Receive a weekly summary and discussion of the top papers of the week by leading researchers in the field.
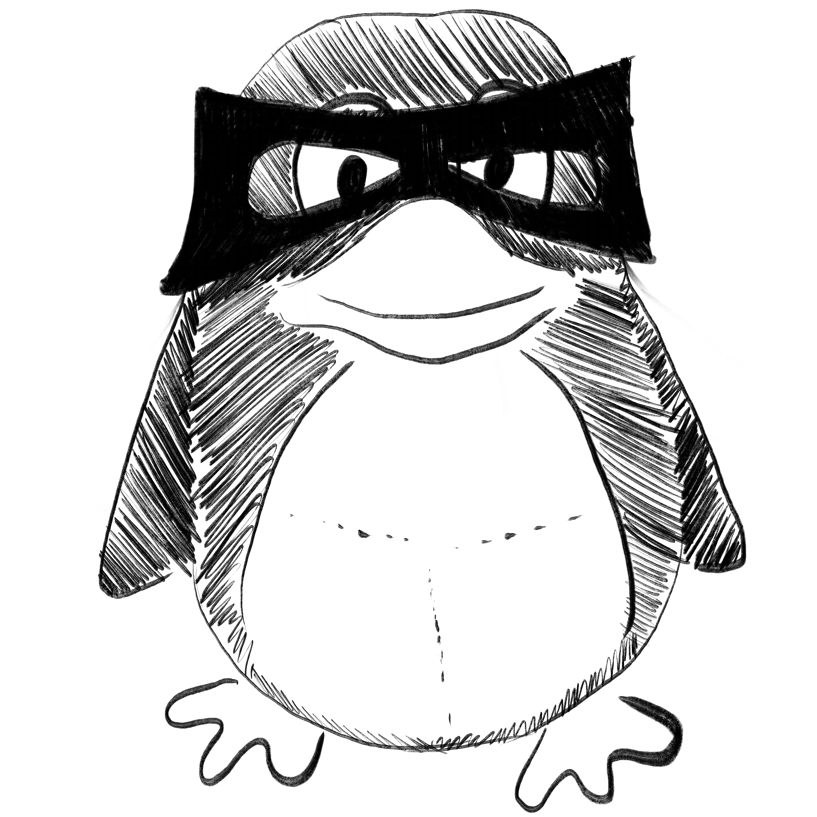
Automated prioritization of sick newborns for whole genome sequencing using clinical natural language processing and machine learning.
In Genome medicine ; h5-index 64.0
BACKGROUND :
METHODS :
RESULTS :
CONCLUSIONS :
Peterson Bennet, Hernandez Edgar Javier, Hobbs Charlotte, Malone Jenkins Sabrina, Moore Barry, Rosales Edwin, Zoucha Samuel, Sanford Erica, Bainbridge Matthew N, Frise Erwin, Oriol Albert, Brunelli Luca, Kingsmore Stephen F, Yandell Mark
2023-Mar-16
DFFNDDS: prediction of synergistic drug combinations with dual feature fusion networks.
