Receive a weekly summary and discussion of the top papers of the week by leading researchers in the field.
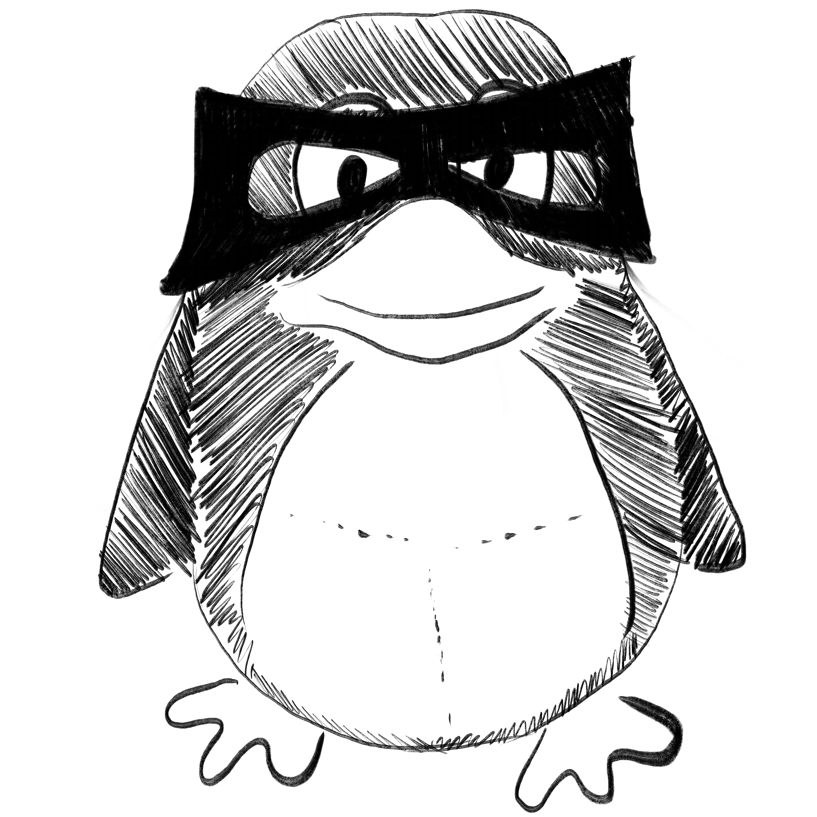
Deep Learning-based Assessment of Internal Carotid Artery Anatomy to Predict Difficult Intracranial Access in Endovascular Recanalization of Acute Ischemic Stroke.
In Clinical neuroradiology
BACKGROUND :
METHODS :
RESULTS :
CONCLUSION :
Nageler Gregor, Gergel Ingmar, Fangerau Markus, Breckwoldt Michael, Seker Fatih, Bendszus Martin, Möhlenbruch Markus, Neuberger Ulf
2023-Mar-16
Convolutional neural network (CNN), Machine learning, Mechanical thrombectomy, Tortuosity, nnUNet
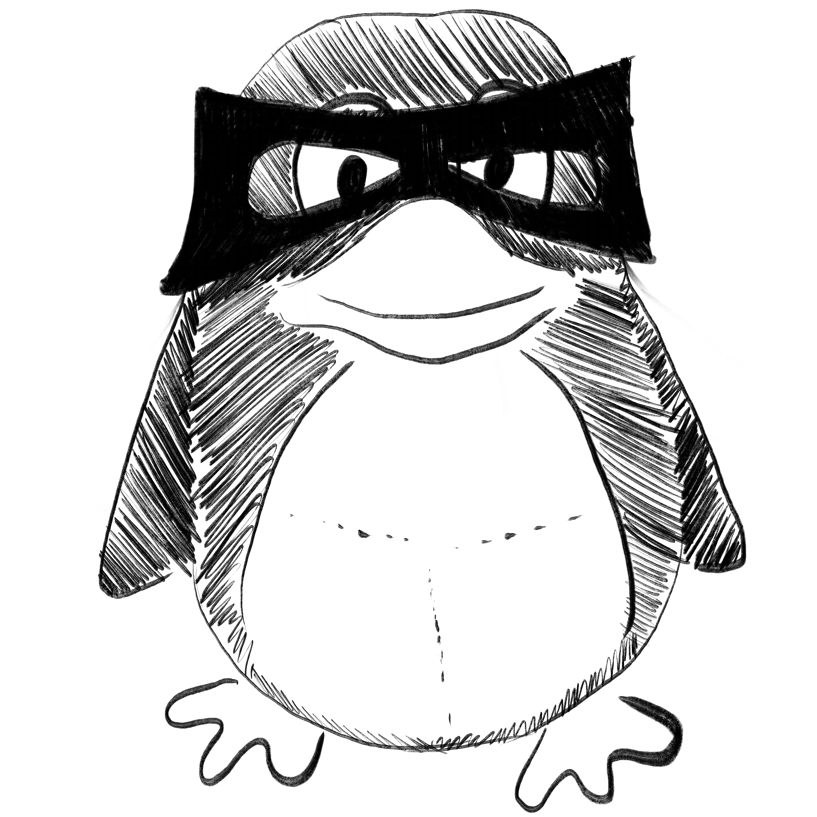
Metabolomics integrated with machine learning to discriminate the geographic origin of Rougui Wuyi rock tea.
