Receive a weekly summary and discussion of the top papers of the week by leading researchers in the field.
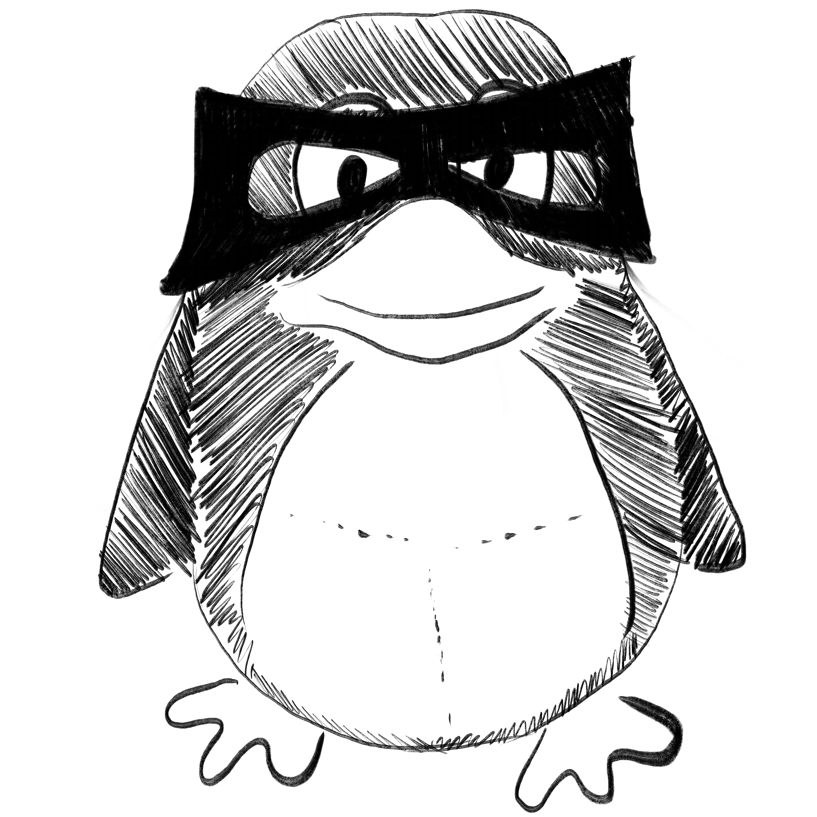
Reproductive variance can drive behavioral dynamics.
In Proceedings of the National Academy of Sciences of the United States of America
Wang Guocheng, Su Qi, Wang Long, Plotkin Joshua B
2023-Mar-21
cooperation, demographic stochasticity, evolutionary game theory, over-dispersion
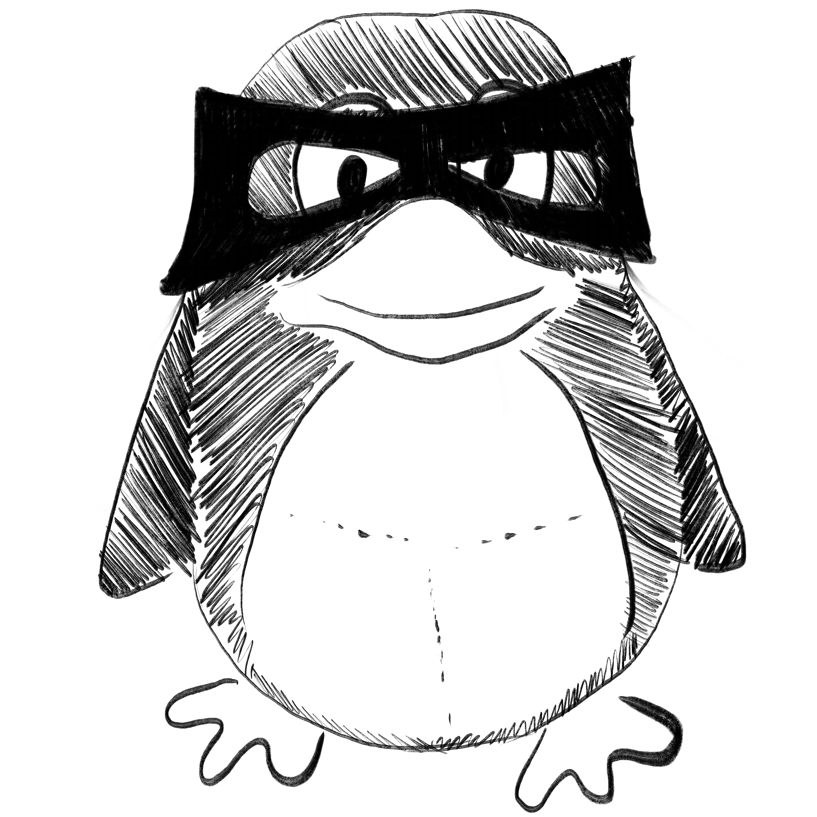
OzNet: A New Deep Learning Approach for Automated Classification of COVID-19 Computed Tomography Scans.
