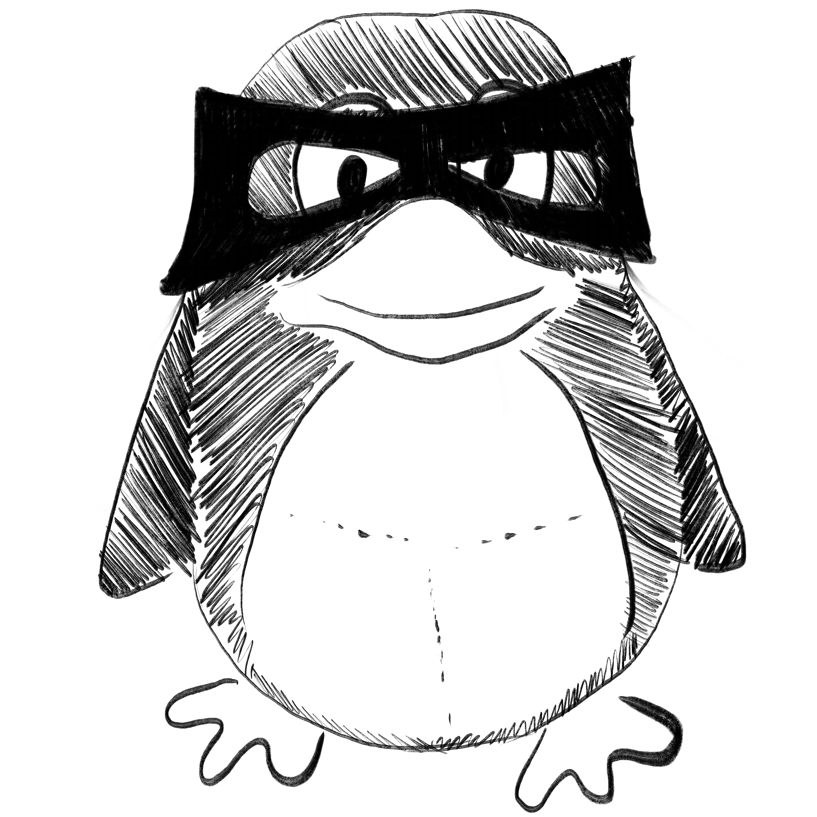
Visualization and accuracy improvement of soil classification using laser-induced breakdown spectroscopy with deep learning.
In iScience
Chu Yanwu, Luo Yu, Chen Feng, Zhao Chengwei, Gong Tiancheng, Wang Yanqing, Guo Lianbo, Hong Minghui
2023-Mar-17
Laser, Machine learning, Soil science
A multimodal analysis of genomic and RNA splicing features in myeloid malignancies.
In iScience
Durmaz Arda, Gurnari Carmelo, Hershberger Courtney E, Pagliuca Simona, Daniels Noah, Awada Hassan, Awada Hussein, Adema Vera, Mori Minako, Ponvilawan Ben, Kubota Yasuo, Kewan Tariq, Bahaj Waled S, Barnard John, Scott Jacob, Padgett Richard A, Haferlach Torsten, Maciejewski Jaroslaw P, Visconte Valeria
2023-Mar-17
Bioinformatics, Cancer, Omics
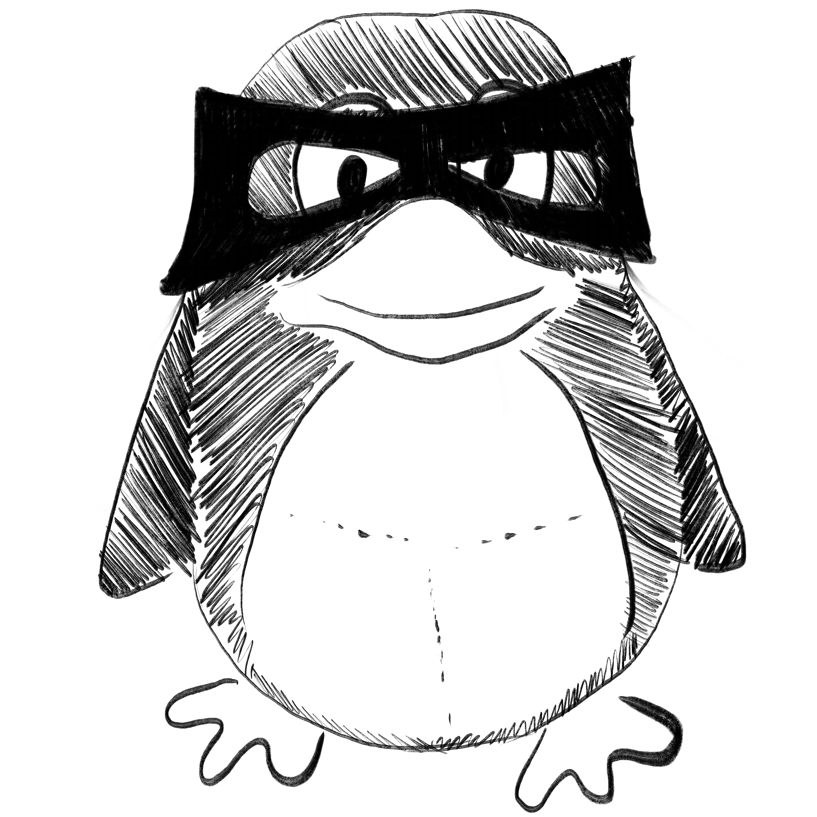
Sleep staging in the ICU with heart rate variability and breathing signals. An exploratory cross-sectional study using deep neural networks.
In Frontiers in network physiology
Ganglberger Wolfgang, Krishnamurthy Parimala Velpula, Quadri Syed A, Tesh Ryan A, Bucklin Abigail A, Adra Noor, Da Silva Cardoso Madalena, Leone Michael J, Hemmige Aashritha, Rajan Subapriya, Panneerselvam Ezhil, Paixao Luis, Higgins Jasmine, Ayub Muhammad Abubakar, Shao Yu-Ping, Coughlin Brian, Sun Haoqi, Ye Elissa M, Cash Sydney S, Thompson B Taylor, Akeju Oluwaseun, Kuller David, Thomas Robert J, Westover M Brandon
2023
artificial intelligence-AI, deep learning-artificial neural network, heart rate variability (HRV), intensive care unit (ICU), respiration, sleep, sleep staging
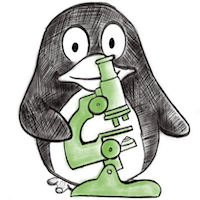
Ranking Breast Cancer Drugs and Biomarkers Identification Using Machine Learning and Pharmacogenomics.
In ACS pharmacology & translational science
Mehmood Aamir, Nawab Sadia, Jin Yifan, Hassan Hesham, Kaushik Aman Chandra, Wei Dong-Qing
2023-Mar-10
Weekly Summary
Receive a weekly summary and discussion of the top papers of the week by leading researchers in the field.