In NPJ science of food
Peng Yifei, Zheng Chao, Guo Shuang, Gao Fuquan, Wang Xiaxia, Du Zhenghua, Gao Feng, Su Feng, Zhang Wenjing, Yu Xueling, Liu Guoying, Liu Baoshun, Wu Chengjian, Sun Yun, Yang Zhenbiao, Hao Zhilong, Yu Xiaomin
2023-Mar-16
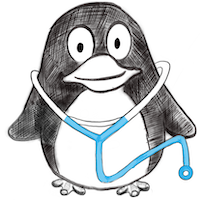
Adapted clustering method for generic analysis of histological fibrosis staining as an open source tool.
In Scientific reports ; h5-index 158.0
Remes Anca, Noormalal Marie, Schmiedel Nesrin, Frey Norbert, Frank Derk, Müller Oliver J, Graf Markus
2023-Mar-16
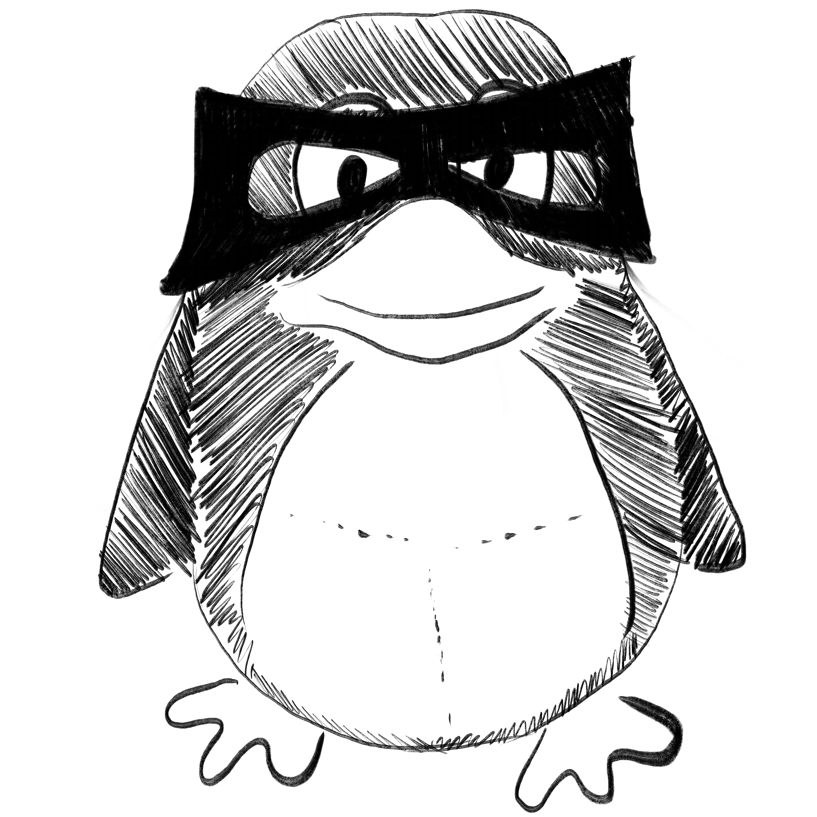
Artificial intelligence-based optimization for chitosan nanoparticles biosynthesis, characterization and in‑vitro assessment of its anti-biofilm potentiality.