In Journal of cheminformatics
Xu Mengdie, Zhao Xinwei, Wang Jingyu, Feng Wei, Wen Naifeng, Wang Chunyu, Wang Junjie, Liu Yun, Zhao Lingling
2023-Mar-16
Deep learning, Drug combination, Dual-feature fusion, Synergistic effect
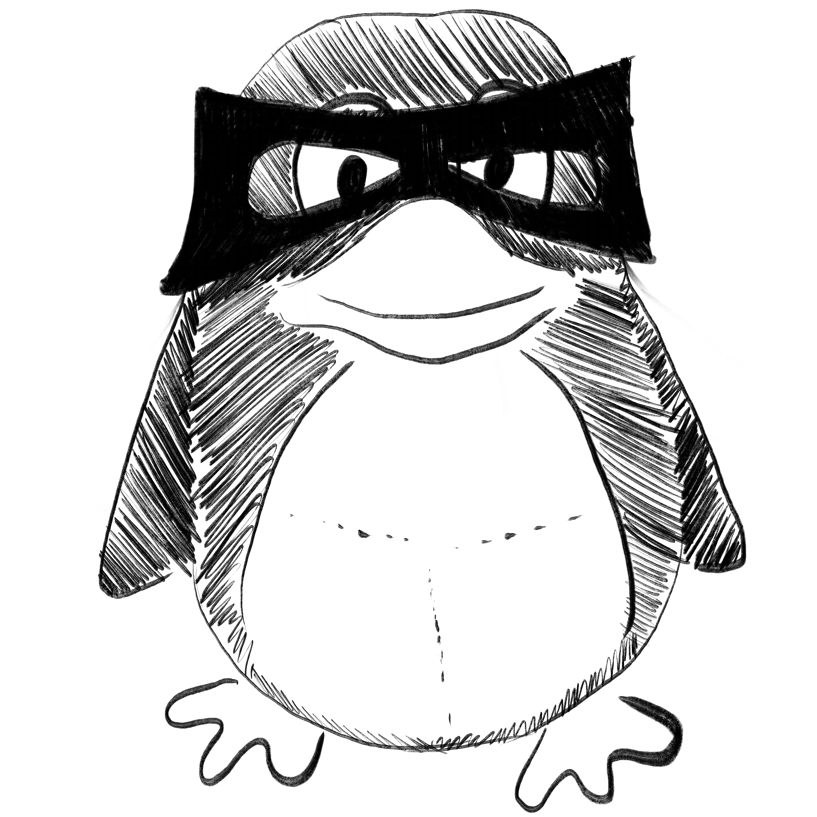
B-LBConA: a medical entity disambiguation model based on Bio-LinkBERT and context-aware mechanism.
In BMC bioinformatics
BACKGROUND :
RESULTS :
CONCLUSIONS :
Yang Siyu, Zhang Peiliang, Che Chao, Zhong Zhaoqian
2023-Mar-16
Bio-LinkBERT, Candidate ranking, Cross-attention, ELMo, Medical entity disambiguation
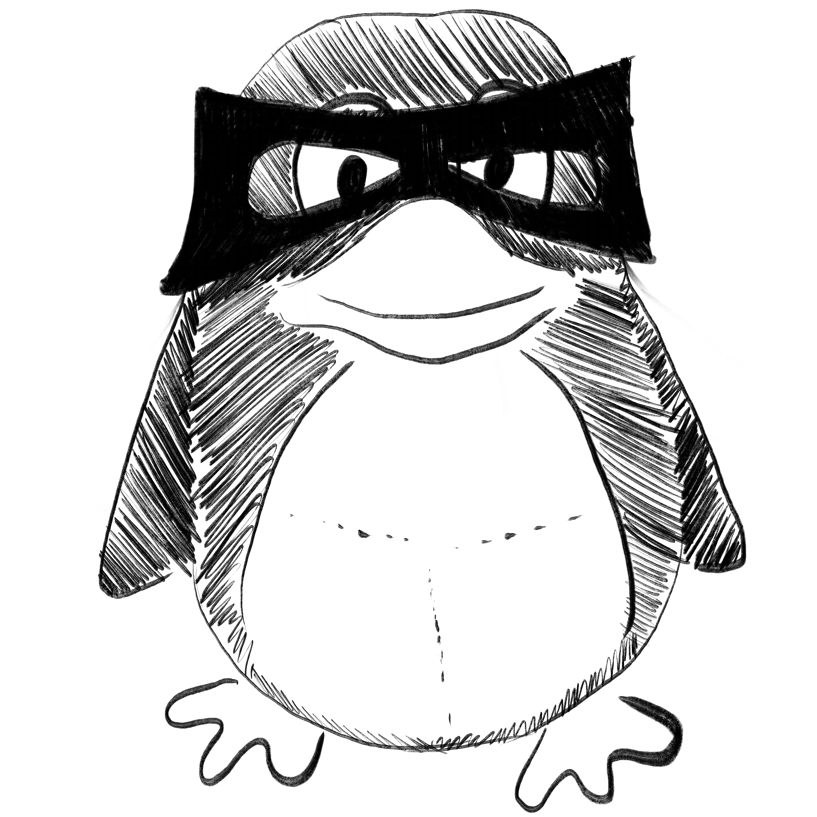
Prediction model for biochar energy potential based on biomass properties and pyrolysis conditions derived from rough set machine learning.