In Big data
Ozaltin Oznur, Yeniay Ozgur, Subasi Abdulhamit
2023-Mar-16
2D-DWT, CNN, COVID-19 CT scans, classification, intensity adjustment
Culture intelligent workflow, structure, and steps.
In Frontiers in artificial intelligence
INTRODUCTION :
METHOD :
RESULTS :
DISCUSSION :
CONCLUSION :
Henry James Andrew
2023
artificial, health, intelligence, management, population
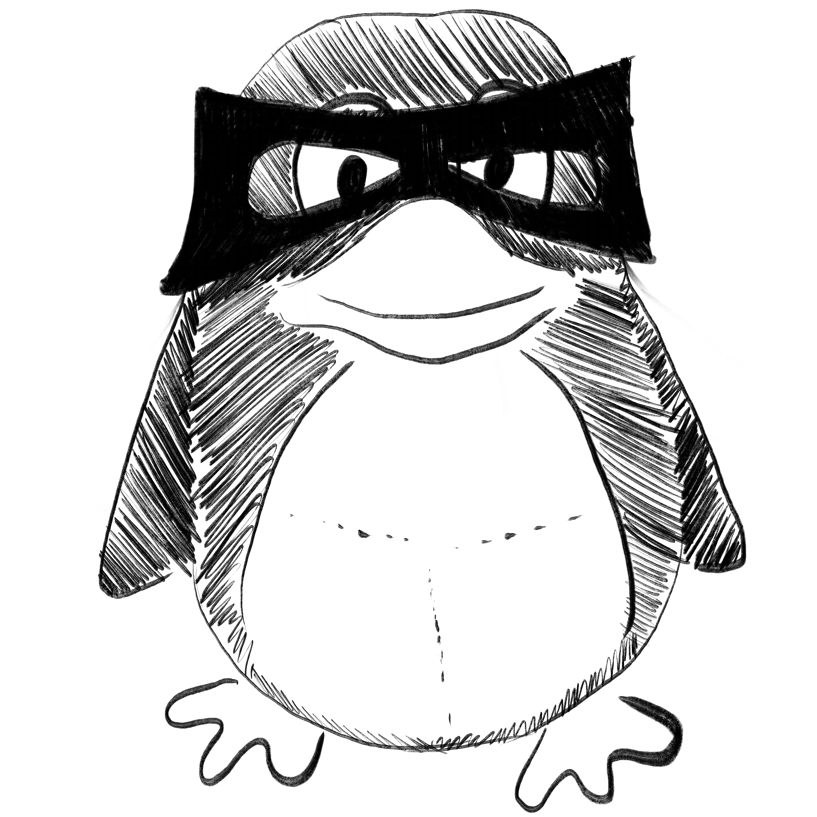
OzNet: A New Deep Learning Approach for Automated Classification of COVID-19 Computed Tomography Scans.