In Scientific reports ; h5-index 158.0
El-Naggar Noura El-Ahmady, Dalal Shimaa R, Zweil Amal M, Eltarahony Marwa
2023-Mar-16
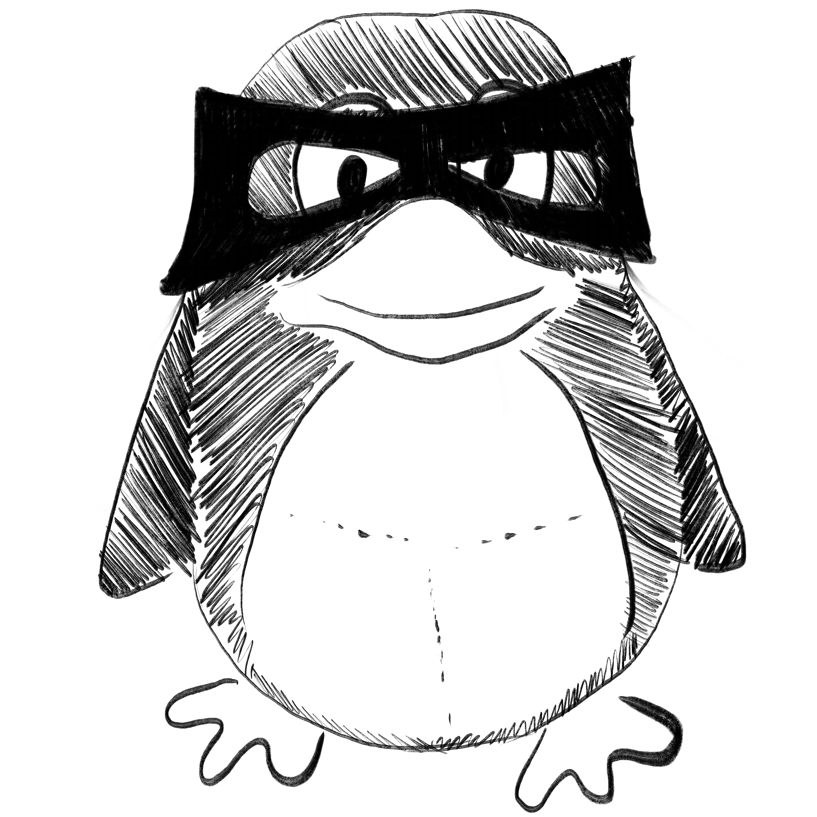
Mapping of Phragmites in estuarine wetlands using high-resolution aerial imagery.
In Environmental monitoring and assessment
Walter Matthew, Mondal Pinki
2023-Mar-17
Classification, Invasive, Machine learning, Phragmites, Vegetation
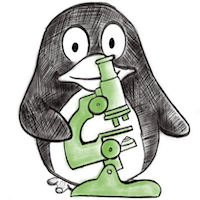
Challenges Posed by the Banff Classification: Diagnosis and Treatment of Chronic active T-cell mediated Rejection.
In Nephron
Yamamoto Izumi, Kawabe Mayuko, Hayashi Ayaka, Kobayashi Akimitsu, Yamamoto Hiroyasu, Yokoo Takashi
2023-Mar-16
Weekly Summary
Receive a weekly summary and discussion of the top papers of the week by leading researchers in the field.