Receive a weekly summary and discussion of the top papers of the week by leading researchers in the field.
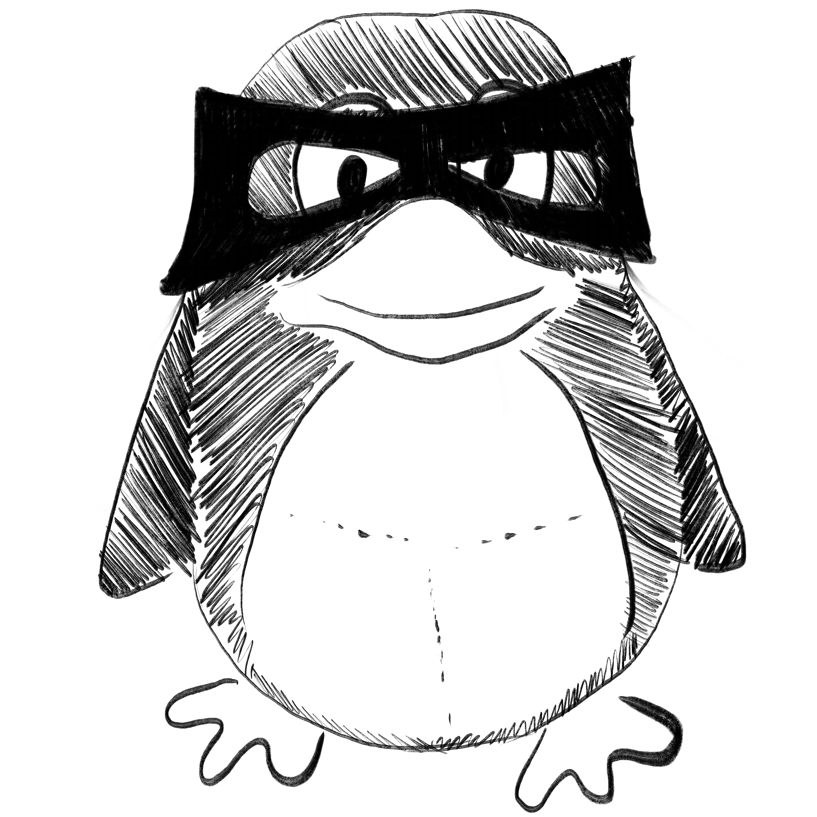
Application of MobileNetV2 to waste classification.
In PloS one ; h5-index 176.0
Yong Liying, Ma Le, Sun Dandan, Du Liping
2023
Comparison of a machine and deep learning model for automated tumor annotation on digitized whole slide prostate cancer histology.
In PloS one ; h5-index 176.0
Duenweg Savannah R, Brehler Michael, Bobholz Samuel A, Lowman Allison K, Winiarz Aleksandra, Kyereme Fitzgerald, Nencka Andrew, Iczkowski Kenneth A, LaViolette Peter S
2023
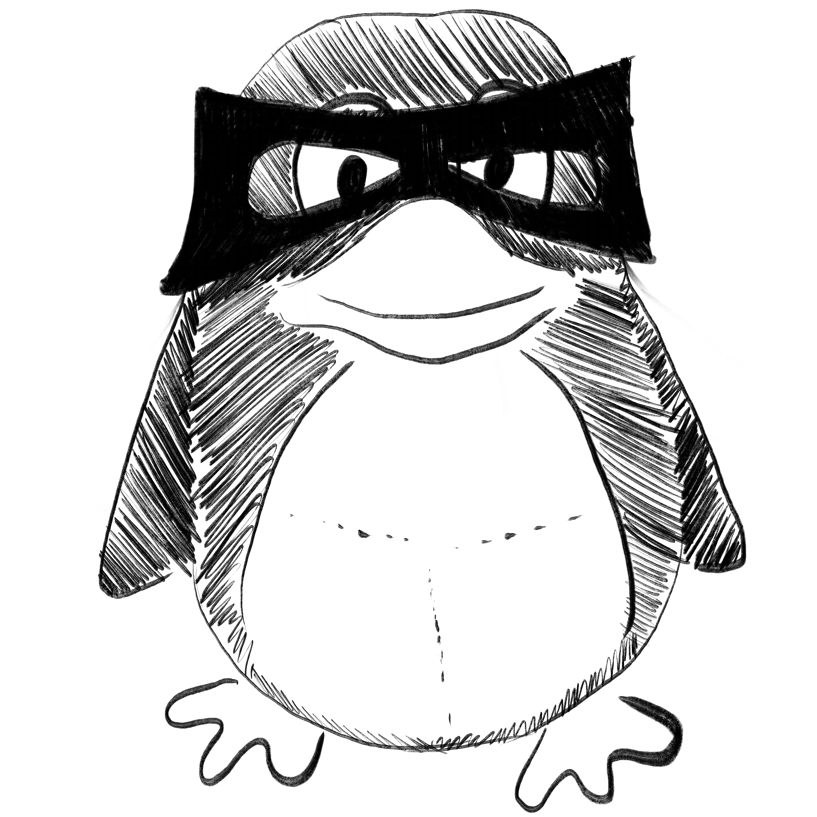
Small training dataset convolutional neural networks for application-specific super-resolution microscopy.
In Journal of biomedical optics
SIGNIFICANCE :
AIM :
APPROACH :
RESULTS :
CONCLUSIONS :
Mannam Varun, Howard Scott
2023-Mar
biomedical imaging, convolutional neural networks, dense encoder-decoder, dense layer, diffraction-limited, fluorescence microscopy, fully convolutional networks, generative adversarial networks, machine learning, small datasets, super-resolution
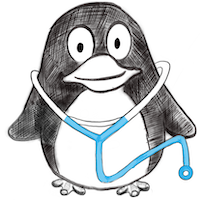
Artificial intelligence-based point-of-care lung ultrasound for screening COVID-19 pneumoniae: Comparison with CT scans.
In PloS one ; h5-index 176.0
BACKGROUND :
METHODS :
RESULTS :
INTERPRETATION :
Kuroda Yumi, Kaneko Tomohiro, Yoshikawa Hitomi, Uchiyama Saori, Nagata Yuichi, Matsushita Yasushi, Hiki Makoto, Minamino Tohru, Takahashi Kazuhisa, Daida Hiroyuki, Kagiyama Nobuyuki
2023
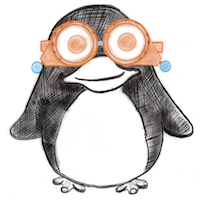
Visual electrophysiology and "the potential of the potentials".
In Eye (London, England) ; h5-index 41.0
Mahroo Omar A
2023-Mar-16
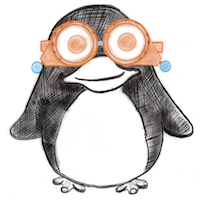
A deep learning-based framework for retinal fundus image enhancement.
In PloS one ; h5-index 176.0
PROBLEM :
AIM :
METHOD :
RESULTS :
CONCLUSION :
Lee Kang Geon, Song Su Jeong, Lee Soochahn, Yu Hyeong Gon, Kim Dong Ik, Lee Kyoung Mu
2023
Weekly Summary
Receive a weekly summary and discussion of the top papers of the week by leading researchers in the field.