In Big data
Ozaltin Oznur, Yeniay Ozgur, Subasi Abdulhamit
2023-Mar-16
2D-DWT, CNN, COVID-19 CT scans, classification, intensity adjustment
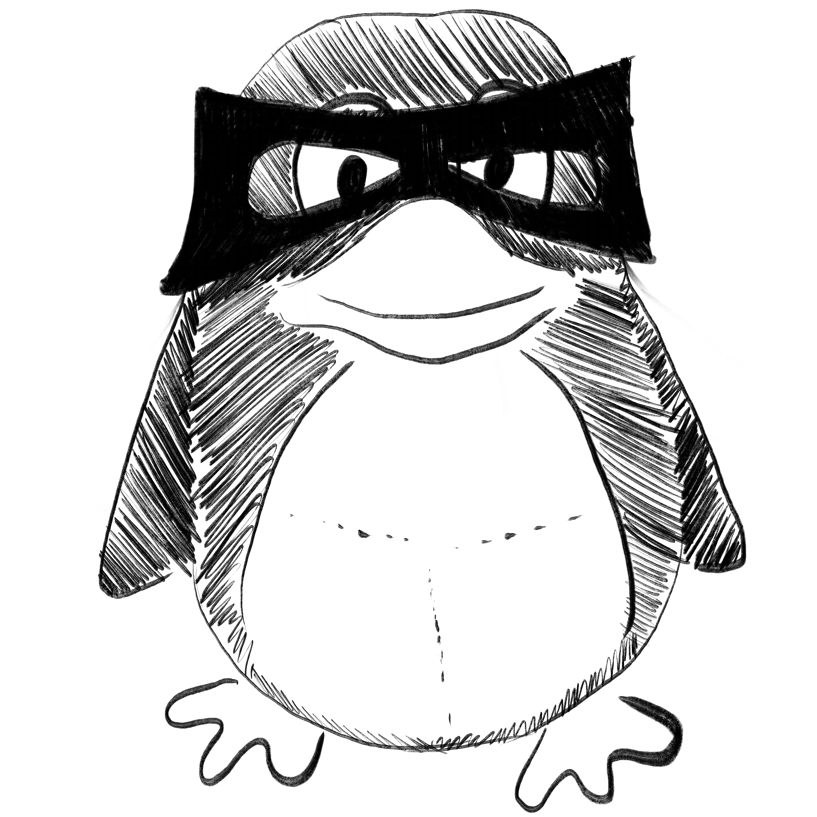
Evolutionary-scale prediction of atomic-level protein structure with a language model.
In Science (New York, N.Y.)
Lin Zeming, Akin Halil, Rao Roshan, Hie Brian, Zhu Zhongkai, Lu Wenting, Smetanin Nikita, Verkuil Robert, Kabeli Ori, Shmueli Yaniv, Dos Santos Costa Allan, Fazel-Zarandi Maryam, Sercu Tom, Candido Salvatore, Rives Alexander
2023-Mar-17
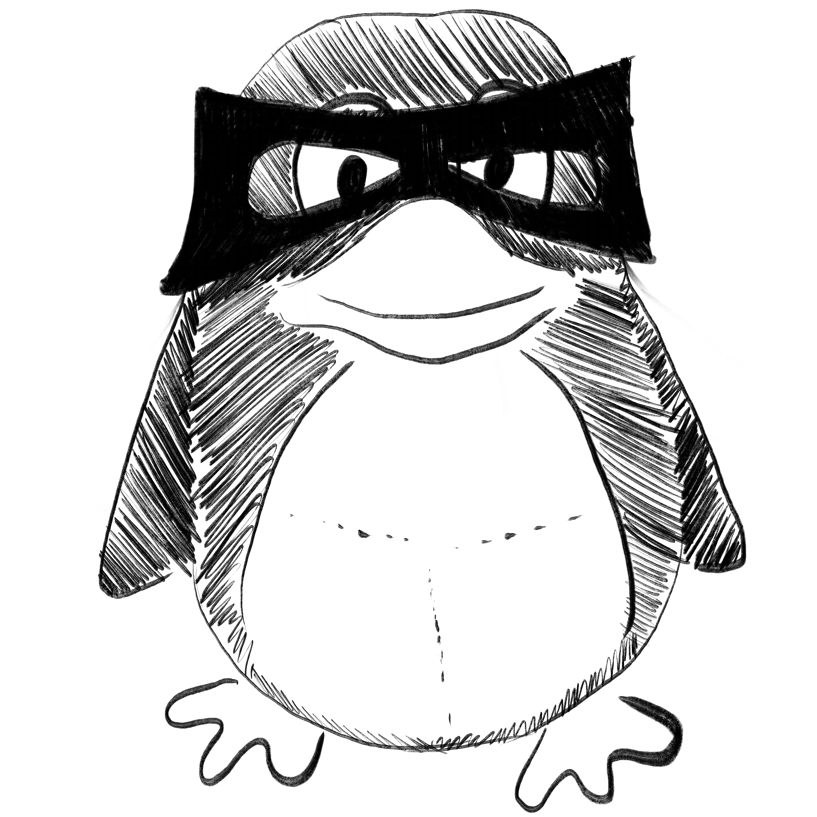
Proteochemometric Modeling Identifies Chemically Diverse Norepinephrine Transporter Inhibitors.
In Journal of chemical information and modeling
Bongers Brandon J, Sijben Huub J, Hartog Peter B R, Tarnovskiy Andrey, IJzerman Adriaan P, Heitman Laura H, van Westen Gerard J P
2023-Mar-16
Weekly Summary
Receive a weekly summary and discussion of the top papers of the week by leading researchers in the field.