In Environmental technology
Tang Jia Yong, Chung Boaz Yi Heng, Ang Jia Chun, Chong Jia Wen, Tan Raymond R, Aviso Kathleen B, Chemmangattuvalappil Nishanth G, Thangalazhy-Gopakumar Suchithra
2023-Mar-17
biochar, biochar yield, carbon content, higher heating value, rough set machine learning
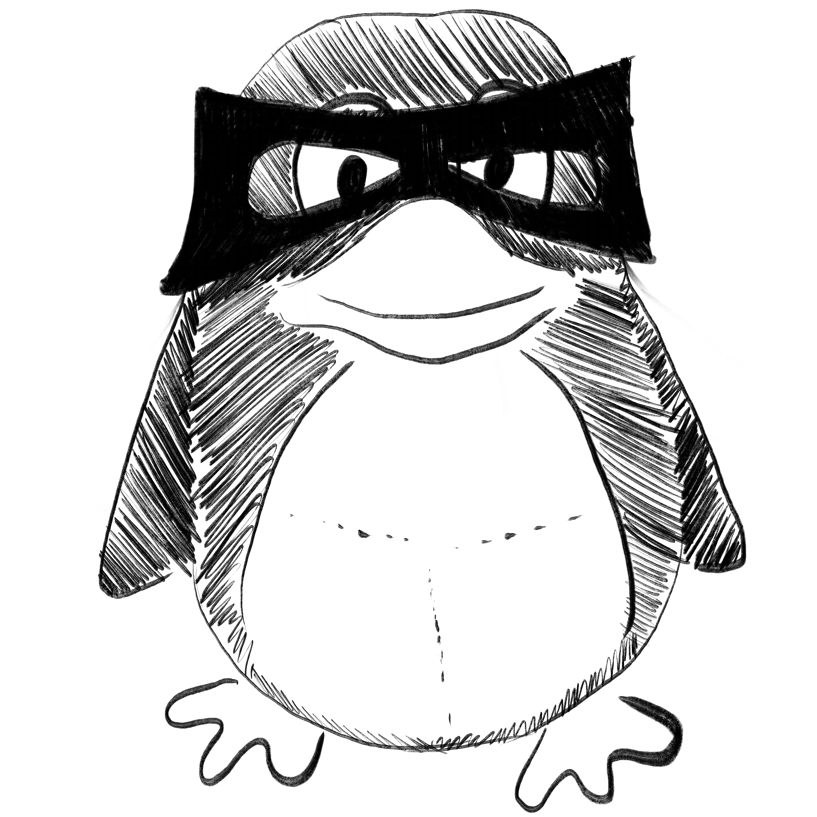
Modeling of methylene blue removal on Fe3O4 modified activated carbon with artificial neural network (ANN).
In International journal of phytoremediation
Altintig Esra, Özcelik Tijen Över, Aydemir Zeynep, Bozdag Dilay, Kilic Eren, Yılmaz Yalçıner Ayten
2023-Mar-17
Dye removal, adsorption, artificial neural networks, desorption, estimation, methylene blue
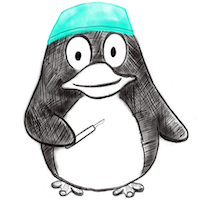
Deep Learning Prediction for Distal Aortic Remodeling After Thoracic Endovascular Aortic Repair in Stanford Type B Aortic Dissection.
In Journal of endovascular therapy : an official journal of the International Society of Endovascular Specialists
PURPOSE :
METHODS :
RESULTS :
CONCLUSION :
CLINICAL IMPACT :
Zhou Min, Luo Xiaoyuan, Wang Xia, Xie Tianchen, Wang Yonggang, Shi Zhenyu, Wang Manning, Fu Weiguo
2023-Mar-16
aortic dissection, aortic remodeling, computed tomography angiography, deep learning
Weekly Summary
Receive a weekly summary and discussion of the top papers of the week by leading researchers in the field.